Deep Learning Innovations in Medical Imaging
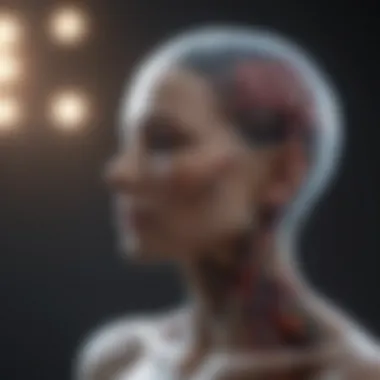
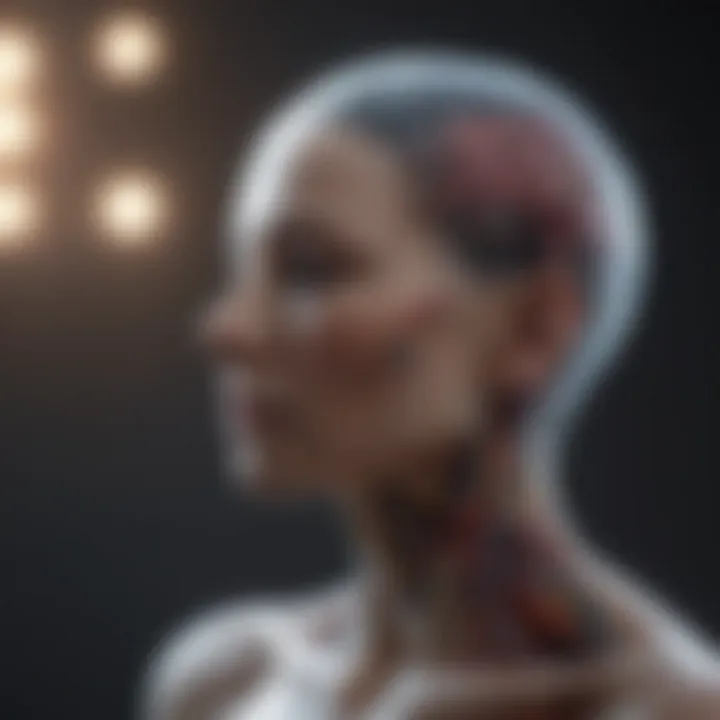
Intro
Deep learning is a rapidly evolving domain within artificial intelligence, gaining significant traction in the field of medical imaging. Its ability to analyze vast amounts of data with high accuracy opens new possibilities in diagnostics and patient care. The potential of deep learning technologies has led to their integration into various aspects of medical imaging, reshaping traditional methodologies and enhancing the accuracy of diagnoses.
The integration of deep learning in this sector focuses primarily on its ability to process and interpret imaging data. This capability elevates diagnostic accuracy, optimizes image processing techniques, and ultimately improves patient outcomes. The following sections will delve into the current advances in deep learning applications within medical imaging, examining its implications on healthcare.
Research Overview
Summary of key findings
Research in deep learning has produced promising results. For instance, convolutional neural networks (CNNs) have shown remarkable effectiveness in detecting diseases from imaging data, such as identifying tumors in X-rays and magnetic resonance images. The use of deep learning also enhances the speed and efficiency of image analysis, significantly minimizing human error.
Additionally, models like the U-Net architecture have been beneficial in segmenting medical images for better visualization of anatomical structures. The incorporation of deep learning algorithms paved way for automated systems that significantly outperform traditional image analysis methods.
Importance of the research in its respective field
The significance of deep learning research cannot be understated. As diagnostic tools become more advanced, they influence patient management and treatment options. Accurate imaging supported by deep learning aids in detecting diseases at an earlier stage, potentially leading to better prognoses.
Moreover, advancements in this field foster innovations in personalized medicine. Improved imaging techniques can help tailor treatments to the individual needs of each patient.
"Deep learning has the potential to dramatically transform healthcare. With its implementation, we can expect enhanced accuracy in diagnostics, enabling targeted patient care."
Methodology
Description of the experimental or analytical methods used
Research on deep learning in medical imaging employs a variety of analytical techniques. The foundation largely rests on supervised learning, where deep learning models are trained using labeled datasets. This allows the algorithms to learn specific features from the images that correlate with various medical conditions.
Techniques such as data augmentation are frequently used to improve the robustness of algorithms. This process generates modified versions of training data, allowing the model to learn about variations in imaging conditions.
Sampling criteria and data collection techniques
Data collection typically involves large datasets obtained from hospitals and medical institutions. These datasets must be carefully curated to include diverse samples that represent different demographics and disease states. The quality of the data is crucial since it directly impacts the model’s performance.
It is essential to ensure that the datasets are representative and free from biases to avoid skewed results and enhance the reliability of the models trained on them.
Preface to Deep Learning in Medical Imaging
Deep learning has emerged as a transformative force in the field of medical imaging. Its influence extends beyond mere technology; it stands as a critical development that amplifies diagnostic capabilities and enhances overall patient care. In this section, we illuminate the significance of deeply understanding how deep learning integrates within medical imaging.
The use of deep learning algorithms in medical imaging brings forth remarkable improvements in accuracy and efficiency. These algorithms have the ability to analyze vast amounts of data, identifying patterns and anomalies in images that might be overlooked by human eyes. This capability is vital in a healthcare environment where time and precision are crucial. As diagnostic imaging relies heavily on software to process and scrutinize numerous images, integrating deep learning can reduce the workload on healthcare professionals and facilitate quicker diagnoses.
Moreover, deep learning allows for better personalization of medical care. By tailoring algorithms to recognize specific conditions, healthcare providers can enhance the precision of diagnoses and, subsequently, treatment plans that suit individual patient needs. This individualized approach fosters improved patient outcomes and enhances the overall reliability of medical imaging.
Understanding deep learning in medical imaging also opens avenues for addressing significant healthcare challenges, such as reducing diagnostic errors and enhancing image resolution. With these capabilities, the potential for improved patient safety and clinical outcomes becomes more pronounced, underscoring the relevance of deep learning in today’s medical landscape.
Preventing human error and ensuring consistent results is inherently valuable; here, deep learning algorithms show their strength. They can serve as an additional line of defense in diagnostics, making them an essential tool in modern medicine. As we delve deeper into this topic, it becomes clear that the integration of deep learning into medical imaging is not merely an option; it has become a necessity for progressive healthcare.
Definition of Deep Learning
Deep learning, a subset of machine learning, employs algorithms known as artificial neural networks. These networks are designed to simulate the way human brains operate, permitting machines to learn from massive datasets. In medical imaging, deep learning enables systems to extract meaningful features from images autonomously, without explicit programming for each task.
With deep learning, the training phase involves feeding the system numerous examples of images, allowing it to identify patterns through layers of computation. This process makes it adept at recognizing various medical conditions depicted in the images, from tumors to fractures. Thus, the definition of deep learning in this context is defined by its capacity to adapt and learn from complex data through structured algorithms.
Importance of Medical Imaging
Medical imaging serves as a pivotal component in contemporary healthcare. It provides critical information necessary for diagnosing diseases and monitoring treatments. Technologies such as MRI, CT scans, and X-rays are integral for visualizing the internal structures and functions of the body. The importance of medical imaging cannot be overstated; it lays the groundwork for effective clinical decision-making.
Here are a few reasons why medical imaging is vital:
- Early Detection: Medical imaging enables earlier detection of conditions like cancer, which significantly increases survival rates.
- Treatment Planning: Accurate imaging assists in planning precise treatment approaches, enhancing the Aeffectiveness of healthcare interventions.
- Monitoring Progress: Imaging facilitates ongoing monitoring of treatment efficacy, allowing adjustments to be made when necessary.
- Research and Development: Advancements in imaging technologies are crucial for medical research, leading to better understanding and treatment of diseases.
Ultimately, medical imaging serves as the visual foundation of effective and precise healthcare, making its continual evolution essential.
"The intersection of deep learning and medical imaging represents a frontier in healthcare efficiency, one that is ripe for exploration and implementation."
Through the exploration of deep learning and its integration into medical imaging, we can appreciate the depth of its impact on healthcare outcomes, especially in this age of rapid technological advancement.
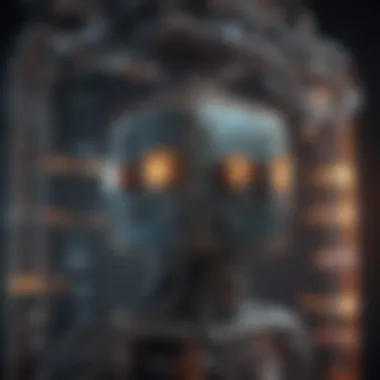
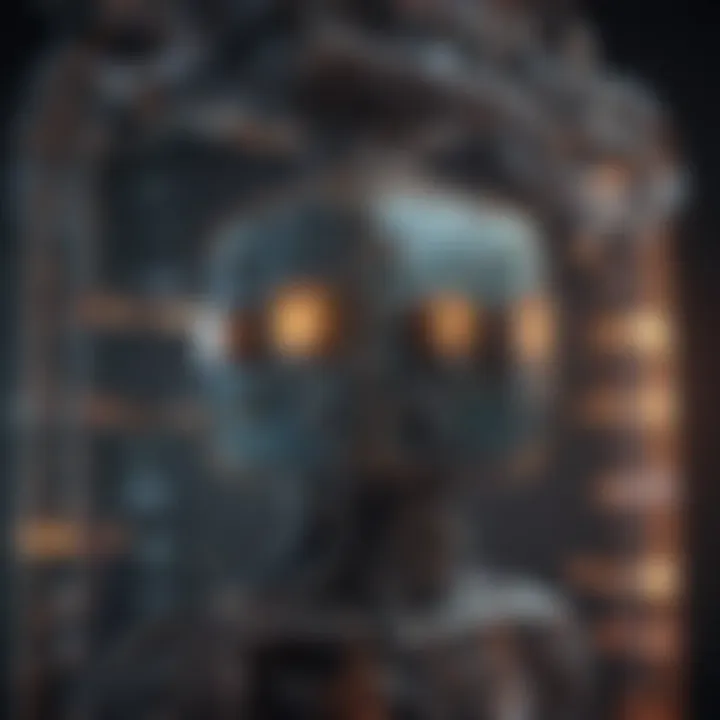
Foundations of Deep Learning
Deep learning forms the bedrock of many innovations in medical imaging today. Understanding its foundations is critical to appreciating how these technologies transform diagnostics. Deep learning relies on techniques that enable computers to learn from vast amounts of data with minimal human intervention. This area of study is dynamic and nuanced, benefitting from ongoing research and development.
Neural Networks Overview
At the heart of deep learning are neural networks. These systems mimic how human brains process information. Composed of layers of nodes, a neural network analyzes data through interconnected pathways. Each node increases the system’s ability to recognize patterns and make predictions. Neural networks excel at handling the complexity of medical images, which often contain subtle cues that are crucial for accurate interpretation.
Types of Neural Networks
The realm of neural networks is diverse, with several specific types that have unique functions in medical imaging. Key types include Convolutional Neural Networks, Recurrent Neural Networks, and Generative Adversarial Networks. Each type has distinct features making it suited for different tasks.
Convolutional Neural Networks
Convolutional Neural Networks (CNNs) are widely recognized for their strength in image processing tasks. A key characteristic of CNNs is their ability to automatically capture spatial hierarchies in data. This capability allows them to identify various features of medical images effectively, such as edges, textures, and shapes. The architecture of CNNs includes layers that systematically reduce the dimensionality of the images while preserving essential information.
The ability to learn from large datasets makes CNNs especially beneficial for detecting anomalies in medical images. They are typically preferred for tasks involving classification and segmentation in radiology.
Recurrent Neural Networks
Recurrent Neural Networks (RNNs) are another type that excels in processing sequential data, making them useful for tasks that involve a temporal dimension, such as analyzing time-series data from medical imaging. A notable feature of RNNs is their inherent memory capability, allowing them to leverage previous information in their predictions. This characteristic is particularly beneficial for longitudinal patient data, where context over time can influence diagnosis and treatment. However, they face challenges such as difficulty in learning long-term dependencies.
Generative Adversarial Networks
Generative Adversarial Networks (GANs) stand out for their unique approach in generating new data instances. Comprised of two models—a generator and a discriminator—GANs work in opposition to improve each other's performance. The generator creates new data, while the discriminator evaluates its authenticity. This dynamic leads to the production of high-quality synthetic images, which can augment training datasets. While GANs offer remarkable benefits in simulation and augmentation, there are concerns about their potential misuse and ethical implications, particularly in generating potentially misleading medical images.
In summary, a deep understanding of these foundational elements in deep learning is critical for leveraging the full potential of neural network techniques in medical imaging. Each type of neural network presents its own benefits and challenges, influencing how they may be utilized effectively in clinical settings.
Applications in Medical Imaging
The application of deep learning technologies in medical imaging is a fundamental aspect that demystifies their impact on healthcare. Medical imaging encompasses various techniques that capture visual representations of the body's interior for clinical analysis. Here, deep learning plays a crucial role. It not only enhances diagnostic capabilities but also improves efficiency and accuracy in interpreting complex datasets. By analyzing vast quantities of imaging data, deep learning technologies can aid healthcare professionals in identifying abnormalities that might be missed by the human eye.
Image Classification
Image classification involves the automatic categorization of images into predefined classes. In medical imaging, this can refer to distinguishing between benign and malignant tumors in mammograms or detecting different types of pneumonia in chest X-rays. Deep learning algorithms, particularly Convolutional Neural Networks (CNNs), have emerged as powerful tools for image classification tasks.
Training a deep learning model for image classification requires substantial datasets. The model learns to recognize patterns through numerous examples. As the model trains, it adjusts its internal parameters to minimize error in classification. The benefit of this technology in a clinical setting is significant. It allows radiologists to quickly verify initial assessments and supports clinical decision-making.
Object Detection
Object detection goes beyond classification by locating and identifying objects within images. In a medical context, this means pinpointing the exact location of tumors, fractures, or lesions in various imaging modalities like CT scans or MRI scans. Detecting these objects accurately is critical for guiding treatment decisions and surgical interventions.
Deep learning models, particularly those based on architectures like Faster R-CNN or YOLO (You Only Look Once), have revolutionized object detection precision and speed. By processing images in real-time, these models can highlight relevant areas that require further review. Furthermore, this automation decreases the workload on radiologists, enabling them to focus on more complex tasks or direct patient care.
Segmentation Techniques
Segmentation involves partitioning an image into segments that make it easier to analyze. In medical imaging, this can involve identifying the boundaries of organs, lesions, or other significant structures. Accurate segmentation facilitates precise treatment planning, such as in radiation therapy, where precise targeting is paramount.
Deep learning approaches like U-Net, a specialized architecture for biomedical image segmentation, have shown remarkable performance. These models are trained to differentiate between various regions within images, providing detailed insights into the anatomical features of the body. This leads to improved surgical outcomes and more tailored therapies.
Segmentation also opens avenues for quantitative analysis, which can further drive research and improve patient outcomes by enabling more personalized healthcare solutions.
The adoption of deep learning in applications like image classification, object detection, and segmentation signifies a transformative step in medical imaging, fostering better diagnostic capabilities and more efficient clinical workflows.
Transforming Diagnostics with Deep Learning
The integration of deep learning technologies into medical diagnostics marks a pivotal evolution in the healthcare field. These advancements offer numerous benefits that significantly enhance the precision and reliability of medical imaging. Understanding these benefits requires a examination of how deep learning impacts diagnostic accuracy and helps streamline clinical practices.
In the realm of diagnostics, deep learning stands as a powerful tool that facilitates the analysis and interpretation of complex medical images. This is especially relevant in areas like radiology, pathology, and dermatology, where waveform and pixel data can be challenging to interpret for practitioners. As deep learning algorithms process vast amounts of imaging data, they become adept at identifying patterns and anomalies that may go unnoticed by the human eye. The results are often faster and more accurate diagnostics.
Enhancing Diagnostic Accuracy
One of the key advantages of implementing deep learning models in medical diagnostics is the substantial improvement in diagnostic accuracy. These models improve precision by learning from extensive datasets, allowing for a nuanced understanding of various conditions.
- Pattern Recognition: Deep learning models can recognize intricate patterns in images more effectively than traditional methods can. By utilizing Convolutional Neural Networks (CNNs), these algorithms are designed specifically to work with visual data, enabling high levels of detection accuracy in identifying tumors, lesions, and other abnormalities.
- Continuous Learning: Deep learning systems can continually improve their diagnostic capabilities with new data. As more images are processed, the algorithms evolve, refining their performance through techniques such as transfer learning. This adaptability ensures that diagnostic tools remain relevant and effective as medical knowledge expands.
"Deep learning's proficiency in pattern recognition allows for timely and precise diagnostics, minimizing the risk of misinterpretation in critical situations."
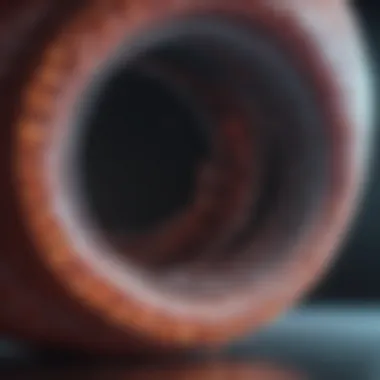
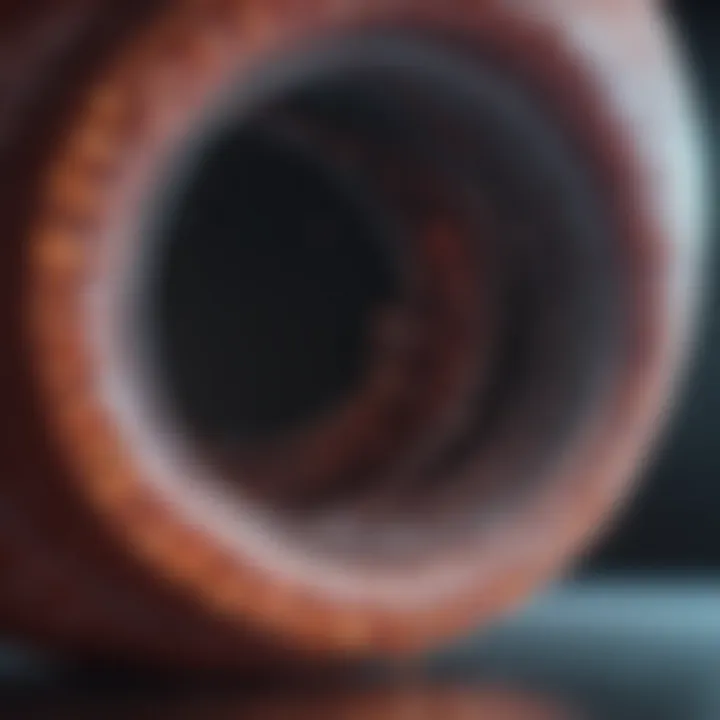
By enhancing diagnostic accuracy, deep learning also contributes to better patient outcomes. Early and precise detection of medical conditions can lead to timely interventions, which often translates into improved prognoses and reduced healthcare costs.
Reducing Human Error
Human error remains a significant concern in medical diagnostics. Fatigue, cognitive overload, and subjective interpretation can impair a radiologist's performance, potentially leading to misdiagnoses. Deep learning serves as a countermeasure by providing consistent, objective analysis of imaging data.
- Automated Assessments: With the capability to analyze images autonomously, deep learning systems can assist or even replace human interpretations in certain scenarios. This means radiologists can focus their expertise on more complex cases or patient interactions, thereby optimizing their workflow.
- Reduced Variation: Algorithms operate under defined parameters, thereby minimizing discrepancies that arise from personal interpretations. This uniformity is crucial in ensuring that diagnostic conclusions remain consistent across different practitioners and institutions.
- Error Detection: Advanced systems are not only capable of making diagnostic recommendations but can also flag images that may need further review. This dual function of detection and recommendation can significantly lower the chances of overlooking critical issues.
In summary, deep learning is transforming the landscape of medical diagnostics through enhanced accuracy and minimized human error. As these systems become integral to clinical workflows, they promise a future where diagnostics are not only faster but also more reliable, paving the way for improved patient care.
Training Deep Learning Models
Training deep learning models is a critical aspect of leveraging this technology in medical imaging. It encompasses various processes and considerations that ultimately impact the effectiveness of the models. Each step of training, from data collection to fine-tuning the algorithms, contributes significantly to improving diagnostic accuracy and optimizing patient outcomes. Without a comprehensive training strategy, models may underperform or yield inaccurate results, which can be detrimental in the medical field.
Data Collection and Annotation
Data collection is the first and foremost step in training deep learning models. It involves gathering medical images from diverse sources, such as hospitals, imaging centers, and research institutions. The quality and diversity of the dataset is crucial, as it directly influences the model's learning ability. Furthermore, images need to be meticulously annotated. This annotation process can include marking regions of interest, labeling diseases, or categorizing findings. Proper annotation ensures that the model learns from accurate and reliable information.
Mislabeling or inadequate data can lead to poor model performance. Thus, engaging experienced clinicians or radiologists in the annotation process enhances the quality of data. Additionally, data privacy and ethical considerations must also be addressed. With the increasing emphasis on patient confidentiality, combining data acquisition practices with adherence to regulations is essential.
Model Training Processes
Model training processes are varied and include distinct strategies that can enhance the performance of deep learning applications. Three notable methods are transfer learning, fine-tuning, and hyperparameter optimization.
Transfer Learning
Transfer learning leverages previously trained models to streamline the training of new models for specific tasks. This is particularly valuable in medical imaging, where available labeled data might be limited. The key characteristic of transfer learning is that it allows models to utilize patterns learned from vast datasets. Hereby, it accelerates the training process while improving accuracy.
One unique feature of transfer learning is the ability to adapt a general model to more niche areas with relatively little data. Nevertheless, it can have limitations, such as inheriting biases present in the original model, which can affect performance in specialized medical tasks. Ultimately, its advantages often outweigh the downsides in many practical applications.
Fine-Tuning
Fine-tuning is a subsequent step that involves making small adjustments to a pre-trained model to better align it with the specific medical imaging task. This process refines the model's weights to improve its predictions on the target dataset.
A primary feature of fine-tuning is its flexibility, allowing models to adapt to new data with relative ease. It often leads to faster convergence and enhances the overall predictive accuracy. However, there is a need for careful monitoring during fine-tuning. Overfitting can occur if a model is trained too long on a narrow dataset. The balance between training duration and model versatility is key.
Hyperparameter Optimization
Hyperparameter optimization focuses on identifying the best settings for the training model, such as learning rate, batch size, and regularization parameters. This meticulous adjustment is vital as it significantly affects the model's learning process and final performance.
The critical feature of hyperparameter optimization is its potential to improve results without needing additional data. Techniques such as grid search or Bayesian optimization can be utilized to speed up this process. However, this method can be computationally intensive, requiring substantial resources and time. The trade-off between resource investment and the resultant model performance needs careful evaluation.
In summary, the training of deep learning models in medical imaging is a multi-faceted endeavor. It requires careful attention to data collection and annotation, as well as employing suitable training processes like transfer learning, fine-tuning, and hyperparameter optimization. As practitioners in this field become more adept at these processes, there is significant potential for improved diagnostic outcomes and enhanced patient care.
Challenges in Implementation
Implementing deep learning in medical imaging presents several key challenges that can impact the effectiveness and acceptance of these technologies. Addressing these challenges is essential for fully realizing the benefits of deep learning in healthcare. Understanding the factors that impede successful implementation can guide future developments, ensuring that deep learning solutions are both effective and ethically sound.
Data Privacy Concerns
Data privacy is a primary concern when incorporating deep learning into medical imaging. Medical data is sensitive and must be handled with care. There is a constant risk of unauthorized access to patient information, which can lead to breaches of privacy. With large datasets being required for deep learning training, protecting patient identities becomes complex. Techniques like anonymization and encryption are necessary to safeguard data, but these methods sometimes hinder the ability to use the data effectively.
Furthermore, the regulations such as GDPR in Europe and HIPAA in the United States impose strict guidelines on data use. Compliance with these rules is non-negotiable, necessitating that practitioners and technologists have a thorough understanding of legal obligations. Organizations need robust data governance frameworks to manage and maintain data privacy while harnessing deep learning's potential.
Algorithmic Bias
Another significant challenge is algorithmic bias, which arises when the data used to train neural networks is not representative of the diverse patient population. Bias can lead to misdiagnoses and inadequate treatment options for underrepresented groups. For example, if a model is trained predominantly on images from a certain demographic, it may perform poorly on patients from different backgrounds, which can exacerbate health inequalities.
Addressing bias involves careful selection of training data and continuous monitoring of model performance across various demographics. It is essential to employ strategies that enhance the dataset's diversity to create more inclusive algorithms. Regular audits and testing of deep learning models can help identify and mitigate bias, fostering a more equitable healthcare system.
Integration into Clinical Workflows
Integrating deep learning tools into clinical workflows is critical for their successful application. Many healthcare settings operate with established protocols and systems that may not easily accommodate new technologies. Resistance from staff or the need for additional training can slow down the adoption process. Thus, smooth integration requires considering how these tools will fit into daily practices.
Additionally, interoperability between deep learning solutions and existing medical imaging systems is crucial. Considerations need to be made on how to seamlessly exchange data without disrupting workflows. Developing user-friendly interfaces that allow healthcare providers to interact with deep learning systems without significant additional burden can enhance acceptance.
"Successful implementation of deep learning in medical imaging requires not only technical solutions but also a thorough understanding of the healthcare environment in which it operates."
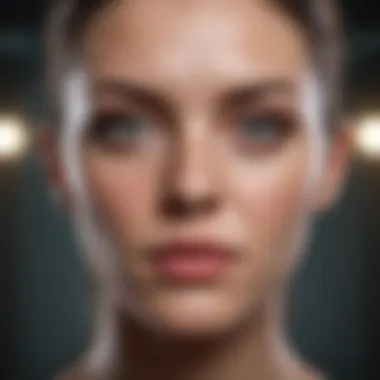
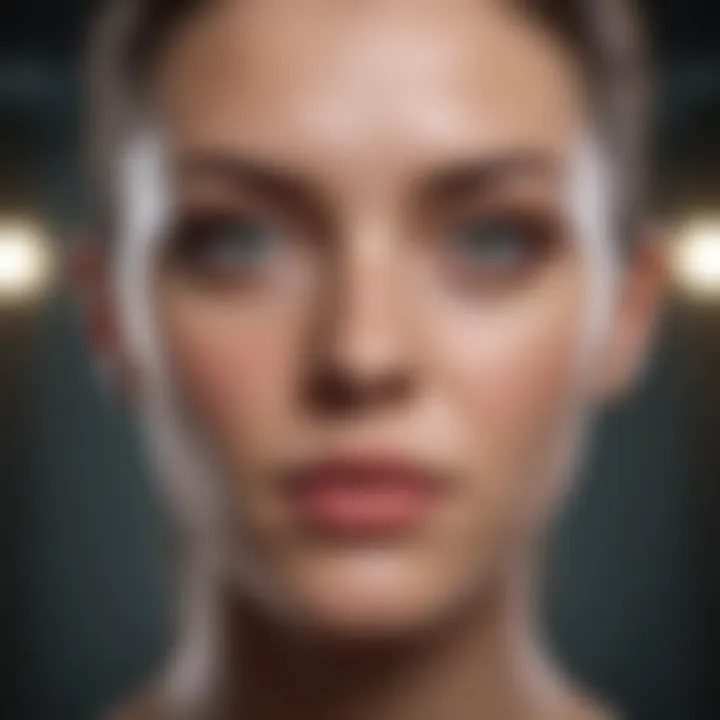
In summary, addressing data privacy, algorithmic bias, and integration into clinical workflows are crucial steps for implementing deep learning in medical imaging. Overcoming these challenges can help maximize the advantages that deep learning offers to the healthcare field.
Ethical Implications
The integration of deep learning into medical imaging presents several ethical considerations that merit serious attention. As these technologies evolve, so too does the need for appropriate ethical frameworks that can address the various dilemmas arising from their use in clinical settings. Ethical implications are essential, not only for compliance with regulations but also for maintaining public trust in healthcare systems that rely on sophisticated algorithms for patient diagnosis and treatment. Understanding these ethical dimensions is crucial for shaping policies that foster responsible use of these technologies.
Informed Consent and Data Use
Informed consent is a cornerstone of ethical medical practice. When implementing deep learning technologies, healthcare professionals must ensure that patients are adequately informed about how their data will be used. This includes explaining how medical images and other personal health information may be collected, analyzed, and potentially shared with third parties. Patients should understand the benefits and risks associated with their data use in training deep learning algorithms. Moreover, the dynamic nature of these technologies raises questions about maintaining consent over time, particularly as models evolve.
Key aspects of informed consent include:
- Clarity: Patients must receive clear and concise information about the processes involved. Legal jargon must be avoided to enhance understanding.
- Autonomy: It is essential that individuals feel empowered to make decisions regarding their health data without coercion.
- Revocation Rights: Patients should also be informed of their right to withdraw consent and how they can do it at any time.
Addressing these factors effectively can promote a more ethical landscape in medical imaging through deep learning.
Transparency in Algorithms
Algorithmic transparency is vital for fostering trust in healthcare applications of deep learning. As these algorithms often operate as "black boxes," understanding how they make decisions is crucial for both healthcare professionals and patients. Transparency aids in elucidating the decision-making process, ensuring clinicians can interpret the outcomes of algorithmic assessments accurately.
Important considerations for algorithm transparency include:
- Methodology Disclosure: Clinics should openly share the methodologies used in developing and refining deep learning models.
- Performance Metrics: Providing insights into the performance of algorithms, including accuracy, sensitivity, and specificity, can help stakeholders gauge reliability.
- Addressing Bias: Algorithms must be scrutinized for biases that can lead to disparities in diagnosis and treatment, particularly for underrepresented groups.
Transparency not only assists in ethical accountability but also enables continuous improvement of deep learning systems, as feedback from medical professionals and patients can guide future developments.
Future Directions
The landscape of deep learning in medical imaging is rapidly evolving. Future directions play a pivotal role in shaping the trajectory of technology and its integration into health care systems. This section discusses the advancements in model architectures and the impact of federated learning, both vital elements for enhancing medical imaging applications.
Advancements in Model Architecture
Advancements in model architecture are essential for improving the efficiency and accuracy of deep learning applications in medical imaging. Innovative architectures, such as EfficientNet and DenseNet, represent significant strides in maximizing performance while minimizing computational costs. These models benefit from features like multi-scale feature extraction and optimized parameter usage.
Key benefits of these advancements include:
- Improved Accuracy: More sophisticated architectures lead to better feature extraction and higher diagnostic accuracy.
- Reduced Overfitting: New designs help mitigate overfitting, which is crucial in clinical settings where generalizability is paramount.
- Faster Training: Advanced models often require fewer training epochs, thus speeding up the development lifecycle of medical imaging AI systems.
As research continues, a focus on architecturally diverse frameworks that can adapt to various imaging modalities, such as MRI and CT scans, will be important. This adaptability enhances the applicability of deep learning across different areas of medical imaging, thus broadening its reach and effectiveness.
Impact of Federated Learning
Federated learning represents a transformative shift in how machine learning models are trained and deployed, especially in sensitive fields like healthcare. This distributed approach allows multiple institutions to collaborate without sharing raw patient data, which directly addresses privacy concerns.
Key considerations regarding federated learning include:
- Data Privacy: Patient data remains local to the institution, enhancing compliance with regulations such as HIPAA.
- Decentralization: Institutions can contribute to model training while maintaining control over their data. This leads to a richer dataset for training without data centralization risks.
- Continuous Learning: Federated learning facilitates ongoing model updates and improvements across sites, reflecting diverse patient populations and conditions.
"Federated learning not only preserves patient confidentiality but also promotes collaborative research across healthcare institutions."
The integration of federated learning into deep learning workflows will be a game changer, enabling more robust and versatile models that are better suited to the dynamic nature of medical diagnostics.
Ending
The conclusion of this article encapsulates the journey through deep learning's impact on medical imaging. Importantly, it reinforces the significance of these advances in diagnostic accuracy, highlighting a major shift in how healthcare is approached. Technologies like convolutional neural networks are not mere trends; they represent a fundamental change in the ability of practitioners to interpret complex medical images with precision.
Notably, the synthesis of insights drawn throughout the article demonstrates how deep learning vastly improves image analysis and enhances clinical decision-making. This can lead to more accurate diagnoses, timely interventions, and ultimately better patient outcomes. As we navigate this complex landscape, considerations must also be given to the ethical implications and challenges presented.
Deep learning does not function in isolation. The broader healthcare ecosystem must adapt to leverage these innovations while ensuring patient privacy and addressing the risks of algorithmic bias. The call for collaboration among technologists, clinicians, and ethicists is paramount.
Furthermore, the necessity for ongoing research cannot be overstated. Innovations in deep learning will keep evolving, and continual adaptation to new findings, methods, and technologies is essential. In this context, the conclusion serves as both a summary and a call to action for stakeholders across the medical field.
Summary of Key Insights
This article has explored several critical dimensions of deep learning in medical imaging. Key insights include:
- Deep learning frameworks offer superior capabilities in image classification and segmentation, which are vital for accurate diagnostics.
- Recent advancements in model architecture and training techniques, such as transfer learning, have made it easier to train models with less data, addressing a significant barrier in medical applications.
- Ethical considerations are critical in integrating AI into healthcare practices; transparency and informed consent must be prioritized.
- Challenges associated with data privacy and integration into clinical workflows must be navigated carefully.
These points underscore the importance of deep learning as a tool not just for efficiency but for enhancing the quality of care delivered to patients.
Call for Continuous Research and Development
The landscape of deep learning in medical imaging is one of rapid evolution. Hence, continuous research and development are crucial. The following aspects merit attention:
- Innovations in AI Algorithms: Frequent updates and improvements in algorithms will lead to better accuracy and reliability.
- Expanding Data Sets: Collecting diverse datasets will help to develop more robust models, reducing biases and improving generalizability.
- Collaboration Across Disciplines: Bridging the gap between computer science, medical professionals, and ethicists will foster responsible innovation.
- Regulatory Oversight: Continued dialogue with regulators will help ensure that safeguards are in place to protect patients while encouraging innovation.