Finding Reliable Datasets for Research Purposes
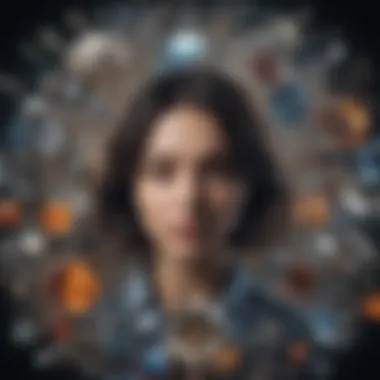
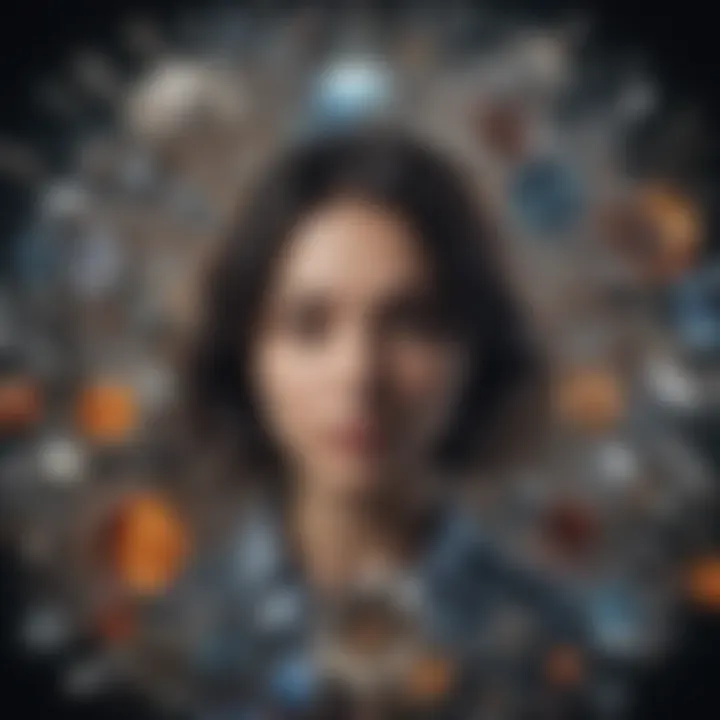
Intro
In today’s fast-paced research environment, the significance of high-quality datasets cannot be overstated. Researchers, educators, and students alike often find themselves on a quest for reliable data that can drive insightful discoveries and validate assumptions. The journey for quality data might seem overwhelming with the vast ocean of available sources. From established repositories to burgeoning platforms sprouting in various scientific fields, knowing where to look is key.
As we dive into this guide, our focal point is not just the ‘where’ but also the ‘how’ and ‘why’ associated with locating datasets that meet rigorous standards of quality and relevance. Striking the right balance between reliability and accessibility is essential. Whether you’re a seasoned investigator or a newcomer to the world of research, understanding the avenues available for obtaining datasets is pivotal.
In this exploration, we will also take a look at the organized approaches and critical thinking strategies that can assist in pinpointing the datasets that suit your study's needs.
Research Overview
The pursuit of high-quality datasets emerges as a fundamental aspect of any research endeavor. Academic integrity hinges on the data's validity, and errant datasets can lead to flawed conclusions. The focus here is on summarizing key findings that elucidate the components of high-quality data.
Summary of Key Findings
When evaluating datasets, various factors come into play:
- Source Credibility: Validity often emerges from reputable sources. Established institutions typically yield more trustworthy datasets.
- Metadata Quality: Well-documented datasets provide the context, facilitating better understanding and analysis.
- Accessibility and Usability: Datasets that are user-friendly and easy to obtain are more likely to be utilized effectively.
- Relevance: Ensuring the dataset aligns with the research question is crucial for producing meaningful outcomes.
Importance of Research in Its Respective Field
In fields like epidemiology, economics, and even machine learning, quality data serves as the backbone of robust findings. High-quality datasets allow researchers to explore complex questions with greater assurance, leading to sound conclusions that can serve the wider community's interest. As more folks delve into data science and analytics, the emphasis on locating and leveraging high-quality datasets becomes increasingly significant.
"The quality of data determines the quality of decisions, and ultimately, the quality of life."
Methodology
Description of Experimental or Analytical Methods Used
Researchers conduct extensive reviews and comparative analyses of available datasets. Approaches such as systematic reviews can help in identifying the breadth and depth of datasets available across different domains. Techniques include:
- Scoping Reviews: To outline the existing literature and datasets.
- Meta-Analysis: To pool data from various studies to uncover trends.
- Network Analysis: To visualize connections between datasets and their applications.
Sampling Criteria and Data Collection Techniques
When therapists pick datasets, they consider:
- Temporal Relevance: How current the data is, given that outdated data can skew findings.
- Geographic Relevance: Datasets must correspond to the targeted demographic areas of research.
- Sample Size: Larger datasets tend to provide more reliable insights but also require thorough quality checks to discern accuracy.
Ultimately, a strategic approach to data collection not only facilitates effective research but also underscores the importance of quality and validity in achieving worthwhile educational outcomes.
Identifying the Need for Datasets
In a world overflowing with information, pinpointing the right dataset can feel akin to searching for a needle in a haystack. Before diving into the vast oceans of data available, it’s crucial to take a step back and recognize why datasets matter. Identifying the need for datasets isn't just an academic exercise; it’s a foundational step that can shape the trajectory of research. Without a well-defined requirement, any datasets scavenged may not only lack relevance but might lead to misguided conclusions, which, let’s face it, is the last thing any researcher wants.
Understanding Dataset Requirements
First and foremost, knowing what you need from a dataset can save you time and energy. It’s essential to delineate specific criteria that your expected dataset must meet. Consider aspects like:
- Type of Data: Are you looking for qualitative data, quantitative data, or perhaps both? Understanding the nature of the data (e.g., numerical, textual, spatial) is key.
- Scope and Scale: What’s the size of the dataset you envision? Large datasets can pack a punch for big data analysis, but smaller datasets might be more relevant for niche studies.
- Time Frame: Depending on your research, you might need historical data, recent data, or even real-time data. Clarifying timeline requirements can refine your search significantly.
- Geographical Focus: Is your research centered in a specific location? If so, datasets localized to that area will yield insights tailored to your context.
Perhaps most importantly, it’s wise to ask yourself how this dataset aligns with your broader research goals. If you can’t articulate that fit, it's a sign you may need to rethink your approach.
Setting Research Objectives
Once you have a grasp on your dataset requirements, the next logical step involves clearly setting your research objectives. These objectives act as your North Star, guiding your exploration of datasets and ensuring you're in the right direction. Start by outlining key questions you intend to answer:
- What Hypothesis Are You Testing? Understanding this provides clarity on needed data attributes.
- Which Variables Are Crucial? Identify the factors that will drive your analysis and dictate what you'll need from a dataset.
- What Insights Are You Aiming For? Envisage the kind of conclusions your research could generate and what datasets could bridge that gap.
Establishing well-defined objectives not only sharpens the focus of your dataset search but also helps streamline data analysis later on. These objectives should be revisited throughout your research journey to remain aligned with your initial vision.
Identifying the need for datasets, understanding requirements, and setting crystal-clear objectives form the bedrock of a successful research endeavor. As we delve deeper into various sources, these preliminary steps will serve well in navigating the data landscape with more confidence.
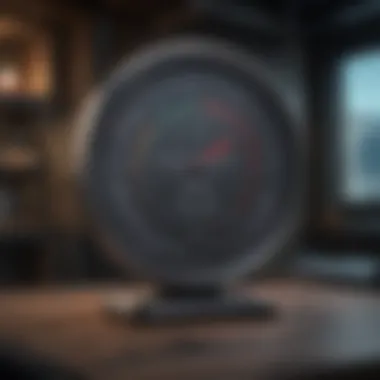
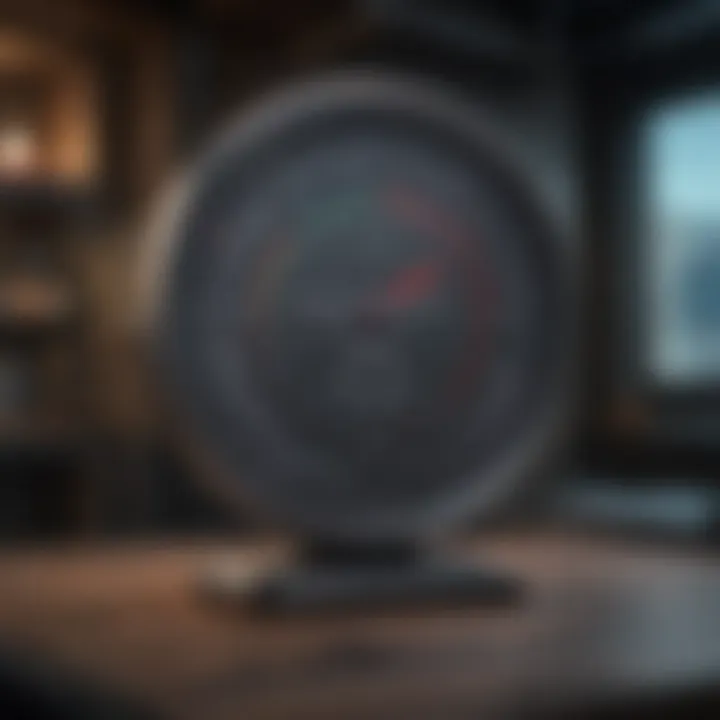
Centralized Data Repositories
Centralized data repositories play a pivotal role in the landscape of data acquisition, especially for academic and professional researchers. These repositories act as hubs, gathering and curating datasets from various sources, which streamlines the search and access process for users. The significance of these repositories cannot be overstated, as they provide a consolidated view of high-quality data across multiple disciplines.
Benefits of Centralized Repositories:
- Accessibility: Centralized repositories typically offer user-friendly interfaces and robust search functions, making it easier for users to find relevant datasets quickly without the hassle of sifting through scattered sources.
- Quality Assurance: Many of these repositories impose strict guidelines for data inclusion, often ensuring that the datasets are rigorously vetted for quality and reliability.
- Diversity of Data: They encompass a wide range of datasets, be it concerning demographics, health, environmental concerns, or more specialized fields like bioinformatics.
- Interdisciplinary Collaboration: Centralized repositories foster a collaborative environment, encouraging researchers from different fields to share insights and findings based on the same datasets, driving innovation and discovery.
Nevertheless, one must consider certain aspects when engaging with centralized repositories. The primary concern often revolves around data licensing and access rights, as not all data is freely available. Furthermore, navigating through these vast repositories can sometimes be overwhelming due to the sheer volume of available datasets.
Overall, centralized data repositories provide indispensable resources for researchers, combining convenience and quality in data access.
University Libraries and Archives
University libraries and archives hold a treasure trove of data that can support a variety of research needs. These institutions often curate both physical and digital datasets collected from past research, dissertations, and ongoing projects. Researchers should not overlook the fact that many universities have dedicated staff or departments focused on managing and providing access to datasets.
For academic users, leveraging university repositories also means you are tapping into resources that align well with your field of study, often with local knowledge that can be extremely beneficial. Beyond just databases, many university archives contain unique datasets not found anywhere else, often tied to specific local or regional projects.
Government Databases
Government databases represent another critical segment in the realm of centralized data repositories. They provide comprehensive datasets that range from economic statistics to public health records, thus forming a backbone for research in various disciplines.
National Aeronautics and Space Administration (NASA)
The National Aeronautics and Space Administration creates and maintains extensive datasets related to space exploration, Earth science, and aeronautics. One of NASA's key strengths is the rich array of datasets available through platforms such as the NASA Earth Data portal. This casual accessibility makes it an attractive option for students and researchers interested in environmental science, climate change, and related fields.
The unique feature of NASA's datasets is their open distribution, encouraging widespread use. However, while the volume and depth of data are significant advantages, newcomers might find the specialized terminology and complexities of some datasets daunting.
Centers for Disease Control and Prevention ()
The CDC stands as a leading authority on public health, providing datasets that are crucial for understanding health trends, disease outbreaks, and epidemiological studies. The beauty of LLC's datasets lies in their comprehensive nature and relevance to global health issues.
With datasets covering a wide range of health-related topics, from vaccination rates to chronic disease statistics, CDC's resources are popular among researchers in the health sciences. While the integrity and accuracy of the data are commendable, users must sometimes navigate specific guidelines for accessing sensitive health information, adding another layer of complexity to its use.
International Organizations
International organizations like the World Health Organization (WHO) and the World Bank Data repository also serve as centralized data repositories, offering datasets that reflect global health trends and economic indicators, respectively.
World Health Organization (WHO)
The WHO labors tirelessly to provide datasets that encapsulate global health metrics, such as disease prevalence and health systems performance. The WHO's extensive network and commitment to data transparency make it a valuable resource for health researchers. Highlighting health data across different countries allows for comparative studies that are crucial for policy development and program evaluation.
Nevertheless, while WHO datasets are thorough and impactful, the process to navigate through their official channels can often be lengthy, and specific data may not always be straightforward to obtain.
World Bank Data
World Bank Data is known for its rich compilation of global economic data, development indicators, and poverty statistics. The key characteristic of this repository is its commitment to promoting current and historical data that guide development policies. Its user-friendly interface and extensive dataset categories make it a favored choice for economists and social scientists alike.
However, while it provides an extensive collection of data, some datasets may require careful interpretation due to the context in which they were collected—this can sometimes lead to misinterpretations if not thoroughly understood.
In summary, centralized data repositories provide a critical base for researchers seeking high-quality datasets. The accessibility and rigor of these repositories ease the path toward meaningful research while keeping quality considerations in focus.
Specialized Data Platforms
Specialized data platforms are becoming increasingly pivotal in the landscape of research, particularly for the pursuit of high-quality datasets. These platforms often cater to specific fields, offering tailored resources that can save researchers considerable time as they search for relevant information. With a myriad of unique datasets available through these specialized avenues, navigating these platforms can enhance the depth of research significantly.
Utilizing these specialized data platforms not only provides access to vast collections of data but also offers contextual understanding that can be critical for validating findings. By focusing on data specific to the discipline at hand, these resources allow scholars to extract insights that might otherwise remain buried under piles of irrelevant information. Furthermore, the quality of the datasets curated by these platforms is often superior, given that they usually undergo rigorous scrutiny to meet the established standards of the respective fields.
Life Sciences Databases
Gene Expression Omnibus (GEO)
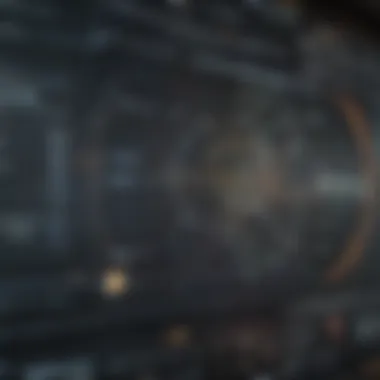
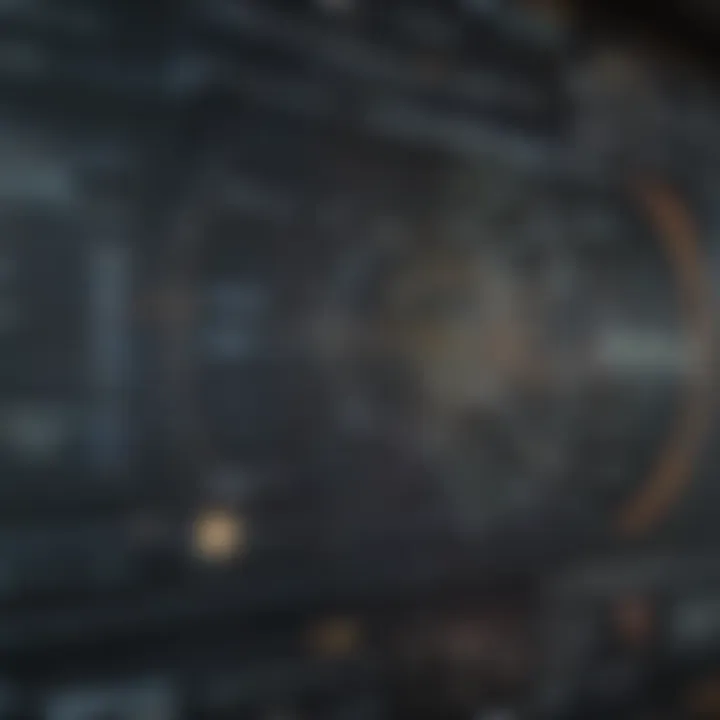
The Gene Expression Omnibus (GEO) excels as a repository for gene expression data, crucial for those in the life sciences realm, especially disciplines like genomics and molecular biology. This platform primarily specializes in storing curated datasets from transcriptomic studies and is instrumental in allowing researchers to access large-scale gene expression datasets.
A key characteristic of GEO is its comprehensive database structure which enables users to search for datasets based on a variety of parameters, such as organism, condition, or experimental design. This feature makes GEO a beneficial choice for many researchers. One unique aspect of GEO is its ability to provide tools for data analysis, allowing scientists to visualize and interpret data trends effectively. However, it’s important to note that while GEO provides a rich repository, the sheer volume of data can be overwhelming for some, requiring users to be acclimated to bioinformatics practices to maximize the platform's offerings.
Protein Data Bank (PDB)
The Protein Data Bank (PDB) serves as a global archive for three-dimensional structural data of biological macromolecules. For researchers focusing on structural biology or bioinformatics, PDB is an invaluable resource. Its contribution to the field cannot be overstated, providing essential data for understanding the complex interactions within biological systems.
One of PDB's core strengths lies in its emphasis on the structure-function relationships of proteins. This wealth of information is particularly advantageous for those developing therapeutics or studying mechanisms of action. A standout feature of PDB is the integration with visualization tools that allow users to interactively explore molecular structures. While this platform is widely regarded for its high-quality datasets, it also poses challenges, such as the high level of expertise required to interpret the structural data meaningfully.
Social Science Data Libraries
Inter-university Consortium for Political and Social Research (ICPSR)
The Inter-university Consortium for Political and Social Research (ICPSR) is an extensive resource for social scientists. It acts as a repository for research data across a wide array of social science disciplines, including political science, sociology, and education. Its contribution to the scholarly community is significant, providing data that is essential for empirical research.
What makes ICPSR a standout is its commitment to preserving social science data and making it available for secondary analysis, which is vital for researchers looking to build on existing studies. The platform also offers tools that assist users in understanding and refining their datasets. However, accessing certain datasets may require affiliation with member institutions, which could be a limitation for independent researchers.
Social Science Research Network (SSRN)
The Social Science Research Network (SSRN) is a hub for researchers to share their drafts, preprints, and working papers in the fields of social sciences. This platform champions open access, allowing scholars from around the globe to disseminate their findings quickly and efficiently.
A key attribute of SSRN is its global reach, bridging researchers from different backgrounds and facilitating collaborations across borders. The platform encourages early-stage research dissemination, making it a popular choice for scholars eager to share their work. A unique feature of SSRN is its metrics system, allowing authors to track engagements with their work. However, it should be noted that the open nature of SSRN means that the quality of papers can vary significantly, and it’s up to the reader to critically assess the research's validity.
In navigating these specialized data platforms, researchers must remain cognizant of their specific needs and the context of their studies, which will guide the selection of the most relevant data repositories.
Open Data Initiatives
Open data initiatives are reshaping the landscape of data accessibility. They encourage a culture of transparency and collaboration, allowing researchers, students, and interested individuals to access datasets for various purposes. These initiatives significantly enhance the research environment by lowering barriers to entry, thus promoting innovation and informed decision-making.
One notable aspect of open data initiatives is their contribution to the democratization of knowledge. When datasets are publicly available, individuals can analyze information on their own terms without having to navigate complex licensing agreements or pay hefty fees. This accessibility can lead to unexpected insights, as amateur analysts or smaller organizations may approach data from fresh perspectives, offering new interpretations that specialists might overlook.
However, it's crucial to recognize that while open access fosters inclusivity, it also comes with certain challenges. The variability in data quality can be a concern; not all datasets follow the same stringent collection and quality control processes as paid or proprietary datasets. Users must cast a critical eye over the available options, discerning which datasets deserve their attention and trust.
The impact of open data initiatives extends beyond academia and research.
- Economic Growth: Businesses can harness public data to drive innovation.
- Civic Engagement: Citizen data projects have emerged, helping communities analyze local issues.
- Education: Students can access vital datasets to enhance their projects and learning experiences.
In essence, open data initiatives are important not just for their available datasets, but for fostering a community of data literacy and engagement across varied sectors.
Exploring Open Access Datasets
The world of open access datasets is large and diverse. Numerous platforms exist where anyone can find and utilize valuable data. Exploring these options requires some navigational skills but can lead you to gold mines of information.
Some prominent sources include:
- Kaggle: Renowned for competitions but equally valuable for its myriad of available datasets in various domains.
- Data.gov: This U.S. government site offers access to a vast range of datasets, from environmental data to economic indicators.
- Open Data Portal: Various countries and cities have their own portals where local data can be accessed.
Delving into these resources opens doors to collaborative projects or personal research. Users should remember to review the accompanying documentation, as it often includes context that elucidates the dataset’s purpose, scope, and limitations, making the data much more usable.
The Role of Data Sharing in Science
Data sharing is the lifeline of the scientific community. It serves not only to validate research but also to inspire further discoveries. When researchers share their datasets, they promote a culture where knowledge is built collaboratively, potentially accelerating the pace of innovation.
Data sharing has several key benefits:
- Validation of Results: Other researchers can reproduce or challenge findings, ensuring robust science.
- Interdisciplinary Collaboration: Access to diverse datasets spurs collaboration across fields, leading to comprehensive insights.
- Informed Policy-Making: Policymakers utilize shared datasets to inform decisions, leading to impactful changes in society.
However, it's not all smooth sailing. Researchers often face hurdles like bureaucracy, fear of losing credit, or concern about data privacy. Thus, frameworks that support equitable sharing, like collaborative agreements and community standards, are vital for promoting an effective sharing culture.
As such, fostering open data initiatives and encouraging data sharing can create a thriving environment for scientific advancement today and into the future.
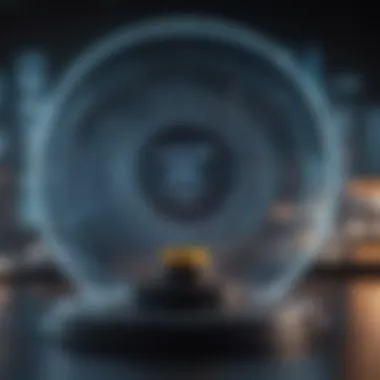
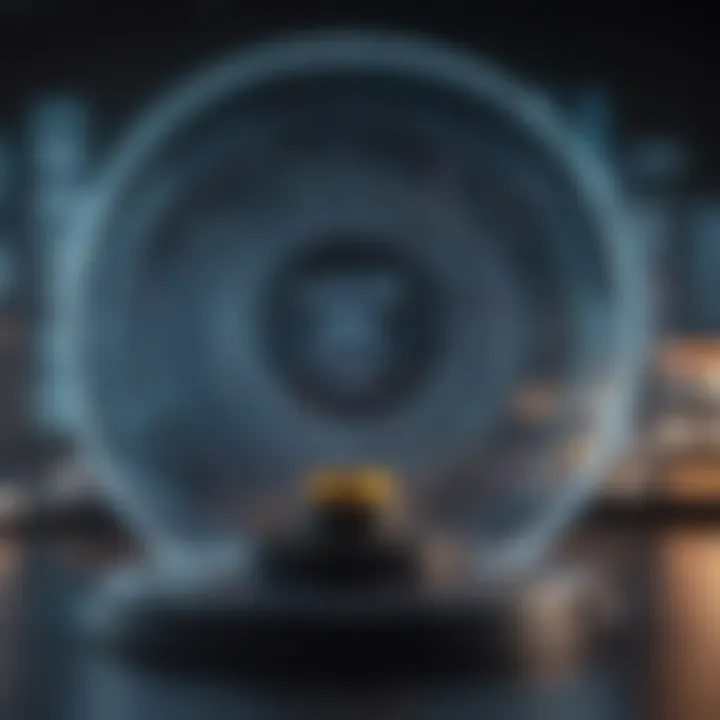
By prioritizing openness, the scientific community cultivates a culture where collaboration leads to new knowledge.
Community and Collaborative Platforms
In the quest for high-quality datasets, community and collaborative platforms offer a treasure trove of resources that can be invaluable. These spaces foster relationships among individuals and organizations, enabling shared knowledge and pooled efforts in data creation, curation, and usage. Unlike traditional data sources, community-driven platforms often provide unique datasets that might not be available elsewhere. This grassroots angle encourages innovation and adaptability, allowing researchers and thrill-seekers alike to capitalize on the collaborative spirit of the internet.
One major element of community platforms is the focus on collective contributions. When individuals from diverse backgrounds come together, they bring varying skills and insights. This synergy leads to datasets that cover a broader range of topics than any one organization might produce alone. In many cases, these platforms facilitate ongoing projects that welcome input from users, exemplifying the notion that many hands make light work.
Collaborative Data Projects
Collaborative data projects stand as the backbone of many community platforms. These initiatives not only seek to gather data but also strive to involve active participation from researchers, students, and even the general public. The advantage here lies in the openness; participants can learn from each other while contributing their unique perspectives. This can greatly enhance both the quality and relevance of the collected data.
For instance, consider the OpenStreetMap project, which relies on users to help map the world using readily available tools. Here, anyone can join in, making contributions that improve the map's overall quality. This kind of collaboration can yield datasets that are incredibly detailed and current, making them timely and relevant for various applications.
"The strength of collaborative data projects lies in their ability to adapt to evolving needs, tapping into the wisdom of crowds to produce a data pool that is both rich and robust."
Collaborative projects can also help in maintaining an ethical stance in data gathering and usage. Participants often adhere to principles of transparency and accountability, ensuring that the data is collected and shared responsibly. In today's world, where data privacy is a significant concern, fostering an ethical framework in data collection is more important than ever.
Crowdsourced Data Platforms
Crowdsourced data platforms take the concept of collaboration a step further by actively soliciting contributions from the public. Unlike traditional databases, which may rely on a more contained group of experts, these platforms rely on collective input regardless of a contributor’s background.
Imagine platforms like Kaggle, which hosts competitions for data scientists to analyze large datasets and provide insights. Users from all over the globe can participate, sharing their findings and analysis. Such environments not only produce valuable datasets but also create learning opportunities where participants can gain expertise through hands-on experience.
Additionally, crowdsourced platforms often lead to the discovery of new insights that established datasets might miss. This happens because diverse participants can approach problems from angles that a homogeneous group may overlook. As a result, users may stumble upon trends, correlations, or anomalies that add significant value to the dataset.
Yet, while the benefits of these platforms are abundant, it is also critical to approach crowdsourced data with a discerning eye. Since anyone can contribute, data integrity can sometimes be an issue. Therefore, evaluation methods and checks should be in place to ensure that the datasets gathered are not just diverse but also reliable.
Navigating the Data Landscape
In today's digital age, navigating the data landscape is more crucial than ever for anyone diving into research. As datasets proliferate, understanding the environment in which they exist becomes fundamental to ensuring quality, relevance, and ethical use. This section will discuss how to approach data acquisition while maintaining a clear focus on key considerations that underpin effective research practices.
Assessing Data Quality
When it comes to utilizing datasets, the quality of that data cannot be overlooked. Quality assurance is king; without it, your research findings could resemble a house of cards—briefly standing until a gust of wind, or in this case, skepticism, knocks it down. Here are a few pointers to consider:
- Source Credibility: Always check where the data is coming from. Well-established institutions, like universities or recognized organizations, tend to publish datasets that are subject to rigorous review processes.
- Data Completeness: Look for datasets that offer comprehensive information. Missing values can skew results, leading to incorrect conclusions. A dataset looking appealing at first glance might hide significant gaps!
- Timeliness and Relevance: Outdated data can lead you astray. Ensure the dataset is current and contextually matches your research. For instance, the economic data from last decade may paint a very different picture compared to the current economic landscape.
- Anonymization Standards: This is particularly critical when dealing with sensitive data. Assess whether the dataset complies with established protocols that safeguard personal information; this impacts not just privacy, but also the ethical standing of your research.
Using established standards and checklists can help streamline the assessment of data quality, making it easier to filter out the imperfect ones during your searches.
Understanding Licensing and Access Options
Data accessibility comes with its own set of complexities that can sometimes feel like navigating a minefield. Grasping licensing agreements and access options is vital for utilizing datasets effectively and legally, without stepping on any toes. Consider the following aspects:
- Open vs. Restricted Access: Some datasets are freely available to the public, while others come with restrictions. Clearly understanding these nuances can save you a world of trouble. This includes recognizing whether the data has usage fees and any constraints on how it can be used, especially in commercial avenues.
- Creative Commons Licenses: Many datasets use Creative Commons licensing, which often allows for remixing and using the data, provided you give appropriate credit. Familiarize yourself with the licensing types, as they dictate how you can engage with the data.
- Attribution Requirements: Knowing how to properly credit the data source is essential. Misattributing data can lead to both academic repercussions and ethical dilemmas. Always read the fine print.
- Embargoes and Expiry Dates: Some datasets may be under embargo for a duration. It’s prudent to check whether you are allowed to use the data and when it can be publicly shared. This is especially the case for datasets that contain sensitive information or are still under study.
"Data is a precious thing and will last longer than the systems themselves." – Tim Berners-Lee
Embracing these considerations will not only enhance your research outcomes but also ensure you're walking the straight and narrow. Understanding the ins and outs of navigating the data landscape can empower you to make informed choices that impact your work positively.
Future Trends in Dataset Accessibility
Understanding how datasets are made available is crucial, especially in an age where information is king. The evolution of dataset accessibility is influenced by various factors such as technological advancements and societal needs. These trends not only shape how researchers, students, and professionals access data but also inform the approaches that institutions and organizations adopt in data curation and distribution. As we delve into the future, we need to consider two main elements: the role of technology in data management and the implications of emerging data privacy regulations.
Emerging Technologies in Data Management
With the advent of machine learning and artificial intelligence, data management is taking a transformative turn. These technologies enable more efficient methods for storing, processing, and analyzing massive datasets. Here’s how emerging technologies are set to reshape the landscape of dataset accessibility:
- Cloud Computing: The shift towards cloud-based systems allows for real-time access to datasets from anywhere in the world. This is particularly beneficial for collaborative projects where multiple users need simultaneous access.
- Blockchain Technology: This decentralized method ensures data integrity and authenticity, offering a secure means of sharing datasets. Researchers can verify the origin and maintain the safety of their data.
- Automated Data Curation: Algorithms that can sort and prioritize datasets save time and effort in locating high-quality data. This automation can lead to more efficient research processes.
These advancements promise to create a seamless experience in accessing datasets while promoting transparency and reliability. Institutions that embrace such technologies can expect to better cater to the growing demands for quick and easy data retrieval.
The Impact of Data Privacy Regulations
As dataset accessibility increases, so does the necessity for robust data privacy regulations. Understanding and adhering to these regulations is paramount. Here are some considerations on how these legal frameworks impact dataset access:
- Regulatory Compliance: Organizations must align their data management practices with laws like the General Data Protection Regulation (GDPR) to protect user privacy. Violation of these regulations can result in hefty fines and loss of public trust.
- Data Sharing Constraints: Privacy laws may limit the type and extent of data that can be shared publicly. As such, repositories need to carefully navigate these waters, balancing transparency with protection.
- Informed Consent: Specifically within the domains of research, ensuring that data subjects are aware and consenting to how their data is used is essential. This ethical consideration might restrict access to certain datasets or necessitate anonymization.
In summary, future trends in dataset accessibility not only highlight the importance of innovative technologies but also emphasize the pivotal role of data privacy regulations. Both aspects dictate the ecosystem of data availability moving forward.