Artificial Intelligence Transforms Emergency Medicine
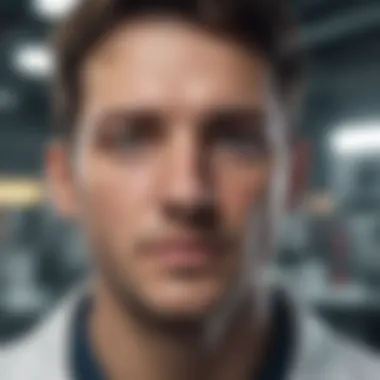
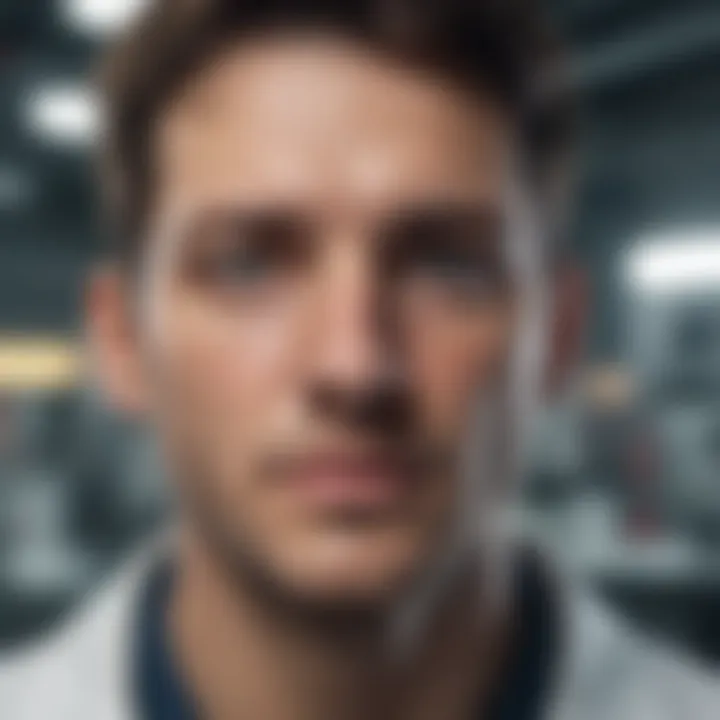
Intro
Artificial Intelligence (AI) is increasingly becoming crucial in various fields, including emergency medicine. The pressing nature of emergency care demands rapid decision-making and precise interventions, making AI a valuable asset. This article seeks to unravel how AI contributes to enhancing diagnostic accuracy, streamlining patient management, and improving operational efficiency in emergency settings.
As AI technologies develop, their integration into healthcare is transforming traditional practices. Technological advancements facilitate predictive analytics, enabling earlier detection of life-threatening conditions. Additionally, AI supports healthcare professionals by providing real-time data that helps in swift decision-making. Understanding these concepts can illuminate the potential impact of AI on patient outcomes.
Research Overview
Summary of key findings
Recent studies indicate that implementing AI in emergency medicine can significantly reduce response times and improve patient outcomes. Machine learning algorithms can analyze vast amounts of data quickly, showcasing patterns that may be overlooked by human practitioners. Furthermore, AI applications in imaging, such as deep learning analysis in radiology, exhibit promising results in identifying critical conditions like strokes or fractures.
Importance of the research in its respective field
The importance of this research cannot be overstated. Understanding AI’s capabilities within emergency medicine provides insights into how specialists can adopt these technologies effectively. By aligning AI innovations with clinical practice, healthcare systems can enhance service delivery, ensuring timely and accurate medical responses that ultimately save lives.
Methodology
Description of the experimental or analytical methods used
The methodologies in assessing AI's impact in emergency settings often involve mixed methods approaches. This includes quantitative analyses of data obtained from AI intervention outcomes, as well as qualitative studies capturing firsthand accounts from healthcare professionals. Combined, these methods yield a well-rounded view of AI's effectiveness in emergency care.
Sampling criteria and data collection techniques
Typically, studies incorporate samples from various emergency departments across different hospitals. Data is collected through electronic health records, AI intervention trial reports, and physician feedback on AI tool performance. The diverse sampling improves the reliability and validity of the findings.
Intro to Artificial Intelligence in Emergency Medicine
Artificial intelligence is becoming an integral facet of emergency medicine, transforming the way healthcare providers respond to urgent situations and manage patient care. The implementation of AI technologies can lead to increased efficiency, improved diagnosis, and better patient outcomes. This integration is significant because emergency medicine requires rapid decision-making, often based on limited information. By leveraging AI, clinicians can enhance their ability to assess scenarios and provide timely interventions.
AI in emergency settings yields various benefits. For instance, it enables more precise triage processes, allowing healthcare professionals to prioritize patients based on the severity of their conditions. Furthermore, AI-driven analytics can aid in predicting patient outcomes, resulting in tailored treatments suited to individual needs.
As the healthcare landscape continues to evolve, understanding the role of AI is vital. There are several considerations to ensure that technology aligns with medical ethics and enhances care rather than complicating it. The balance is crucial; without proper integration, AI may create more challenges than solutions.
The following sections will delve into defining AI technologies, examining the unique features of emergency medicine, and exploring how AI can significantly impact this critical field of healthcare.
Defining Artificial Intelligence
Artificial intelligence encompasses a range of technologies designed to simulate human intelligence in machines. This includes machine learning, natural language processing, and computer vision. AI systems learn from data, allowing them to identify patterns, make predictions, and assist in decision-making. In emergency medicine, these technologies can enhance clinical workflows and diagnostic processes effectively.
AI's capacity to analyze vast data sets is particularly beneficial in emergency contexts. By processing patient information quickly, AI can help clinicians determine the best course of action in real time. The implications of this are profound, as faster and more accurate assessments can directly influence patient survival and recovery.
Overview of Emergency Medicine
Emergency medicine is a medical specialty focused on the immediate care of patients facing acute illnesses or injuries. This field prioritizes rapid diagnosis and treatment, often in high-pressure environments such as emergency rooms and ambulatory settings. The main goal is to stabilize patients and address life-threatening conditions efficiently.
Clinicians in this specialty often make complex decisions based on incomplete data. This is where AI can play a pivotal role by offering decision support tools. With advancements in AI, emergency medicine can integrate innovative solutions to manage workflows and patient care, which is essential in a field characterized by its unpredictability and urgency.
In summary, the intersection of AI and emergency medicine promises to change the way healthcare is delivered during critical instances. As we move further into this discussion, it is imperative to explore historical contexts and current applications that highlight the transformative nature of this technology.
Historical Context of AI in Healthcare
Understanding the historical context of artificial intelligence (AI) in healthcare is crucial for appreciating its current applications and future potential, especially in the field of emergency medicine. This section explores the evolution of AI applications and technologies, detailing their significance in transforming healthcare paradigms. The insights drawn from this history provide a lens through which we can evaluate the integration of AI in emergency settings today.
Early Applications of AI in Medicine
AI's journey in the medical field began in the 1950s with simple rule-based systems. Early pioneers initiated projects like Dendral, an AI program designed to analyze chemical compounds, and MYCIN, which focused on diagnosing bacterial infections and recommending treatments. These systems laid the groundwork for subsequent developments by demonstrating AI's ability to handle complex medical data, showcasing potential benefits like improved diagnostic accuracy and operational efficiency.
Moreover, the foundational models reflected the increasing need for support tools in clinical decision-making. The success of these early AI applications, although limited, illuminated a path forward for integrating technology into healthcare. The focus during this period was primarily on processing structured data and improving patient outcomes through data-driven decision-making. Yet, challenges such as data limitation and integration barriers limited the reach of these early innovations.
Evolution of Emergency Medical Services
The evolution of emergency medical services (EMS) has closely intertwined with advancements in technology, including AI. In the 1960s and 1970s, EMS systems began adopting radio communication for quick dispatching and operational coordination. As healthcare advanced, the integration of AI technologies became increasingly relevant.
By the late 1990s, researchers started exploring AI's potential to optimize emergency services, particularly in triage and patient assessment. For example, systems that utilized decision support could help triage patients more effectively, prioritize resource allocation, and predict potential emergencies before they escalate.
As technology continued to develop, it became evident that AI could further enhance emergency responses. For instance, real-time data processing allowed emergency responders to access crucial patient information promptly, facilitating quicker decision-making during critical situations.
AI's impact on EMS has evolved through several phases, moving from purely reactive approaches to proactive strategies that leverage data analytics and machine learning. This evolution illustrates how emergency medical services transformed, influenced by historical AI applications and technological advancements.
The historical context reveals the gradual melding of AI with healthcare, setting the foundation for profound transformations in emergency medicine today.
Current Applications of AI in Emergency Medicine
The integration of artificial intelligence into emergency medicine is not merely a theoretical discussion. It is a reality that holds the promise of drastically improving patient care and operational efficiency. Current applications of AI are particularly significant because they address real-time challenges in emergency settings. The benefits can be substantial: from enhancing triage protocols to improving diagnostic accuracy and predicting patient outcomes. These systems are designed to support clinicians in delivering more precise, timely, and effective care.
AI in Triage Systems
AI in triage systems represents a pivotal advancement. Traditionally, triaging patients depends heavily on the judgment of medical professionals, which can be influenced by fatigue or bias. AI-enhanced triage systems use algorithms to analyze a patient's symptoms and medical history, helping prioritize cases based on urgency. For instance, some centers have implemented AI tools that analyze data from various sources like electronic health records and algorithms to suggest the most urgent cases requiring immediate attention. This method can dramatically reduce the time taken to assess patients, leading to faster interventions.
"AI systems can process complex patient information in moments, something human judgment may take longer to accomplish."
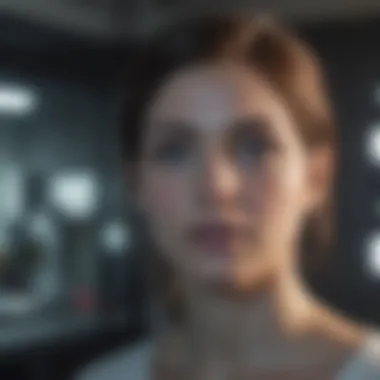
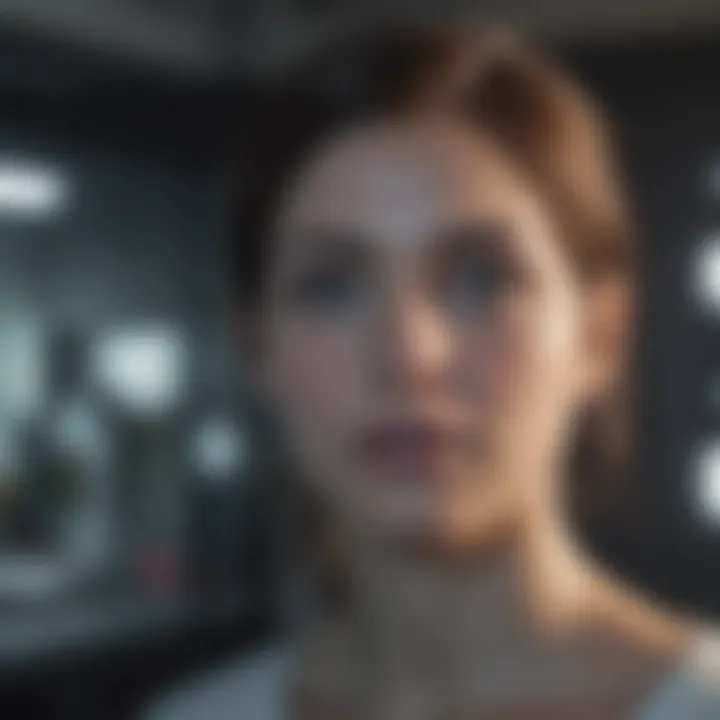
When considering the implementation of AI for triage, hospitals should examine various factors. Cost, staff training, and software integration into existing workflows are vital aspects. Also, AI must be transparent; understanding how it reaches decisions is crucial for healthcare providers to trust its recommendations.
AI-Driven Diagnostic Tools
Another significant application of AI is the development of diagnostic tools. These tools leverage machine learning to interpret diagnostic images, such as X-rays or CT scans, with great accuracy. For example, some AI systems are capable of identifying signs of pneumonia or fractures that might be missed by the human eye.
The potential for enhancing diagnostic reliability is immense. When clinicians utilize AI-driven tools, they can make more informed decisions based on objective data analysis. This boosts confidence in diagnosis and treatment plans, which is critical in emergency cases where every second counts. However, one should not overlook the importance of integrating these tools seamlessly into the clinical workflow to ensure maximum effectiveness.
Predictive Analytics for Patient Outcomes
Predictive analytics represent a further leap forward for patient management in emergency medicine. This AI application processes vast amounts of patient data to identify trends and outcomes based on historical cases. For instance, AI models can predict which patients are at risk for complications, enabling preemptive action to mitigate these risks. This foresight is beneficial in emergency departments, where swift decisions can have life-altering consequences.
Moreover, predictive analytics can assist in resource management by forecasting patient inflow and optimizing staffing levels, thereby ensuring that the most critical cases receive appropriate care without delay.
As these technologies evolve, it is essential to include ongoing evaluations and validations to maintain their accuracy and reliability in real-world scenarios. The development of these advanced AI systems can transform emergency care, ensuring that patients receive the most efficient treatment possible, ultimately leading to improved outcomes.
Enhancing Decision-Making with AI
The integration of artificial intelligence in emergency medicine significantly enhances decision-making processes. In a field where time is crucial, reducing latency in data interpretation can directly influence patient outcomes. AI technologies enable healthcare professionals to make informed choices quickly, supporting clinicians in high-stakes environments.
Real-time Data Processing and Analysis
Real-time data processing is a pivotal feature of AI applications in emergency settings. This capability allows for immediate analysis of information from various sources such as electronic health records, imaging studies, and vital sign monitors. The immediacy of access to this data aids emergency medical teams in developing a clear picture of patient conditions, leading to timely interventions.
For example, AI algorithms can rapidly assess a patient's history, current symptoms, and test results to notify healthcare providers of critical changes in a patient’s status. This is particularly important in situations like cardiac arrest, where every second counts.
The effectiveness of real-time data processing can be improved through multiple data streams. This includes input from wearable devices that monitor heart rates or blood glucose levels, giving clinicians comprehensive insights. Such integration diminishes the risk of human error and enhances the reliability of assessments, which can be a life-saving advantage.
Support for Clinical Decision-Making
AI serves as a vital support tool for clinical decision-making in emergency medicine. Algorithms and machine learning models can offer recommendations based on vast datasets derived from previous cases, helping healthcare workers overcome the limitations of human memory and experience alone.
Clinical decision support systems can analyze patterns and flag potential issues that a clinician might overlook. For instance, an AI system may suggest a diagnosis, recommend specific tests, or identify drug interactions that could endanger a patient. These suggestions are based on evidence-based guidelines, which can lead to more accurate diagnoses and thought-out treatment plans.
The adoption of AI in this aspect encourages a shared decision-making model that combines human expertise with technological support. Clinicians can focus on patient interaction while having AI as a reliable reference point. This partnership not only streamlines the workflows but also enhances the overall quality of care.
The blend of AI-driven insights with the knowledge of medical professionals marks a substantial evolution in emergency care, producing better outcomes for patients.
In summary, enhancing decision-making with AI in emergency medicine is not merely an upgrade in technology. It is a transformative shift towards greater efficiency, accuracy, and ultimately improved patient care. As these tools continue to evolve, their role in decision-making will only become more integral.
Operational Efficiency in Emergency Care
Operational efficiency in emergency care is a critical aspect that directly affects patient outcomes and healthcare costs. With the complexity and urgency inherent in emergency medicine, optimizing operations can lead to better resource utilization, shorter wait times, and improved patient experiences. In an environment where every second counts, the integration of artificial intelligence can significantly streamline processes.
Issues such as overcrowding, limited resources, and high patient turnover can challenge traditional workflows in emergency departments. This is where AI can play a transformative role. By analyzing large volumes of data quickly, AI-driven tools can help prioritize patients based on the severity of their conditions, which is essential for timely interventions.
Resource Optimization
Resource optimization focuses on ensuring that medical staff and equipment are used effectively. AI can enhance this by predicting patient inflow, allowing emergency departments to allocate staff and equipment proactively rather than reactively. For instance, machine learning algorithms can analyze historical data to forecast busy periods and adjust staffing levels accordingly. This proactive approach can ease the burden on healthcare providers and ensure patients receive timely care.
Moreover, AI systems can optimize inventory management for medical supplies. By tracking usage patterns in real time, hospitals can maintain adequate stock levels without excess waste. If a hospital frequently runs out of specific supplies during peak hours, AI can suggest adjustments to inventory processes.
Workflow Automation
Workflow automation involves using AI to enhance the efficiency of tasks within emergency care. Clinical documentation, patient tracking, and communication can become streamlined through automated systems. By automating routine tasks, healthcare professionals can dedicate more time to patient care, thus enhancing overall service quality.
AI tools can facilitate the documentation process through natural language processing, which automatically transcribes physician notes and patient interactions. This minimizes clerical work, allowing providers to focus on clinical decisions. Additionally, automated alerts can ensure that critical information reaches healthcare teams promptly, enhancing coordination in emergency situations.
By leveraging these tools, emergency departments can enhance their workflow significantly. For instance, an automated triage system can quickly assess patients' conditions and prioritize care based on urgency. This results in reduced wait times and improved overall efficiency in the emergency care setting.
Impact of AI on Patient Management and Outcomes
The integration of artificial intelligence in emergency medicine holds great importance in transforming patient management and outcomes. AI technologies streamline diagnosis, enhance treatment protocols, and improve patient tracking. Healthcare professionals face many challenges in emergency situations; therefore, the adoption of AI technologies can greatly benefit decision-making and efficiency in care delivery.
Reduction of Errors in Emergency Settings
Errors in emergency settings can have dire consequences. Misdiagnosis, medication errors, and delays in treatment are common issues that impact patient safety. AI can significantly reduce these errors by assisting medical staff with accurate data analysis and real-time information processing.
- Real-Time Data Interpretation: AI systems can analyze data from various sources, such as patient records and lab results, to identify trends and anomalies that could indicate a medical condition. This capability can help identify issues earlier and facilitate timely interventions.
- Decision Support Models: By utilizing predictive analytics, AI tools assess risks and recommend potential treatment options. This ability aids clinicians in making informed decisions while reducing reliance solely on human judgment.
- Enhanced Communication: AI can streamline communication among team members in high-pressure environments, ensuring essential information is shared quickly. This capability can minimize the chance of errors that stem from miscommunication during emergencies.
"AI technologies can serve as a safety net in emergency medicine, improving diagnostic accuracy and ultimately saving lives."
Improved Response Times
Response time is critical in emergency care. Even a minute's delay can affect a patient's chance of survival. AI systems can optimize various processes to enhance response times in emergency medical services.
- Efficient Triage Systems: AI algorithms can aid in triage by quickly evaluating patient conditions based on symptoms and vital signs. These algorithms can prioritize cases requiring immediate attention, thus improving resource allocation.
- Predictive Scheduling: AI can analyze historical data to predict peak times for emergency departments. By anticipating demand, hospitals can better prepare their staff and resources, leading to reduced wait times.
- Automated Dispatch: In pre-hospital settings, AI can help dispatch resources more effectively. By integrating geographic data and real-time traffic conditions, AI tools can optimize routes for emergency vehicles, ensuring faster arrival times at the scene.
Challenges and Limitations of AI in Emergency Medicine
The integration of artificial intelligence in emergency medicine carries great promise but is not without significant hurdles. Addressing these challenges and limitations is crucial for the effective adoption of AI technologies in high-pressure environments such as emergency rooms. This section will focus on three critical areas: data privacy and security issues, the integration of AI with existing systems, and the dependence on quality data. Understanding these aspects will allow stakeholders to devise strategies for overcoming obstacles and improving patient care outcomes.
Data Privacy and Security Issues
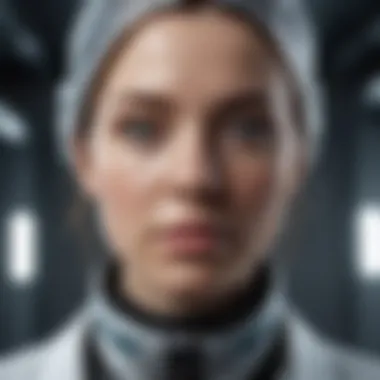
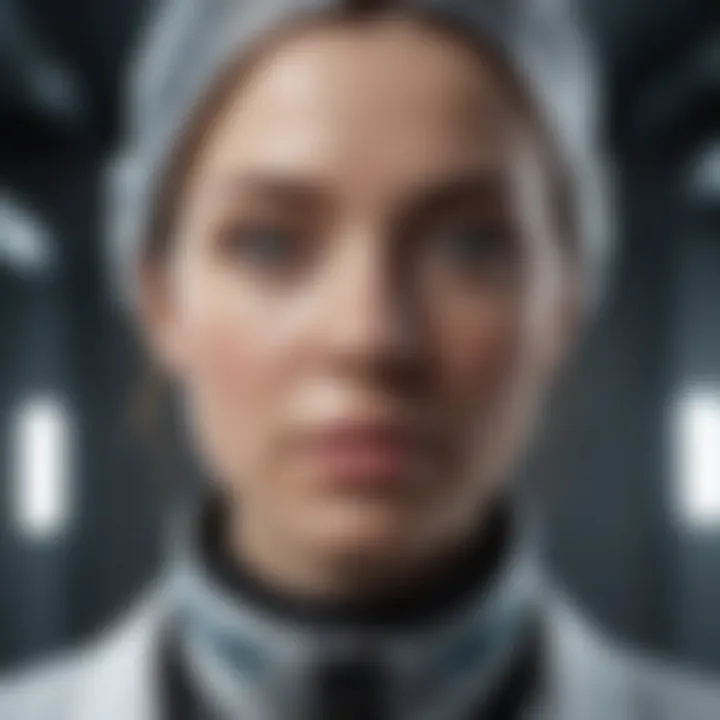
Data privacy and security are paramount in healthcare. With the increasing reliance on AI, vast amounts of sensitive patient data are collected, processed, and stored. This raises concerns about unauthorized access and potential breaches. Regulations such as the Health Insurance Portability and Accountability Act (HIPAA) aim to safeguard patient information. However, as technology evolves, so do the methods by which data can be compromised.
Medical institutions must adopt robust cybersecurity measures. These might include encryption protocols, regular software updates, and employee training programs. Compliance with legal standards must be a priority. Failure to ensure data privacy could lead to severe legal repercussions and a loss of trust among patients, complicating AI’s role in emergency medicine further.
Integration with Existing Systems
The integration of AI into existing healthcare systems presents another substantial challenge. Many emergency departments rely on legacy systems that are not designed to work seamlessly with new technologies. This lack of compatibility can hinder the effectiveness of AI applications. For example, if an AI-driven diagnostic tool cannot communicate efficiently with a hospital's electronic health records (EHR), it may result in delayed decision-making.
Healthcare facilities must evaluate their current IT infrastructure. Upgrading systems to ensure compatibility with AI solutions is essential. In addition, adequate training for healthcare professionals becomes crucial. They need to understand how to leverage AI tools effectively without disrupting the workflow. Meaningful integration will streamline operations and improve patient outcomes significantly.
Dependence on Quality Data
The effectiveness of any AI system fundamentally relies on the quality of data it processes. In emergency medicine, timely and accurate information is vital. However, variations in data collection methods and standards can compromise AI performance. Incomplete or biased datasets may lead to inaccurate predictions and recommendations, ultimately affecting patient safety.
To address this issue, healthcare providers must implement rigorous data management practices. This involves standardizing data collection protocols and ensuring that the data used for training AI algorithms is representative of the diverse patient population. Moreover, ongoing assessments of AI system performance are necessary to identify and rectify any biases or inaccuracies.
Addressing these challenges is not merely a technical issue; it is also a commitment to upholding patient care standards in an era dominated by technological advancements.
By understanding and tackling these limitations, stakeholders can establish a robust foundation for integrating AI in emergency medicine, thus enhancing both the capabilities of medical staff and the quality of patient care.
Ethical Considerations in AI Implementation
As artificial intelligence becomes more prevalent in emergency medicine, the ethical implications of its use demand significant attention. The integration of AI in healthcare carries the potential to enhance patient care. However, this must be balanced with considerations about fairness, accountability, and ethical dilemmas that may arise.
AI systems are only as good as the data they use. This raises critical concerns about bias. It is essential to ensure that AI is developed and trained with diverse datasets so that it does not favor one demographic group over others. Failure to do this can lead to unfair treatment of patients based on race, gender, or socioeconomic status. Therefore, developers should prioritize inclusivity in AI training datasets. Moreover, accountability becomes a key issue. If an AI system makes a decision that results in patient harm, it is not always clear who is responsible.
Ultimately, ethical considerations guide the framework within which AI operates in emergency medicine. They must not be an afterthought but rather integrated into the design and application processes of these systems.
Ensuring Fairness and Accountability
To navigate the ethical landscape of AI in emergency medicine, it is vital to establish frameworks that promote fairness and accountability. Transparency in AI algorithms can help build trust among healthcare providers and patients. It enables stakeholders to understand how decisions are made, which is crucial when people's health is at stake.
Implementing regular audits of AI systems is one way to ensure accountability. These audits monitor how AI interacts with patient data and ensures that diverse populations are represented in training datasets. Ethical boards can also play a role in overseeing AI deployment, ensuring compliance with both legal standards and societal expectations.
"Ensuring fairness in AI systems is not merely a technical challenge but a moral imperative for the healthcare sector."
Balancing Human Oversight and AI Autonomy
As AI takes on more roles in emergency medicine, the balance between human oversight and machine autonomy becomes essential. Human oversight is necessary to validate AI-generated recommendations and decisions. Clinicians must be involved, providing context that the AI might not fully comprehend. A machine can analyze vast datasets quickly but lacks human judgment and empathy.
At the same time, over-reliance on human oversight can hinder the efficiency that AI promises. Therefore, finding the right balance is critical. Clear guidelines should delineate when AI can act autonomously and when to revert to human intervention. This ensures that AI serves as an aide rather than a substitute for healthcare professionals.
Future Prospects of AI in Emergency Medicine
The future prospects of artificial intelligence in emergency medicine stand at the intersection of technological innovation and urgent healthcare needs. As emergency medicine continues to evolve, the potential of AI to reshape its landscape enhances both the efficiency and efficacy of care delivered under high-pressure conditions. Addressing critical challenges in time-sensitive environments is paramount, making the integration of AI not merely advantageous but essential. The need for innovative solutions to support medical professionals is driving this field forward.
Innovations on the Horizon
Innovations in artificial intelligence will likely redefine emergency medicine in the coming years. One significant area of focus includes AI-powered diagnostic systems, which utilize vast amounts of data to assist in accurate and swift diagnoses. For example, systems like Google’s DeepMind are at the forefront, employing algorithms that analyze medical imaging. This advances the speed at which conditions can be detected, allowing rapid intervention.
In addition, wearables equipped with AI capabilities are becoming more prevalent. These devices can continuously monitor patient vitals, relaying critical information to emergency teams before the patient arrives at the facility. Such innovations aim not only to improve immediate response but also to enhance the quality of information shared between pre-hospital and in-hospital care.
Moreover, advancements in natural language processing are enabling better communication tools for healthcare providers. These tools can assist in documenting patient interactions dynamically, allowing for more timely and accurate data collection while alleviating some of the administrative burdens that healthcare professionals face.
Potential for Global Health Improvement
The potential for global health improvement through AI in emergency medicine is substantial. By leveraging AI capabilities, regions with limited access to medical resources can improve diagnostic accuracy and patient outcomes. For instance, telemedicine initiatives supported by AI can facilitate remote consultations, particularly in rural or underserved areas where specialists are scarce. This can save lives and reduce unnecessary transport to urban centers.
Furthermore, machine learning algorithms can identify patterns in health crises, such as outbreaks or resource shortages. By analyzing data from various sources, these systems can predict demand surges in emergency departments, allowing for proactive planning and resource allocation. This ability to anticipate needs can significantly decrease hospital overcrowding and enhance patient care quality.
"AI in emergency medicine is poised to not just support clinical staff but transform how emergency care is architected, especially in resource-limited settings."
Case Studies of AI in Emergency Medicine
Case studies serve as practical illustrations of AI’s potential in emergency medicine. They showcase real-world applications, demonstrating how AI tools can enhance clinical outcomes. The importance of case studies cannot be overstated. They reveal the obstacles faced and solutions devised during AI implementation. Furthermore, these examples can guide future projects by providing valuable insights into best practices and pitfalls.
Successful Implementations
Successful case studies highlight how AI systems have improved efficiency and patient care. One notable example comes from the use of AI algorithms for triage in emergency departments. In a study published in a prominent medical journal, AI-powered systems were able to prioritize patients more effectively than traditional methods. This led to a reduction in waiting times and optimized resource allocation.
Another significant case is the integration of machine learning into diagnostic imaging. AI systems can analyze medical images with high accuracy, identifying conditions such as fractures or hemorrhages rapidly. Hospitals that adopted these technologies reported decreased diagnostic errors and faster therapeutic interventions. These successful implementations show the transformative power of AI in real-time scenarios.
Lessons Learned from Failures
Not all AI implementations in emergency medicine have been successful. By examining failed projects, valuable lessons can be learned. For instance, certain AI systems underestimated the complexity of emergency care. An attempt to utilize an AI tool for predictive analytics suffered setbacks due to poor data quality and lack of integration with existing workflows. This failure emphasized the necessity of high-quality data and robust system integration.
Moreover, experiments in deploying AI applications without adequate training for medical staff have led to misunderstandings and misuse of technology. These failures highlight the importance of user training and the consideration of human factors in developing AI systems. Acknowledging these lessons helps refine future approaches, ultimately improving patient care and operational efficiency.
"Learning from both success and failure is essential for the advancement of AI in healthcare."
In summary, case studies of AI in emergency medicine provide a dual perspective: they reveal both the potential of AI technologies and the obstacles to overcome. By analyzing what works and what doesn’t, stakeholders can better prepare for future innovations.
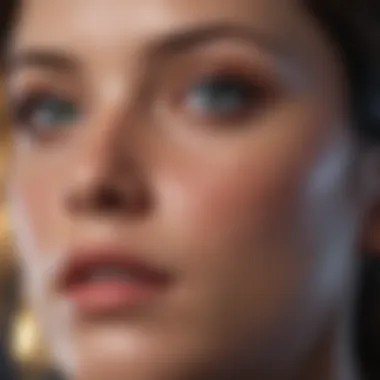
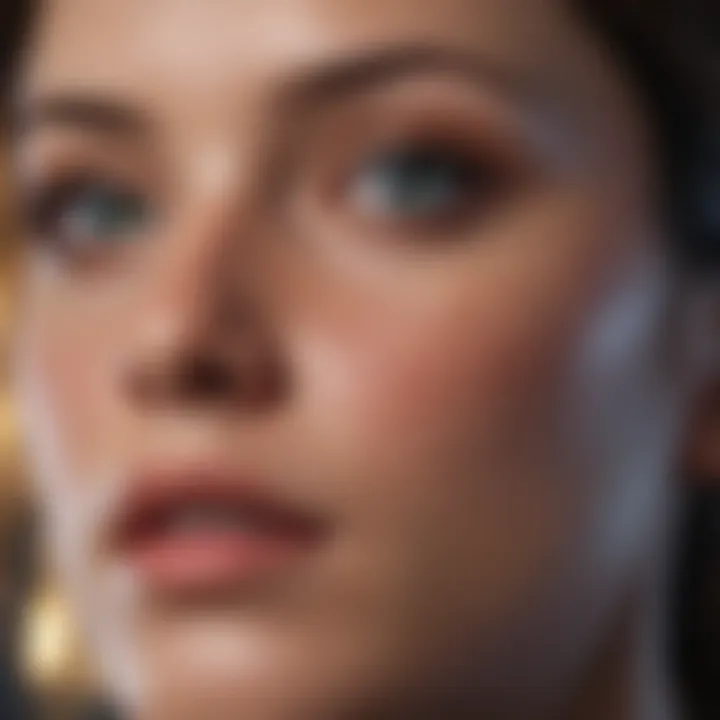
Training and Education for Medical Professionals
The integration of artificial intelligence (AI) into emergency medicine is changing the landscape of healthcare delivery. With its capacity to improve patient outcomes and operational efficiencies, training and education around AI for medical professionals cannot be overlooked. As this technology evolves, it is essential that practitioners understand not just how to use these tools, but also the underlying principles that govern their functionality. Educational initiatives, which include curriculum changes and ongoing professional development, will prepare medical staff to meet the demands that AI presents in emergency settings.
Curriculum Integration of AI Concepts
Incorporating AI concepts into the medical training curriculum ensures that future healthcare professionals are equipped to utilize these technologies effectively. Curriculum design must focus on several key elements:
- Understanding AI Fundamentals: Medical students should learn the basics of AI, including machine learning, neural networks, and data analytics. This foundational knowledge is vital in comprehending how AI can assist in diagnostics and patient management.
- Case Studies and Practical Applications: Including real-world applications of AI in emergency medicine within the coursework will enhance practical knowledge. Engaging with successful case studies enables students to see the tangible benefits of these technologies in improving patient outcomes.
- Interdisciplinary Collaboration: Courses should promote collaboration between healthcare and technology disciplines. Exposure to computational methods and their clinical applications fosters a cohesive understanding of AI's role in emergency settings.
Curriculum changes must emphasize not only the technical aspects but also the ethical implications and societal impacts of AI in healthcare. As AI becomes more prevalent, understanding its benefits and limitations will help physicians make informed decisions.
Workshops and Continuous Learning
The dynamic nature of AI technology necessitates ongoing education through workshops and training sessions. Here are some critical considerations for implementing effective continuous learning programs:
- Hands-On Training: Workshops should provide practical training on various AI tools utilized in emergency medicine. Hands-on experience will reinforce theoretical concepts and build confidence in using these systems.
- Update on Emerging Technologies: Regular workshops can ensure that healthcare professionals stay informed about the latest advancements in AI. This may include updates on innovative diagnostic tools or new AI-driven protocols that optimize patient care.
- Interprofessional Learning: Collaboration between different healthcare specialties during workshops encourages a comprehensive approach to AI in emergency care. Such settings foster discussions on best practices and shared learning experiences.
In summary, training and education in AI are essential components for effectively applying this technology in emergency medicine. Ensuring that medical professionals are well-versed in both AI principles and practical applications is the key to unlocking the full potential of AI in improving patient outcomes and the overall efficiency of emergency services.
"The integration of AI in emergency services demands a mindset that embraces innovation while prioritizing ethical standards and quality patient care."
Continued investment in education will lead to a healthcare workforce that is prepared to navigate the opportunities and challenges that AI presents.
Regulatory Frameworks and Policies for AI in Healthcare
The integration of artificial intelligence (AI) into emergency medicine necessitates a clear and structured regulatory framework. This is critical to ensure patient safety, promote innovation, and maintain public trust in the healthcare system. Regulatory frameworks serve as guidelines for the development, deployment, and oversight of AI technologies in emergency settings. They address legal, ethical, and safety considerations essential to the effective use of AI.
A comprehensive regulatory framework not only ensures that AI systems operate within defined safety margins but also safeguards against misuse and data breaches. Moreover, it promotes accountability among developers and healthcare providers. This section will examine the current regulations affecting AI deployment and future developments that may shape the regulatory landscape in healthcare.
Current Regulations Impacting AI Deployment
The current regulatory environment for AI in healthcare is evolving. Several major organizations and authorities are involved in creating and enforcing guidelines that govern AI in medicine. In the United States, the Food and Drug Administration (FDA) has become a central authority in evaluating AI technologies intended for diagnostic or therapeutic purposes. It focuses on ensuring that AI systems demonstrate effectiveness and safety before they reach the market.
Key regulations that impact AI deployment include:
- FDA Guidance Document: This document outlines the framework for AI medical devices, setting standards for clinical data requirements and post-market surveillance.
- Health Insurance Portability and Accountability Act (HIPAA): This act protects patient privacy and data security, which is crucial when handling AI-generated patient data.
- General Data Protection Regulation (GDPR): In Europe, GDPR affects how data is collected and used, giving patients greater control over their information.
These regulations highlight the emphasis on patient safety, data protection, and the need for ongoing monitoring of AI technologies.
Future Regulatory Developments
Looking ahead, the regulatory landscape for AI in emergency medicine is likely to continue to evolve. This evolution will be driven by technological advancements and the increasing complexity of AI systems. Some anticipated developments include:
- Adaptive Regulatory Frameworks: Future regulations may adopt adaptive approaches that allow quicker responses to innovations in AI technologies. This would enable compliance without stifling innovation.
- Collaborative Efforts: Greater collaboration between regulatory bodies, healthcare providers, and AI developers will be essential in formulating comprehensive policies.
- Standardization of Data: There may be future efforts to standardize data formats for AI systems, enhancing interoperability between different systems.
These potential changes will create an environment where AI can thrive while ensuring safety and accountability. The importance of establishing a solid foundation for regulation cannot be understated, as it will play a vital role in the successful integration of AI into emergency medicine.
Collaboration Between AI Developers and Healthcare Providers
Collaboration between AI developers and healthcare providers is crucial for the successful integration of artificial intelligence into emergency medicine. This partnership fosters innovation, enhances the effectiveness of AI applications, and ultimately paves the way for improved patient care. As healthcare faces increasing demands for efficiency and accuracy, aligning the goals of AI technology with the realities of medical practice becomes essential.
Creating Synergistic Relationships
Synergistic relationships between AI developers and healthcare providers can lead to significant advancements in emergency care. When both parties work together, AI tools can be better tailored to meet the specific needs of medical professionals.
- Shared Expertise: Developers understand the capabilities of AI, while healthcare providers know the practical challenges in emergency settings. This mutual understanding helps to create more effective solutions.
- Iterative Feedback Loop: Continuous input from medical personnel allows for ongoing refinement of AI systems. This loop helps developers to make adjustments based on real-world experiences, enhancing performance and relevance.
- Prototyping and Testing: By involving healthcare providers in the development phase, AI solutions can be tested in realistic environments. This can lead to better adoption rates and user satisfaction once tools are deployed in actual emergency settings.
Understanding User Needs and Feedback
Understanding user needs and active feedback from healthcare providers is a cornerstone of effective AI implementation. This ensures that the solutions developed truly address the problems faced within emergency medicine.
- User-Centric Design: AI tools must be designed with the end-user in mind. Input from healthcare workers about their workflows, preferences, and the challenges they face informs the design process.
- Feedback Mechanisms: Establishing regular channels for feedback allows for the identification of any issues post-deployment. AI systems must be adaptable and regularly updated based on this user feedback.
- Training and Support: Providing ongoing education to healthcare providers about the AI tools ensures they feel comfortable and competent in using them. Understanding how to maximize the benefits of technology is crucial in a high-stakes environment like emergency medicine.
Collaborative efforts between AI developers and healthcare providers lead to more relevant and useful AI applications that can significantly enhance emergency medical responses and outcomes.
In summary, collaboration between AI developers and healthcare providers is not just beneficial; it is necessary for the evolution of emergency medicine. Through creating synergistic relationships and understanding user needs, the integration of AI can be smooth, resulting in tools that truly meet the demands of healthcare professionals and improve patient care.
End: The Role of AI in Shaping the Future of Emergency Medicine
The integration of artificial intelligence in emergency medicine has the potential to reshape how we approach patient care. This conclusion underscores the essential role of AI in enhancing diagnostic accuracy, improving patient outcomes, and streamlining operational efficiencies. As shown in previous sections, AI's capabilities, such as real-time data processing and predictive analytics, greatly benefit emergency medical services.
AI technology assists in decision-making at critical moments, allowing healthcare providers to prioritize cases more effectively. The promise of AI lies not only in increasing the speed of treatment but also in enhancing the precision of interventions. By minimizing human errors and optimizing resource allocation, AI creates a more responsive healthcare environment.
Finally, while the benefits are significant, there must be a vigilant approach towards ethical considerations and data privacy. This responsibility ensures that the advancements in technology align with the fundamental principles of healthcare.
Summarizing Key Insights
The journey through this article highlights several critical insights regarding the transformational role of AI in emergency medicine:
- Enhanced Diagnostic Capabilities: AI-driven tools assist in assessing patient conditions more accurately and quickly than traditional methods.
- Operational Efficiency Improvements: AI technologies streamline workflows, allowing health professionals to focus on direct patient care.
- Predictive Analytics: Leveraging data to forecast patient outcomes significantly improves pre-hospital and in-hospital care.
- Ethical Considerations: Continuous evaluation of AI applications helps balance technological advancements with the ethical implications of medical practice.
These insights foster a robust understanding of how AI is steadfastly molding the field of emergency medicine.
Call to Action for Continued Research and Development
In light of the findings presented, there is an imperative need for sustained research and development in AI applications for emergency medicine. Encouraging collaborations among tech developers, healthcare providers, and regulators is vital for creating a synergistic environment that fosters innovation. Consideration of the following points is essential:
- Investment in Research: Funding initiatives focusing on AI innovations specifically designed for emergency settings can lead to breakthroughs in performance and patient care.
- Developing Ethical Guidelines: Setting regulatory standards tailored for AI solutions ensures technology serves humanity ethically and responsibly.
- Educational Programs: Training medical professionals on the use of AI tools should be an integral element of medical education to ensure effective implementation in practice.