Revolutionizing Clinical Trials with Data Science
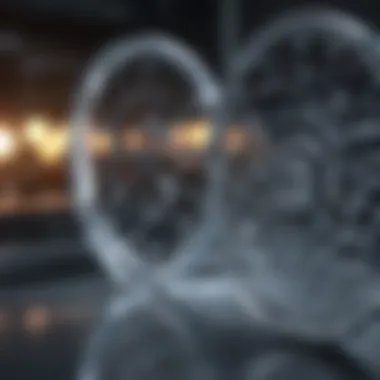
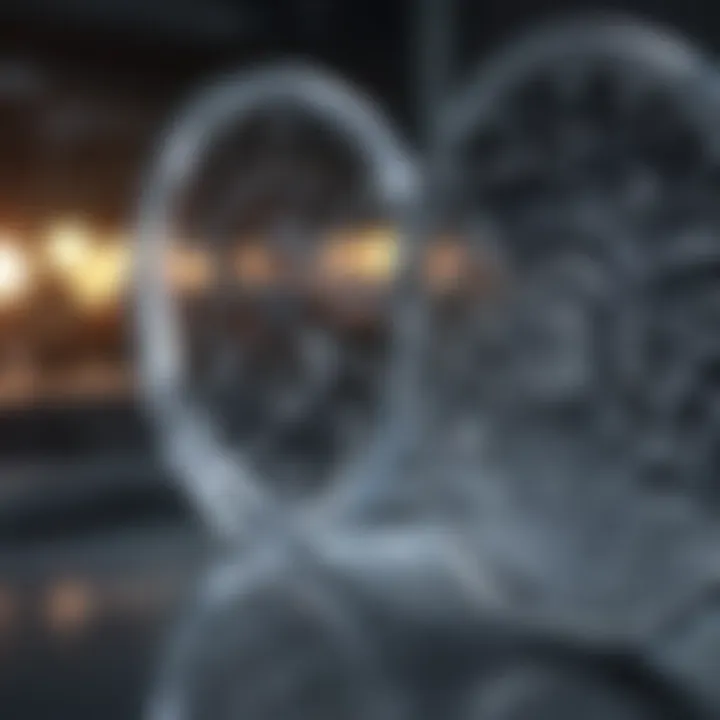
Intro
Data science has become a significant contributor to advancing clinical trials, providing a framework that enhances efficiency, accuracy, and decision-making. As the volume of data generated within the medical research field continues to grow, integrating data science methodologies transforms traditional approaches to clinical investigations. This section will establish a foundation for understanding how data science is incorporated within clinical trials, emphasizing the implications and advancements it brings to patient care.
A focus on data collection methodologies accompanies the integration of statistical models and machine learning applications, enabling researchers to derive meaningful insights from complex datasets. Ethical considerations also come into play, influencing how data is managed and interpreted. Finally, the future landscape of clinical trials will be discussed, spotlighting the impact of big data analytics on the overall efficiency of medical research.
Foreword
In recent years, data science has emerged as a transformative force within the realm of clinical trials. This integration is not just an enhancement but a necessary evolution in how medical research is conducted. The realm of clinical trials relies heavily on accurate data to assess the effectiveness and safety of new treatments. As the complexity of these studies increases, so does the need for sophisticated data handling and analysis.
Moreover, data-driven insights can improve decision-making processes significantly. Clinical trials often face significant challenges, including patient recruitment, retention, and data integrity. Understanding how data science reshapes these areas can lead to better planning and execution of trials. Furthermore, data science enables a more personalized approach to medicine, aligning treatment plans with individual patient needs based on predictive modeling.
"Data science has the potential to reshape outcomes in clinical trials, making the process not only more efficient but also more reliable."
In this article, we will delve into multiple dimensions of data science within clinical trials. From understanding various phases of trials to examining data collection methods and statistical modeling, each aspect is crucial. By outlining these components, we can better appreciate the role of data science in enhancing patient care and advancing medical research. Ultimately, this exploration aims to clarify how data-driven methodologies contribute to more informed decisions and improved outcomes in clinical trials.
Understanding Clinical Trials
Understanding clinical trials is crucial for grasping how data science is applied in medical research. Clinical trials are structured studies that investigate the effectiveness and safety of new treatments, drugs, or medical devices. This section will explore the importance of clinical trials, their phases, and regulatory frameworks.
Phases of Clinical Trials
Clinical trials generally progress through several distinct phases. Each phase has specific goals, methodologies, and outcomes.
- Phase I: This phase focuses on assessing the safety of a new treatment. It usually involves a small group of healthy participants. Here, researchers determine how the drug is metabolized and identify any potential side effects.
- Phase II: In this phase, the emphasis shifts towards evaluating the efficacy of the treatment. It involves a larger group of participants who may have the disease or condition being studied. The data collected here helps assess the optimal dosage and further evaluate the treatment's safety.
- Phase III: This phase involves a larger and more diverse group of participants. The goal is to confirm the treatment's effectiveness, monitor side effects, and compare it with standard or existing therapies. Successful completion often leads to approval by health authorities.
- Phase IV: These studies occur after a treatment has been approved and is on the market. They gather information on long-term effects and effectiveness in broader patient populations.
Understanding these phases aids researchers in navigating the complexities involved in clinical trials, ensuring the incorporation of data science at every step.
Regulatory Framework
The regulatory landscape is a fundamental aspect of clinical trials. Health authorities such as the U.S. Food and Drug Administration (FDA) and the European Medicines Agency (EMA) establish guidelines that ensure research quality and participant safety.
Each phase of clinical trials must adhere to strict protocols, which include preclinical studies, ethical considerations, and continuous monitoring. Regulations dictate the design, conduct, analysis, and reporting of clinical trials.
Incorporating data science into the regulatory framework enhances transparency and accountability through better data management. This is vital since any issues regarding data integrity can lead to significant delays or the rejection of a new therapy.
Common Challenges Faced
Clinical trials are not without challenges. Some common obstacles include:
- Recruitment and Retention: Finding eligible participants and keeping them engaged throughout the trial can be difficult. This can slow down the trial process and increase costs.
- Data Management: The collection, management, and analysis of clinical trial data can overwhelm researchers. Poor data quality, compliance issues, and inconsistent reporting can lead to unreliable results.
- Adverse Effects: Monitoring and addressing any adverse side effects caused by treatments can be a significant concern. Accurate reporting of these effects is essential for patient safety and regulatory compliance.
- Cost: Clinical trials are expensive, and funding can be a significant hurdle. Financial constraints can limit the scope of trials and impact the quality of research.
Addressing these challenges through effective strategies can improve the execution of clinical trials, enhancing the role of data science in developing new medical solutions.
Data science plays a central role in the realm of clinical trials, significantly enhancing the processes involved in medical research. It integrates diverse data sources, accelerates patient recruitment, optimizes trial designs, and improves the accuracy of results. Data science is not just a supplementary tool; it is fundamental to modern clinical trials, affecting how researchers generate hypotheses and analyze their findings.
Definition and Importance
Data science encompasses the methods and technologies used to extract insights and knowledge from large datasets. In clinical trials, this means using statistical analysis, machine learning, and predictive modeling to interpret data effectively. The importance of data science in this context cannot be overstated—it introduces a level of rigor and sophistication that leads to more informed decision-making.
It allows teams to process vast amounts of information, identifying trends and patterns that may not be visible through traditional analytical methods. The ability to analyze real-world data along with clinical data enhances the generalizability of study results. Consequently, this leads to innovations in drug development and treatment strategies, ultimately improving patient care outcomes.
Types of Data Utilized
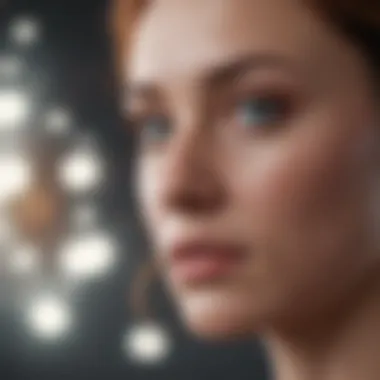
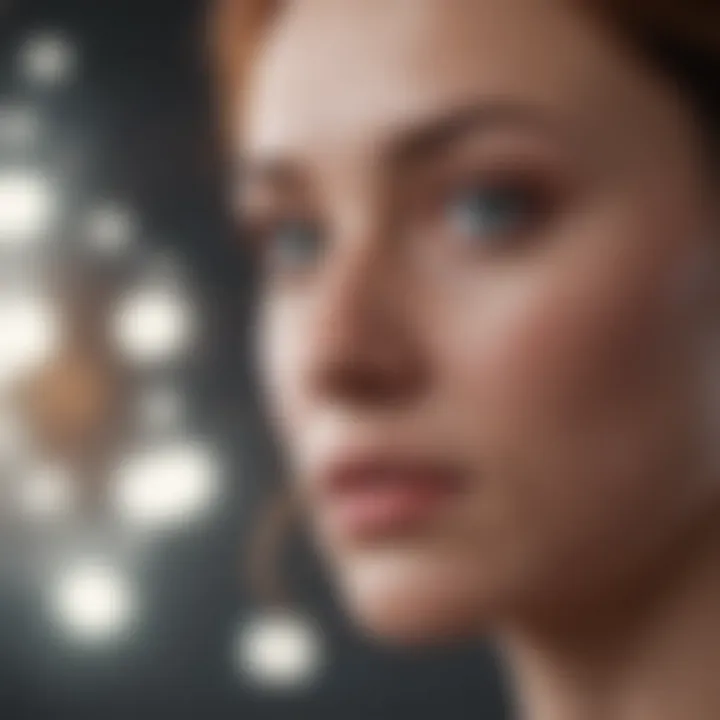
In clinical trials, a wide variety of data types can be harnessed to support objectives. The following are key categories of data utilized:
- Clinical Data: This includes primary data from trials, such as laboratory results, patient demographics, and medical history. It forms the backbone of any clinical trial.
- Electronic Health Records (EHR): These provide comprehensive patient data, enabling researchers to gather additional insights about a participant's medical history and current treatments.
- Real-World Data: This type of data includes information collected outside of traditional clinical settings. It may come from sources such as health apps, social media, and registries. This is valuable for understanding patient behavior and treatment effectiveness in everyday life.
- Genomic and Biomarker Data: As precision medicine grows, genetic data becomes increasingly important in understanding responses to treatment.
- Wearable Device Data: Data from devices that monitor patients' health metrics can provide real-time insights into patients' wellbeing during trials, thus offering richer datasets for analysis.
Utilizing these diverse data types enables deeper insights and more nuanced analyses, which can lead to better design, robustness, and efficiency in clinical trials.
"The integration of diverse datasets enriches the knowledge base of clinical trials, enhancing their reliability and applicability across varied populations."
In summary, the role of data science is crucial in clinical trials. It provides an array of tools and techniques to optimize study designs and interpret complex data landscapes. Through the integration of multiple data sources, researchers can achieve a clearer understanding of patient outcomes and treatment efficacy.
Data Collection Methods
Collecting data effectively is crucial in clinical trials. The type and quality of the data collected can influence study outcomes and the validity of findings. Today, a variety of data collection methods are employed to gather both quantitative and qualitative data from participants. Understanding these methods aids researchers in designing trials that are not only robust but also comprehensive.
Electronic Health Records
Electronic Health Records (EHRs) serve as a rich source of patient information, documenting everything from medical history to treatment plans. They facilitate the streamlined collection of data in real-time, which is vital for monitoring patient responses during trials. Researchers can track adverse events, medication adherence, and other patient behaviors through EHR integrated systems. Moreover, EHRs help reduce the risk of data entry errors while ensuring that regulatory requirements are met.
However, reliance on EHRs also comes with challenges. Data completeness and accuracy can vary among institutions. Standardizing EHR systems remains difficult, impacting data integration across studies. Addressing these issues is essential for maximizing the utility of EHRs in clinical trials.
Wearable Devices and Mobile Apps
The advent of wearable technology and mobile applications has transformed how researchers collect real-time health data. Devices such as fitness trackers and smartwatches can continuously monitor biometric parameters like heart rate and activity levels. This real-time data enhances the understanding of patient experiences and health outcomes throughout the trial.
Mobile apps further enrich data collection by enabling participants to report symptoms, medication intake, or side effects directly. The immediacy of this data can enhance responsiveness to patient needs. Yet, there are considerations regarding data privacy and device reliability. Ensuring consent and understanding participants’ technological proficiency is necessary for effective implementation.
Surveys and Patient-Reported Outcomes
Surveys are an essential tool for capturing patient-reported outcomes (PROs). They allow patients to share their perceptions of treatment effects based on their lived experiences. These insights can be instrumental in understanding the overall impact of an intervention beyond clinical measurements.
Patient surveys encompass various aspects, including quality of life, symptom management, and satisfaction with care. They are particularly valuable in trials for chronic diseases where subjective evaluation is crucial. Despite their advantages, surveys face challenges related to bias and the need for clear, concise questions. Careful questionnaire design is required to minimize misunderstandings and optimize response rates.
Statistical Models in Data Science
Statistical models serve as the backbone of data analysis in clinical trials. They assist researchers in understanding patterns, making predictions, and drawing conclusions from complex datasets. The integration of these models is essential not only for analyzing outcomes but also for ensuring the integrity of the research process. By applying various statistical techniques, researchers can verify hypotheses and improve the quality of their findings.
The importance of statistical models can be summarized in several key points:
- Data Interpretation: These models provide frameworks for interpreting vast amounts of data. Without them, data remains just numbers. Statistical approaches convert raw data into actionable insights.
- Decision Support: They inform decision-making by quantifying uncertainty, thus guiding healthcare professionals in choosing effective treatments based on empirical evidence.
- Risk Assessment: Statistical models facilitate risk assessment in clinical trials. They help to identify factors that increase the likelihood of adverse effects, allowing for better patient safety.
In this article, we will delve deeper into the specific types of statistical models used in clinical trials, starting with Descriptive Statistics.
Descriptive Statistics
Descriptive statistics represent a fundamental element of data analysis. They summarize and describe the essential features of a dataset, offering insights into the distribution, central tendency, and variability of data points. Common descriptive statistics include measures such as mean, median, mode, and standard deviation.
When applied in clinical trials, descriptive statistics can provide a snapshot of the study population, including demographics, treatment outcomes, and any relevant baseline characteristics. This initial understanding is crucial for planning subsequent analyses and ensuring that the study design is appropriate.
For example, a researcher might use descriptive statistics to identify the average age of participants or the proportion of different genders, which can have implications for treatment efficacy.
Inferential Statistics
Inferential statistics go beyond merely describing data; they allow researchers to make inferences about a population based on a sample. This type of statistical modeling enables the establishment of relationships between variables and testing of hypotheses, which can be critical in understanding the effectiveness of treatments.
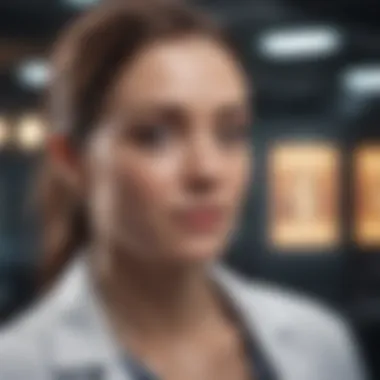
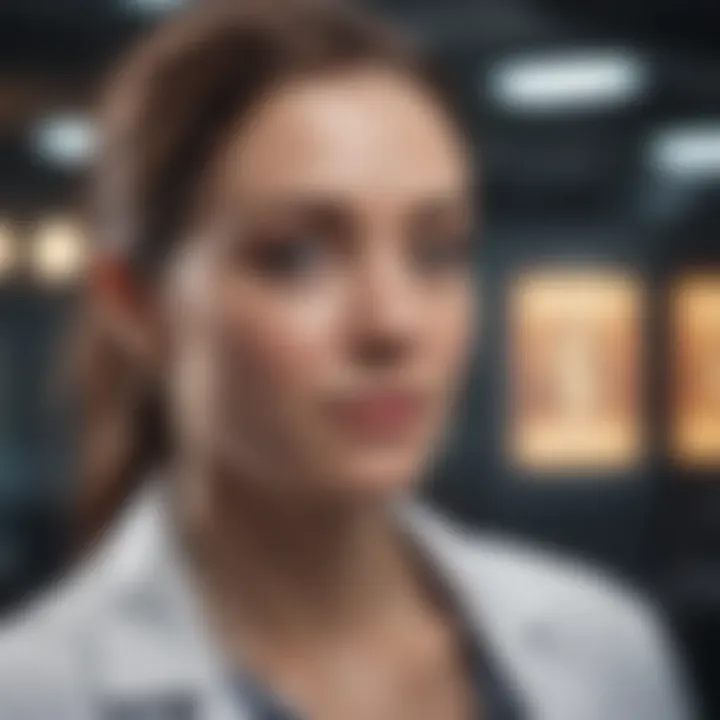
In a clinical trial, inferential statistics enable conclusions to be drawn about greater patient populations, which can guide healthcare practices. Techniques such as t-tests, chi-square tests, and regression analysis are often employed to ascertain whether observed effects are statistically significant or likely due to chance.
This aspect of statistical modeling is vital for evaluating treatment efficacy and safety in clinical trials, as it informs regulatory decisions and clinical guidelines.
Predictive Analytics
Predictive analytics combines historical data with statistical algorithms to forecast future outcomes. In the context of clinical trials, predictive models can identify patterns that may help anticipate how patients will respond to a particular intervention.
For instance, researchers might employ logistic regression to predict the likelihood of successful treatment based on patient characteristics such as age, health status, and previous treatments.
The benefits of using predictive analytics in clinical trials include:
- Improved Patient Outcomes: By identifying the most responsive patient subsets, treatments can be tailored to increase effectiveness.
- Resource Allocation: Efficient allocation of resources can be achieved by predicting how many participants are likely to benefit from a given treatment.
- Early Detection of Failure: Predictive models can signal when a trial is likely to fail, allowing for timely modifications or terminations to waste less time and resources.
The integration of statistical models in data science transforms the clinical trial landscape, enabling better design, execution, and analysis of research studies.
Machine Learning Applications
Machine learning has become a pivotal force in the realm of clinical trials. Its capacity to analyze vast datasets quickly and accurately has transformed traditional research methodologies. This section delves into key applications of machine learning, particularly focusing on risk prediction models and patient stratification. Engaging with these subjects reveals how integrating machine learning can lead to significant improvements in the efficiency and effectiveness of clinical trials.
Risk Prediction Models
Risk prediction models are critical in clinical trials as they allow researchers to forecast potential adverse events in participants. By using past data, these models can identify which patients are at a higher risk for outcomes like non-responsiveness to treatment or severe side effects. The process typically employs various algorithms that can learn patterns from historical datasets.
Key benefits of risk prediction models include:
- Increased Safety: By identifying high-risk individuals, trials can adapt protocols to mitigate risks.
- Resource Optimization: Researchers can allocate resources effectively by focusing on patients who might benefit more from specific treatments, ultimately minimizing unnecessary healthcare costs.
- Enhanced Recruitment Strategies: Understanding risk factors can aid in selecting appropriate patients for studies, improving recruitment success rates.
Machine learning algorithms such as logistic regression, support vector machines, and neural networks are commonly used in developing these models. They adapt over time as more data becomes available, increasing their predictive accuracy, thereby assuring better outcomes in clinical trials.
Patient Stratification
Patient stratification is another major application of machine learning in clinical trials. This process involves segmenting patients into subgroups based on characteristics that influence treatment responses. By doing so, researchers can tailor therapies to specific populations, enhancing the chances of success.
The following are important aspects of patient stratification using machine learning:
- Personalization of Treatment: Different patients respond uniquely to treatments. Machine learning allows for the identification of these differences, facilitating the development of personalized medicine strategies.
- Improved Outcomes: Targeted therapies can lead to higher rates of efficacy since treatments are better suited to the genetic or phenotypic profile of the patient.
- Efficient Design of Clinical Trials: By stratifying patients, trials can be designed to focus on specific demographics or genetic types. This precision not only streamlines the research process but may increase the reliability of the findings.
Ethical Considerations
In the realm of clinical trials, the integration of data science brings forth a series of ethical considerations that must be addressed. These considerations are crucial, given the sensitive nature of health data and the potential implications on patient rights and safety. Researchers must understand the responsibilities they have toward participants, ensuring that their rights are respected while maintaining the integrity of the data collection process.
Data Privacy and Security
Data privacy and security are paramount in clinical trials. The sensitive information derived from participants can include personal details, medical histories, and treatment responses. Therefore, it is imperative to implement robust security measures to protect this data from unauthorized access or breaches. The use of encryption, secure data storage, and limited access to personal information are essential strategies.
Moreover, organizations handling such data must comply with regulatory standards, such as the Health Insurance Portability and Accountability Act (HIPAA) in the United States. Violating these regulations can result in severe consequences for both the institutions and the individuals involved. Ensuring that data privacy is maintained fosters trust between participants and researchers, which is essential for the success of clinical trials.
Informed Consent
Informed consent is a pillar of ethical standards in clinical research. It is vital that participants fully understand the nature of the trial, the risks involved, and how their data will be used. This process should not only include providing detailed information but also ensuring that participants can ask questions and receive clear answers. The consent form must be presented in a language that is easy to understand, avoiding jargon that might confuse potential participants.
Furthermore, it is critical to emphasize that consent is not a one-time event. Participants should feel free to withdraw from the trial at any point without facing any penalties. This aspect of informed consent underscores the voluntary nature of participation, reinforcing the ethical principle of autonomy.
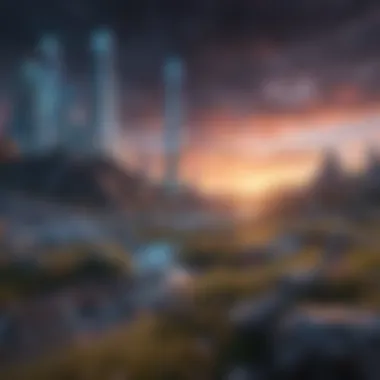
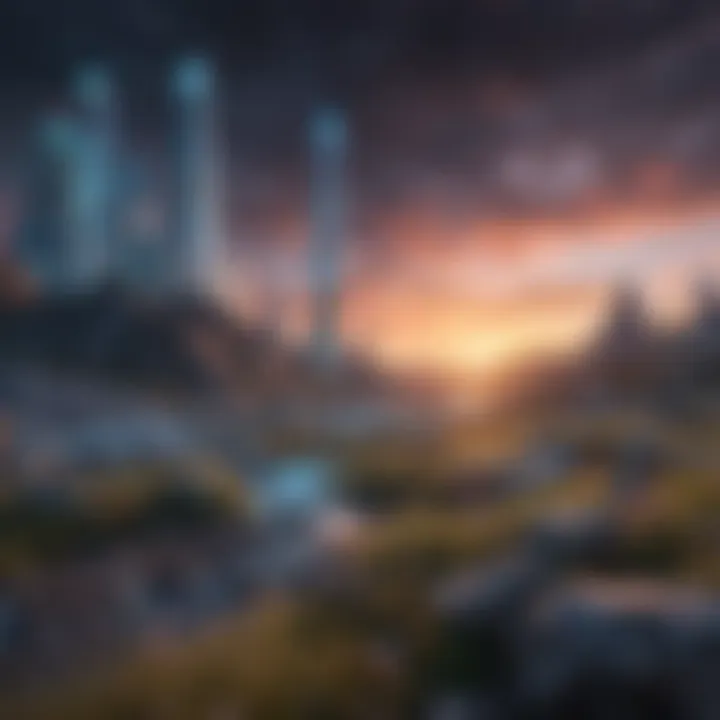
Ethical considerations in clinical trials ensure that participants are treated with respect and dignity, reinforcing trust in the research community.
In summary, ethical considerations in clinical trials, particularly data privacy and informed consent, highlight the delicate balance between advancing medical knowledge and protecting individual rights. A thorough understanding of these elements will facilitate the creation of clinical trials that are not only effective but also ethically sound.
Challenges in Data Integration
Data integration is a critical aspect of clinical trials, particularly with the rise of data science. The ability to combine various data types and sources enhances the efficiency and effectiveness of research. However, significant challenges arise during this process. The following sections delve into two primary issues: data quality and interoperability of systems.
Data Quality Issues
Data quality is paramount in clinical research. Poor quality data can compromise the results and conclusions of any trial. There are several facets to this problem. First, data collection methods vary greatly. For instance, information gathered from surveys may not align with data extracted from clinical systems like Electronic Health Records. Furthermore, disparities among data entry standards often lead to inconsistencies.
Additionally, errors during data entry can worsen the situation. Human mistakes or software malfunctions might introduce inaccuracies that distort findings. Validating the data before analysis is necessary. This ensures that it meets required standards and relevance to the clinical trial objectives.
"High-quality data is crucial for reliable insights in data science applications."
To enhance data quality, researchers can establish protocols that dictate data entry guidelines. Regular audits and monitoring can also help in identifying and rectifying quality issues early on.
Interoperability of Systems
Interoperability refers to the ability of different systems and organizations to work together seamlessly. This is especially important in clinical trials where data flows from various platforms like laboratories, hospitals, and patient monitoring applications. When systems lack interoperability, the integration of data becomes cumbersome and often fails to deliver timely results.
One major challenge is that many health systems use proprietary software. These systems do not communicate well with others. This can lead to siloed information, where valuable patient data remains isolated and unusable. In extreme cases, this might delay critical analysis and hinder timely decision-making in clinical trials.
Finding solutions for interoperability requires a concerted effort across organizations. Adopting open standards for data exchange can greatly improve compatibility among systems. Furthermore, fostering collaboration between tech developers and healthcare providers can yield systems that facilitate easier data sharing.
The Future of Clinical Trials with Data Science
As data science continues to evolve, its role in clinical trials becomes progressively central. The intersection of big data analytics and clinical research is not just about efficiency; it's about reshaping the entire framework of how trials are conducted. As we look ahead, several factors stand out that are likely to define future practices in this domain.
Trends in Clinical Research
The landscape of clinical research is changing. There is an increasing reliance on real-world data. This type of data comes from various sources, such as electronic health records, registries, and patient surveys. It represents the health outcomes of a broad patient population, providing richer insights than traditional clinical trial data.
The integration of advanced data analytics enables researchers to identify trends faster. For example, artificial intelligence can discover patterns in large datasets. This capability offers the potential to predict patient responses to treatment based on historical data. Researchers can thus design more effective and targeted trials, minimizing costs and time.
Moreover, remote monitoring is on the rise. Wearable devices and mobile applications allow for continuous data collection in real-time. Participants can provide data directly from their homes, which leads to better patient engagement. This method can significantly reduce barriers to participation, increasing diversity in trial populations and improving overall validity of results.
"Data science not only helps in the effective management of data but also aids in interpreting outcomes in a more nuanced manner."
Personalized Medicine
Personalized medicine is no longer a future concept; it is actively shaping clinical trials today. With the use of data science, researchers can devise treatments tailored to the individual characteristics of a patient. By analyzing genetic data alongside clinical information, researchers can understand which groups of patients would respond best to specific therapies.
The implementation of personalized approaches can be seen in oncology, where doctors use biomarkers to select treatments suited to the patient’s tumor profile. This advancement underscores the shift from a one-size-fits-all model to more customized healthcare solutions, reflecting the nuances in patient requirements.
Furthermore, personalized medicine fosters a more holistic view of patient care. Data science amplifies this by ensuring the integration of genetic, environmental, and lifestyle factors. Consequently, clinical trials can potentially yield treatments that are not only more effective but also associated with fewer adverse effects.
The End
One of the primary considerations discussed is how data science enables improved decision-making. With the ability to analyze large volumes of data, researchers can identify trends, patterns, and potential outcomes much more effectively. This level of insight not only facilitates better design of clinical trials but also allows for quick adjustments based on real-time data feedback, thereby ensuring patient safety and enhancing the trial's validity.
The benefits of incorporating data science into clinical trials are numerous:
- Enhanced data collection: Accurate and extensive data gathering supports meaningful analysis.
- Statistical rigor: Advanced modeling techniques provide clear insights into the efficacy and safety of treatments.
- Better patient stratification: Machine learning algorithms assist in identifying patient subgroups that may respond differently to treatments, thus fostering personalized medicine.
Moreover, ethical considerations, particularly around data privacy and informed consent, underscore the need for a thoughtful and stringent approach to data handling. The responsibility of researchers to safeguard participants’ information cannot be overstated. This forms a foundational pillar that supports trust in clinical research.
As we look to the future, the transformative impact of data science on clinical trials is clear. With ongoing advancements in technology and methods, the landscape of clinical investigation will continue to evolve. The promise of personalized medicine and insights drawn from vast datasets will likely redefine therapeutic approaches, ultimately leading to better patient outcomes.