Decoding PPMI Data: Insights for Researchers
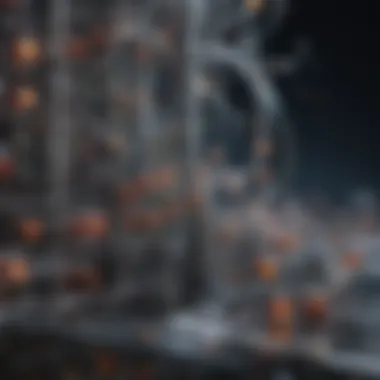
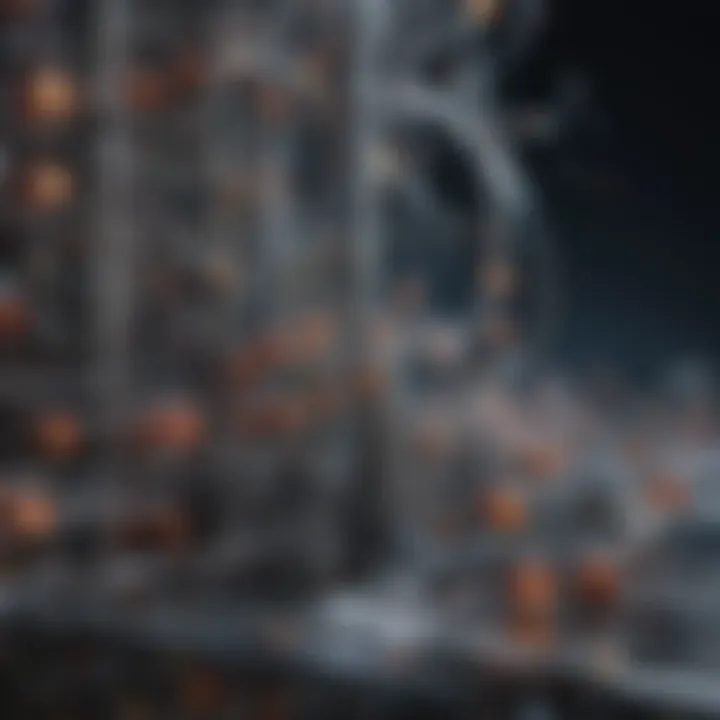
Intro
In the ever-evolving landscape of scientific research, PPMI data plays a crucial role across varied fields. Understanding this data is essential for students, educators, and researchers aiming to leverage its potential insights.
PPMI, or the Parkinson’s Progression Markers Initiative, focuses on collecting and analyzing data related to Parkinson's disease. The importance of this data extends beyond its origins. It is a sophisticated resource that enables researchers to explore nuanced aspects of Parkinson's, allowing them to develop better treatment strategies and understand disease progression.
This article seeks to unpack the intricacies of PPMI data, guiding the reader through its origins, methodologies of collection, interpretation, challenges encountered, and implications of the findings. By doing so, it aims to present a holistic view of PPMI data, ultimately enhancing the researcher's arsenal with valuable knowledge.
Research Overview
The exploration of PPMI data opens a window into the potential of understanding Parkinson's disease deeply. Some key findings have emerged from this data:
- Patient Profiles: Detailed demographics are available, showing how different populations are affected by Parkinson’s disease.
- Biomarkers: The correlation between biological markers and disease progression has been documented clearly.
- Treatment Outcomes: Insights into how various treatments impact patient health over time can provide critical information for future studies.
The significance of this research lies within the wider field of neurology and neurodegenerative diseases. By collating extensive data on Parkinson’s disease, researchers can foster breakthroughs in treatment, thereby improving the quality of life for many individuals.
Methodology
Description of the Analytical Methods Used
PPMI data collection employs a multi-faceted approach. Researchers utilize both qualitative and quantitative methodologies:
- Qualitative Methods: Interviews and surveys help paint a comprehensive picture of patient experiences and challenges.
- Quantitative Methods: Statistical analyses and computational models are used to interpret complex datasets.
Sampling Criteria and Data Collection Techniques
Sampling for PPMI data involves precise criteria to ensure robust findings:
- Participants are often selected based on specific medical histories and disease stages.
- Data is collected through various means, including clinical assessments, brain imaging, and genetic testing.
Data consistency is essential. Thus, rigorous protocols are in place to maintain integrity throughout the data collection process.
"Collecting and interpreting PPMI data is intricate, requiring attention to detail and consistency."
By understanding these methodologies, we set the stage for a deeper exploration of PPMI data's implications in scientific research.
Intro to PPMI Data
The study of PPMI data holds significant importance in the realm of scientific research. Understanding this topic allows students, educators, researchers, and professionals to appreciate how PPMI data contributes to a broader understanding of various scientific phenomena. The clarity of this data directly influences the validity of research results, making it a crucial aspect of investigative methodologies.
Definition of PPMI Data
PPMI stands for Positive Psychology Movement Initiative, which collects data focused on the positive aspects of human behavior. This data encompasses a range of variables, including psychological assessments, behavioral observations, and demographic information. The objective is to enhance insights into human well-being, study development, and psychological resilience. In essence, PPMI data serves as a repository of critical information that can be used for statistical analysis and interpretation.
Historical Context
The journey of PPMI data began in the early 21st century as researchers aimed to shift the focus from pathology to positive psychology. Initial projects laid the groundwork for structured data collection methods. Over time, collaboration among institutions and researchers has expanded PPMI data's scope. It is now used extensively in fields like psychology, education, and even healthcare. The historical evolution of this data illustrates a growing recognition of the significance of positive psychological factors in assessing human behavior.
PPMI data reflects a paradigm shift in psychology, promoting a proactive approach to studying human strengths rather than just addressing weaknesses.
This historical context not only provides a backdrop for current research frameworks but also illustrates how the evolution of PPMI data has contributed to our growing understanding of human behavior and resilience.
Structure of PPMI Data
The structure of PPMI (Prevention of Progression in Parkinson’s Disease) data is vital for understanding its use and application in research. A clear structure allows researchers to efficiently analyze and interpret data, leading to meaningful conclusions. This organization encompasses essential elements that define how data can be used, shared, and understood. Developing a deep comprehension of this structure can facilitate the scientific community in maximizing the potential of PPMI data in their studies and applications.
Key Components
The key components of PPMI data are foundational to its overall structure. They provide a systematic arrangement of information that influences how researchers engage with and utilize the data. Some primary components include:
- Subject Information: Includes demographics, medical histories, and participant consent details. This is crucial to ensure the validity and ethical usage of the data.
- Clinical Assessments: Regular assessments, including neurological evaluations, cognitive tests, and lifestyle surveys, contribute to a comprehensive understanding of how Parkinson’s disease affects individuals.
- Biomarker Data: This component offers insights into biological markers vital for understanding disease mechanisms. Blood samples, imaging data, and other biological indicators help chart disease progression over time.
Each of these components carries its relevance, contributing to a holistic view of the participant’s health and disease trajectory. Researchers must carefully consider these aspects during data collection and analysis to preserve the integrity of their findings.
Data Formats
Data formats are another crucial aspect of the PPMI structure. Different formats can affect how data is stored, shared, and processed. Common formats found in PPMI data include:
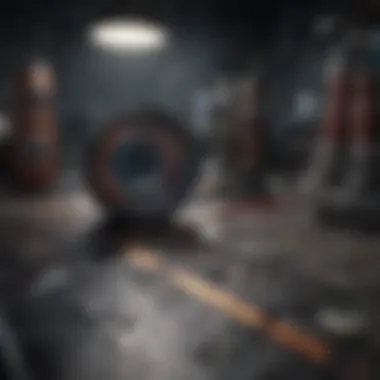
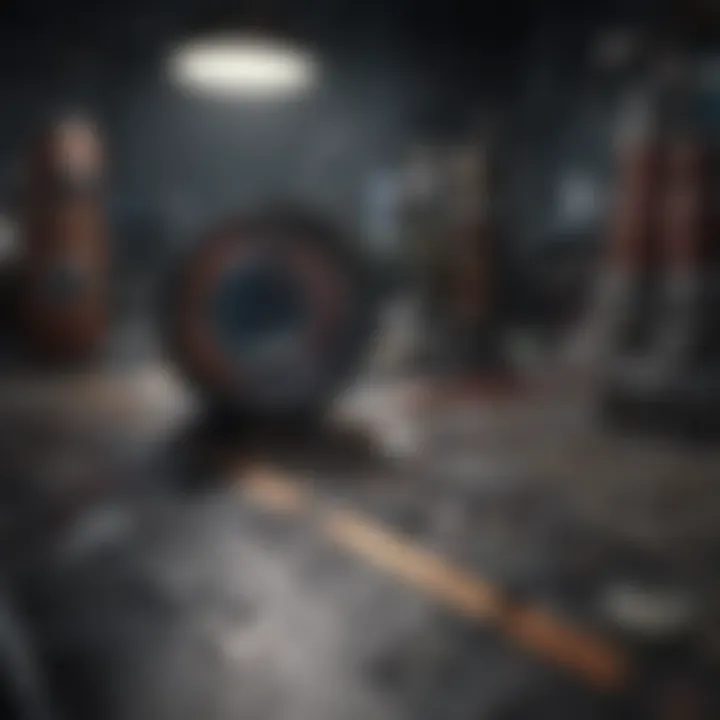
- CSV (Comma-Separated Values): Frequently used for exporting data from databases, it allows for easy data manipulation and analysis in software like Excel or R.
- DICOM (Digital Imaging and Communications in Medicine): This format is standard for handling, storing, and transmitting medical imaging information. It is vital for the analysis of neuroimaging data.
- FHIR (Fast Healthcare Interoperability Resources): This is an emerging standard in healthcare data exchange, designed to support interoperability among different healthcare systems, enhancing its utility in diverse research settings.
The choice of data format can significantly impact analysis capabilities and collaboration across research groups. By understanding these formats, researchers can better integrate PPMI data into their workflows, ensuring accurate and insightful results.
In summary, recognizing the structure of PPMI data, including its key components and formats, can provide critical insights into its use. This understanding is not just beneficial, but necessary for researchers aiming to contribute effectively to the body of knowledge surrounding Parkinson’s disease.
Importance of PPMI Data in Research
PPMI data plays a crucial role in the landscape of modern research. Its significance is not merely based on its availability but on how it enriches the understanding of various phenomena across multiple disciplines. Researchers utilize PPMI data to navigate the intricacies of their studies and address complex questions that traditional data sources may not effectively resolve. This section delves into the applications of PPMI data in scientific studies and its wider implications for policy and decision-making.
Applications in Scientific Studies
PPMI data is instrumental in numerous scientific arenas, providing researchers with a robust foundation for their investigations. In biological research, for instance, PPMI data helps in tracking changes in physiological responses. This data often informs studies that examine the effects of environmental factors on health outcomes. Moreover, in fields like pharmacology, researchers can analyze how certain treatments impact patient populations, ultimately aiding in the development of tailored therapies.
Specific Applications of PPMI Data Include:
- Epidemiological Studies: Assessing the spread and control of diseases by analyzing demographic, environmental, and genetic factors.
- Clinical Trials: Evaluating the efficacy of new drugs and interventions, while ensuring that results are valid and generalizable.
- Public Health Programs: Informing strategies for disease prevention and health promotion based on comprehensive data analysis.
"PPMI data provides a rich context for understanding complex biological and social systems. It is not just data; it is a key to interpreting the unknown."
Impact on Policy and Decision Making
The implications of PPMI data extend beyond the laboratory and into the realm of public policy. Decision-makers in health and environmental sectors rely on informative data analyses to shape policies that affect communities at large. By leveraging PPMI data, policymakers can better forecast trends, allocate resources, and implement effective interventions.
Key Impacts Include:
- Resource Allocation: Data-driven insights aid governments and organizations in distributing resources where they are most needed.
- Policy Formation: Evidence derived from PPMI data can guide the formulation of health policies, resulting in improved outcomes.
- Public Awareness Campaigns: Designing initiatives aimed at specific groups based on comprehensive data assessments enhances effectiveness.
In summary, the importance of PPMI data in research cannot be overstated. Its relevance in scientific studies allows for more thorough investigations, and its impact on policy and decision-making fosters informed action that can lead to significant societal advancements.
Methodological Approaches to PPMI Data Collection
The methodological approaches to PPMI data collection are significant for ensuring accuracy, reliability, and validity of the research outcomes. These methods dictate how data is gathered and how robust it is for scientific inquiry. Understanding these methodologies provides clarity on the precision with which researchers can operate within the PPMI framework. Effective data collection is foundational to any research project; inadequate methods can undermine even the most rigorous theoretical frameworks.
Sampling Techniques
The choice of sampling techniques is crucial in PPMI data collection. It defines the demography and diversity of the sample used in studies, impacting the generalizability of the findings. Common sampling methods include random sampling, stratified sampling, and cluster sampling.
- Random Sampling ensures every individual has an equal chance of being selected. This mitigates bias and helps create a true representation of the population.
- Stratified Sampling involves dividing the population into subgroups and sampling from each group. This is useful for ensuring all segments are adequately represented, especially in varied populations.
- Cluster Sampling is often used when the population is too large and spread out. Researchers can randomly select clusters of participants, easing logistical challenges while maintaining diversity.
Each of these methods has its own strengths and weaknesses. The selection must align with the research objectives and characteristics of the population involved, thus increasing the overall integrity of the data.
Data Recording Procedures
Proper data recording procedures are essential in the PPMI data collection process. They outline how data is documented, stored, and managed. The correctness in these procedures can directly influence the quality and usability of the data.
Data recording may involve:
- Digital Data Entry where researchers input collected data into databases or spreadsheets. It is critical to have checks in place to minimize errors during this phase.
- Manual Recording traditionally utilizes paper forms. This process can be prone to human error but remains necessary in certain contexts or environments where technology is unavailable.
- Automated Data Collection tools such as mobile applications can streamline data entry, reduce errors, and enhance real-time data availability. They often come with features that validate data as it is being entered.
Adopting stringent procedures will ensure that the data remains authentic and useful for future analysis.
Important Note: Ensuring clear recording procedures is a safeguard against data loss, which can significantly affect research outcomes.
In summary, well-defined methodologies, specifically in sampling techniques and data recording, directly correlate to the reliability of PPMI data. They are integral to conducting quality research across various scientific disciplines.
Challenges in PPMI Data Interpretation
The interpretation of PPMI data presents several challenges that could potentially compromise the validity of research findings. These challenges are critical to address, as they impact the reliability and utility of data across scientific fields. The importance of recognizing and navigating these obstacles cannot be overstated. Accurate interpretation of PPMI data is essential for drawing valid conclusions and making informed decisions in research and policy-making.
Data Integrity Issues
Data integrity refers to the accuracy and consistency of data throughout its lifecycle. In the realm of PPMI data, ensuring integrity is vital. Data integrity issues can arise from various sources, including data collection methods, storage, and transmission processes.
- Contamination During Collection: When gathering data, researchers must adhere to strict protocols. Any deviation can introduce noise into the data. For example, using improper sampling techniques may yield results that are not representative of the target population.
- Human Error: Mistakes can occur during data entry and analysis. Inconsistent data entry can lead to inaccuracies, affecting overall study outcomes.
- Technological Failures: Equipment malfunctions or software bugs can corrupt data sets. These failures can go undetected unless robust validation checks are in place.
- Inappropriate Data Handling: Mismanagement of data in storage can lead to loss or alteration. This is particularly pertinent in long-term studies where data is collected over many years.
It is important to implement strong protocols and use reliable technology to maintain data integrity. Regular audits and checks can help ensure that data remains trustworthy.
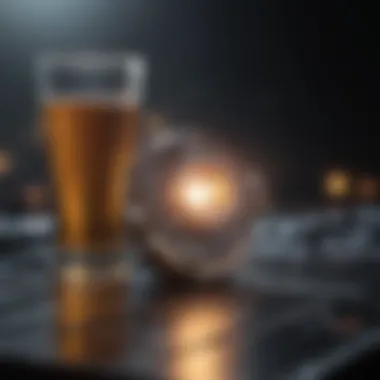
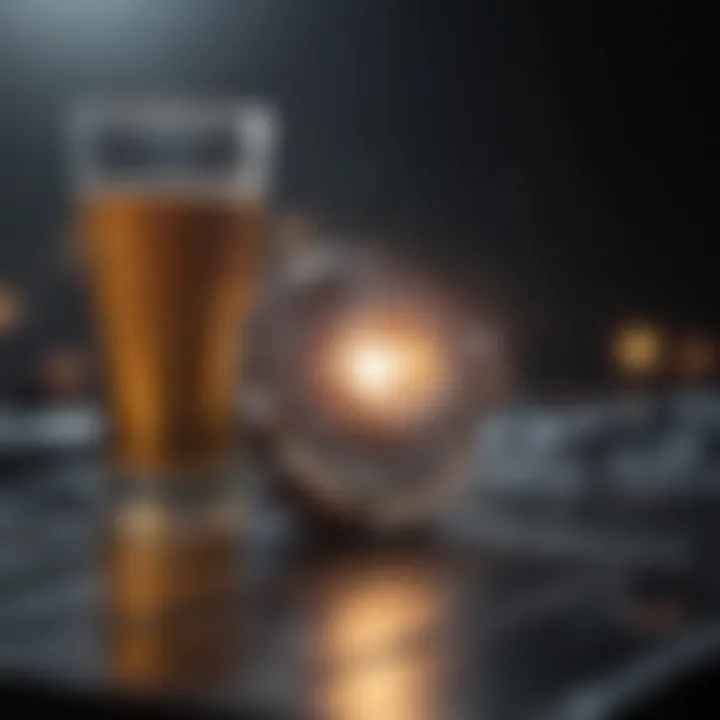
Bias and Error Considerations
Bias and error are two intertwined concepts that can significantly hinder the interpretation of PPMI data. Both can result in misleading conclusions, so it is crucial to understand their impacts thoroughly.
- Selection Bias: If the sample used in the study is not representative of the broader population, the findings may be skewed. Researchers must strive for random sampling to mitigate this risk.
- Measurement Error: This type of error occurs when the data collection instruments are faulty or when the respondents do not understand the questions as intended. For example, if a survey question is ambiguous, the responses may not reflect true opinions or behavior.
- Confirmation Bias: Researchers can unintentionally focus on data that supports their hypotheses while neglecting data that contradicts their views. Keeping an open mind during the analysis process is essential for objective interpretation.
- Misinterpretation of Statistical Significance: Common misunderstandings surrounding p-values and confidence intervals can lead researchers to draw incorrect conclusions from their analyses. Educating researchers about statistical concepts can mitigate this known issue.
"Awareness of biases and errors is essential for improving the interpretative accuracy of PPMI data."
Statistical Analysis of PPMI Data
Statistical analysis of PPMI data is crucial for several reasons. It allows researchers to derive meaningful insights from vast amounts of data collected in various studies. The ability to process and interpret this data helps in drawing valid conclusions, identifying trends, and guiding future research directions. Further, skilled statistical analysis can enhance the reliability of findings and bolster the credibility of research efforts.
Techniques and Tools
Several techniques and tools are employed when conducting statistical analysis of PPMI data. As the data can vary in type and format, applying appropriate methods is essential. Common techniques include:
- Descriptive Statistics: These summarize and describe the main characteristics of the dataset. Measures such as mean, median, mode, and standard deviation offer insights into central tendencies and variations.
- Inferential Statistics: This involves making predictions or inferences about a population based on sample data. Techniques include hypothesis testing, confidence intervals, and regression analysis.
- Multivariate Analysis: When dealing with complex datasets that contain multiple variables, multivariate techniques such as factor analysis or cluster analysis become useful.
Tools that support these techniques often include:
- R: A powerful programming language and environment for statistical computing.
- Python: Its libraries, such as Pandas and SciPy, are widely used for data manipulation and statistical analysis.
- SPSS: A software package designed for interactive or batched statistical analysis.
- MATLAB: Used for numerical computing and often utilized in advanced data analysis tasks.
Interpreting Statistical Results
Interpreting statistical results requires a careful approach. The results generated from statistical analyses tell a story about the data but need context to fully understand their implications. Key considerations when interpreting these results include:
- Statistical Significance: Results that demonstrate a statistically significant relationship indicate that the findings are unlikely due to chance. Common thresholds for significance include p-values less than 0.05 or 0.01.
- Effect Size: This quantifies the size of the difference or relationship. It provides insight into the practical significance of the findings, beyond mere statistical significance.
- Confidence Intervals: These give a range in which the true parameter is expected to lie. Wider intervals may denote less certainty in the estimate.
Researchers should avoid overextending conclusions beyond what the data supports. Misinterpretation can lead to erroneous claims and ultimately affect the validity of research outcomes. "Less is more" is a keen reminder when dealing with data.
Statistical analysis is not just about crunching numbers; it is about answering questions and making sense of the uncertainty inherent in research.
In summary, mastering statistical analysis of PPMI data is essential for researchers wanting to contribute valuable insights to their fields. By utilizing appropriate techniques and tools, alongside careful interpretation, one can maximize the value of PPMI data.
PPMI Data in Different Scientific Disciplines
Understanding PPMI data is crucial across various scientific fields. Its multifaceted nature allows for diverse applications. In biological sciences, researchers can track patient outcomes, genetic variations, and the effectiveness of treatments. In chemistry and environmental studies, this data aids in monitoring chemical substances and their environmental impact. Physics applications benefit from PPMI data for analysis in experimental designs and theoretical models. Each discipline utilizes PPMI data to generate insights, ultimately enhancing scientific knowledge and informing practices.
Biological Sciences
In biological sciences, PPMI data is particularly valuable for observing the progression of diseases such as Parkinson's. This data provides essential insights into patient demographics, symptomatology, and treatment responses. Comprehensive PPMI datasets empower researchers to correlate clinical outcomes with biological markers, enabling personalized medicine approaches.
Key aspects include:
- Understanding disease mechanisms
- Identifying potential biomarkers
- Evaluating the efficacy of novel treatments
Moreover, the longitudinal data available allows for analyzing patient trajectories over time, contributing to improved therapies and care protocols. Researchers can also share findings collaboratively, broadening the impact of PPMI data across the scientific community.
Chemistry and Environmental Studies
In the realm of chemistry and environmental studies, PPMI data serves as a backbone for studies relating to environmental toxins, chemical behavior, and risk assessments. Researchers leverage PPMI data to construct models predicting the behavior of chemicals in various environments, which is vital for regulatory compliance and public health goals.
Relevant considerations include:
- Tracking chemical exposure levels
- Evaluating environmental impacts of pollutants
- Developing better assessment tools for risk management
This data facilitates extensive field studies and enables scientists to draw correlations between chemical exposure and health outcomes. Therefore, PPMI data enhances both the quality of scientific inquiry and the safety measures implemented worldwide.
Physics Applications
Within physics, PPMI data is often integrated into experimental designs. It provides quantitative analysis that is vital for validating theoretical predictions. Researchers apply statistical tools to analyze the trends and results derived from experiments, allowing for critical evaluations of hypotheses.
The benefits of utilizing PPMI data in physics include:
- Enhancing predictive modeling
- Validating experimental results
- Informing future research directions
By using this robust data, scientists can refine theories and push the boundaries of current knowledge. The rigorous analysis enabled by PPMI data can lead to breakthroughs in various physical phenomena, ultimately advancing the field.
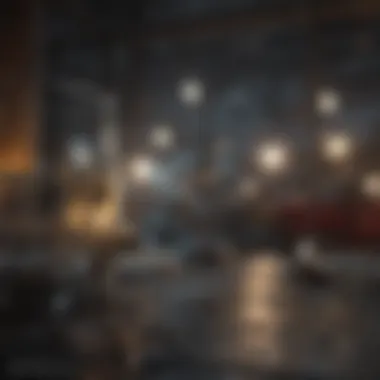
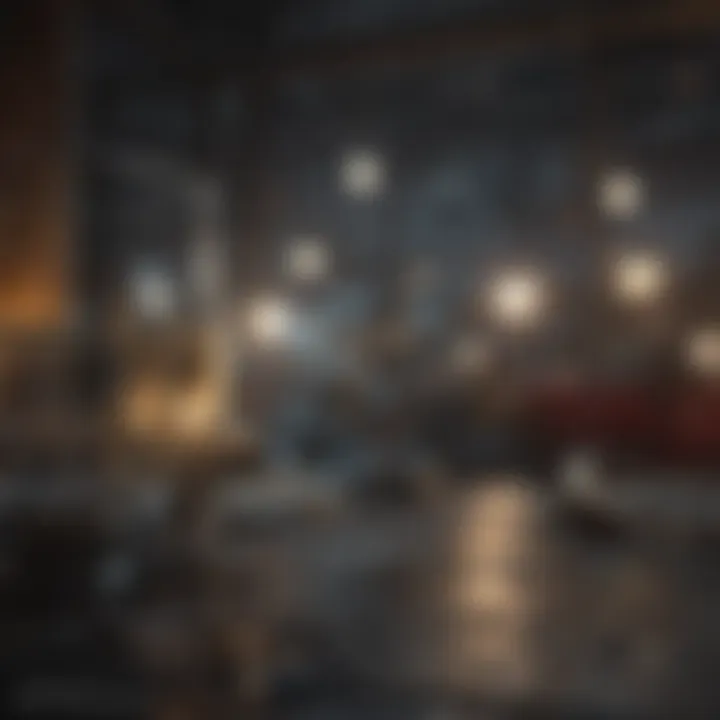
Case Studies Utilizing PPMI Data
Case studies that involve PPMI data play a crucial role in demonstrating its practical application across various scientific fields. These real-world examples provide valuable insights into how PPMI data can influence research outcomes, policy formulations, and further discussions among academics and practitioners. The importance of such case studies cannot be understated. They serve as evidence of the effectiveness of data-driven approaches, showcasing the tangible effects of theoretical frameworks brought to life through investigation.
Significant Research Projects
Research projects employing PPMI data can be expansive and multifaceted. These projects often explore a range of scientific questions, some focusing on understanding disease mechanisms while others might analyze environmental impacts due to certain industrial practices. For instance, studies aimed at uncovering the genetic factors of neurodegenerative diseases frequently utilize PPMI data. This data can delineate how genetic predispositions affect patient outcomes and help identify specific biomarkers that can lead to better-targeted therapies.
Another project might utilize PPMI data to examine the effects of air quality on public health. By correlating health records with environmental data, researchers can uncover the links between pollution levels and respiratory diseases, allowing for informed policy-making that addresses community health concerns.
Key Findings and Their Implications
The findings generated through PPMI data analysis provide profound implications for both policy and practice. For example, if findings from a research project indicate a strong link between a particular genetic marker and the onset of a disease, it could lead to a shift in screening protocols. Similarly, results from environmental studies demonstrating the correlation between poor air quality and increased health care costs might prompt governments to implement stricter regulations on emissions.
The implications of such research extend further, as they also advocate for public awareness. When the community understands the potential health risks associated with environmental issues or genetic factors, it fosters a culture of knowledge that can encourage proactive health behaviors.
"The true value of PPMI data lies in its ability to inform decisions that can enhance collective well-being."
In summary, case studies utilizing PPMI data are not merely academic exercises. They reflect the interconnectedness of data collection, analysis, and real-world application. As such, they hold the potential to advance scientific understanding and facilitate significant changes in policy and practice across diverse industries.
Ethical Considerations in PPMI Data Use
The ethical aspects of utilizing PPMI data are critical to ensuring responsible research practices. As data becomes more central to scientific inquiry, it is imperative to navigate the ethical maze surrounding data collection, usage, and sharing. The implications of mishandling data can affect personal privacy and public trust, which emphasizes the need for ethical scrutiny throughout the research process. Engaging with these considerations is not just a legal obligation; it is also a moral imperative for advancing knowledge while safeguarding the rights of individuals.
Data Privacy Issues
Data privacy is a foremost concern in the realm of PPMI data usage. The information derived from such datasets often contains sensitive details that can identify participants. When utilizing this data, researchers must ensure that personally identifiable information (PII) is adequately protected. This can involve anonymizing data, ensuring secure storage, and limiting access to authorized personnel only. Failure to address these privacy issues can lead to harmful consequences, including breaches of confidentiality and loss of participant trust.
Researchers should adopt a clear data management strategy that emphasizes the principles of data minimization, meaning that only the data necessary for the study should be collected. Moreover, they must clearly communicate to participants how their data will be used, stored, and shared. This transparency is essential in fostering trust and ensuring compliance with regulatory standards, such as the General Data Protection Regulation (GDPR) in Europe.
"Protecting participant data integrity directly correlates with the successful implementation of ethical research practices."
Consent for Data Usage
Obtaining explicit consent from participants is another fundamental aspect of ethical considerations when using PPMI data. Informed consent involves providing potential participants with comprehensive information about the study, its purpose, methods, and any potential risks involved. Participants should not only understand but also accept how their data could be utilized. This respect for autonomy ensures that individuals have agency over their personal information.
Moreover, consent must be renewed and revisited as new research questions arise or methodologies change. This continuous dialogue with participants fosters a culture of respect and reinforces the ethical framework within which researchers operate. Ethical violations related to consent can lead to severe consequences, undermining the credibility of research findings and endangering future studies. In summary, respecting participant consent is vital for maintaining ethical integrity.
Future Directions for PPMI Data Research
In an era where data-driven decision making defines the landscape of research, understanding the future directions of PPMI (Physical Performance Management Indicator) data is crucial. This segment of the article delves into emerging technologies and potential advancements in methodologies that can significantly enhance the use of PPMI data. The importance lies not only in improved accuracy but also in expanding the capacity for researchers to interpret and utilize the data effectively.
Emerging Technologies
Emerging technologies are at the forefront of transforming research practices, including the domain of PPMI data. Advances in artificial intelligence, machine learning, and data analytics have already begun to influence how researchers collect, store, and analyze PPMI data.
- Artificial Intelligence contributes to predictive analytics, enabling researchers to anticipate trends and patterns in PPMI data.
- Machine Learning algorithms can identify complex relationships within the data that might not be visible through traditional analysis methods.
- Cloud Computing allows for vast storage capacities and facilitates collaborative research efforts across different geographical locations.
These technologies enhance not just the efficiency of data analysis but also the ability to draw actionable insights from the vast resources of PPMI data. Integrating real-time data collection through mobile devices and wearables also adds a dynamic aspect that can lead to more timely and relevant research outcomes.
"Emerging technologies have the power to remodel our understanding and application of PPMI data, driving innovation forward."
Potential Advancements in Methodologies
Methodological advancements play a vital role in refining how PPMI data is approached. As research evolves, methodologies must adapt to integrate the new technologies that emerge.
- Interdisciplinary Approaches: Combining methodologies from various fields such as psychology, biomechanics, and informatics may enable a fuller understanding of data utility.
- Flexible Frameworks: Developing frameworks that accommodate the unique contexts of different research environments can enhance data relevance.
- Participatory Research Models: Engaging subjects in the research process can lead to better data collection methods and ethical considerations.
These advancements will likely result in enhanced data quality, greater participant engagement, and more relevant research findings. Overall, it presents a pathway towards a more nuanced understanding of PPMI data that aligns with contemporary research needs.
With continued innovation and exploration in both technology and methodologies, the future of PPMI data research holds significant potential. As scholars and practitioners navigate this evolving landscape, it will be essential to remain open to the changing dynamics and to lead with curiosity and rigor.
Finale
Summary of Key Points
The article illuminated several critical aspects of PPMI data:
- Definition and Structure: We dissected what PPMI data entails and its foundational components.
- Importance in Research: The role of PPMI data in scientific inquiry and its implications on policy-making was emphasized.
- Methodologies and Challenges: We delved into how PPMI data is collected and the potential challenges in its interpretation.
- Future Directions: Emerging technologies and advancements in methodologies were discussed, indicating a promising future for PPMI research.
Final Thoughts on PPMI Data
PPMI data serves not only as a fundamental resource in various scientific domains but also as a catalyst for innovation and development. As research continues to evolve, the nuances of correctly utilizing this data will determine its impact. It is imperative that stakeholders remain vigilant concerning ethical concerns, data privacy, and informed consent. In this manner, the true benefit of PPMI data can be realized, ushering in advancements that resonate across disciplines and contribute to the greater good.