Pooled Analysis of Clinical Trials: A Comprehensive Examination
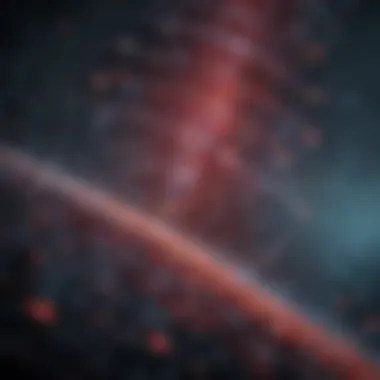
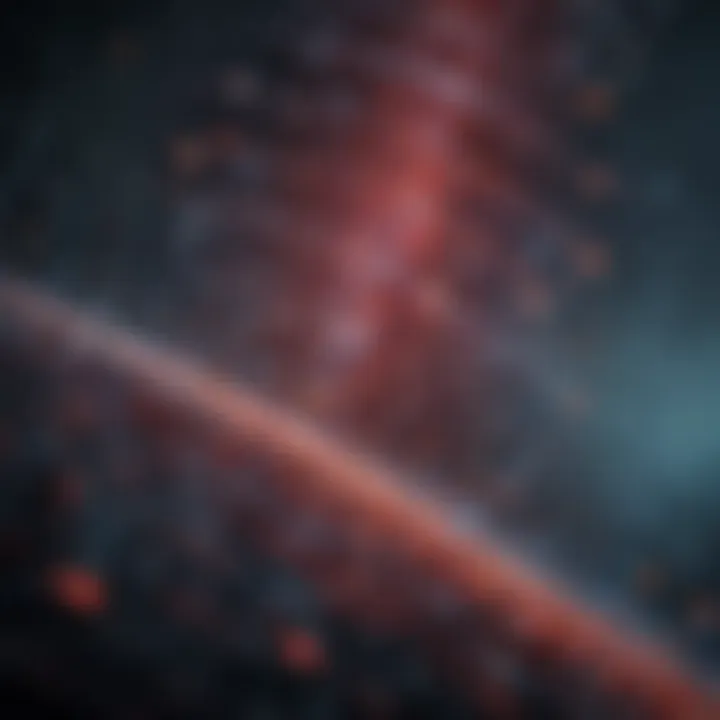
Intro
Pooled analysis stands as a significant methodology within clinical research, offering the ability to merge data from separate trials to arrive at more comprehensive insights. This approach helps in understanding patterns that individual studies may not reveal, ultimately contributing to better clinical decision-making. By analyzing a larger dataset, researchers can enhance the precision of their findings, leading to improved patient outcomes.
In this exploration, the examination of pooled analysis will encompass its definition, significance, methodologies, challenges, and advancements. Specific focus will be given to the statistical techniques involved and the implications such techniques have on real-world healthcare scenarios. Awareness of this methodology is crucial for students, researchers, educators, and healthcare professionals alike.
Research Overview
Summary of Key Findings
The research surrounding pooled analysis reveals several key insights:
- Increased Statistical Power: By consolidating data from multiple trials, the statistical power of the analysis is often significantly enhanced, allowing for the detection of treatment effects that might be missed in smaller studies.
- Generalizability of Results: Findings derived from pooled data tend to have greater external validity, meaning researchers can apply them more confidently to broader populations.
- Identification of Biases: This method allows for a critical examination of biases that may exist in individual studies, providing a more nuanced understanding.
Importance of the Research in Its Respective Field
Pooled analysis is particularly vital in fields where clinical evidence is paramount. Its ability to synthesize data not only bridges gaps in research but also supports healthcare practitioners in making informed decisions. By integrating findings from diverse studies, this method can illuminate commonalities and discrepancies, which helps to delineate best practices in treatment protocols.
Methodology
Description of the Experimental or Analytical Methods Used
The process of conducting a pooled analysis often involves:
- Data Extraction: Systematic retrieval of data from multiple studies, ensuring all relevant variables are consistently captured.
- Statistical Techniques: Utilizing meta-analysis or other statistical models, researchers can calculate overall effects while adjusting for potential confounders.
Sampling Criteria and Data Collection Techniques
When undertaking a pooled analysis, the following sampling criteria are typically observed:
- Studies included must have similar participant demographics to maintain homogeneity.
- The interventions assessed need to be comparable, allowing for meaningful conclusions.
Data collection often involves referencing databases, such as PubMed or clinical trial registries, to identify eligible studies. Assembling such data requires meticulous attention to methodological rigor to minimize any biases that might skew results.
"Pooled analysis stands as a bridge connecting individual studies, enhancing the understanding of clinical phenomena."
Through careful execution of pooled analysis, researchers are better equipped to derive insights that can influence both future research directions and immediate clinical applications.
Prelude to Pooled Analysis
Pooled analysis occupies a crucial niche within the field of clinical trials, allowing the integration of data from multiple studies to enhance the overall validity and reliability of research findings. By combining datasets, researchers can achieve enhanced statistical power, addressing the often complex nature of clinical questions. The significance of this analytical approach cannot be overstated, as it directly translates to improved decision-making in healthcare settings, ultimately affecting patient outcomes. This section seeks to underscore the essential elements of pooled analysis, elaborating on its benefits and considerations.
Definition and Scope
Pooled analysis refers to the statistical method where data from several independent clinical trials are combined and analyzed as a single dataset. This technique is critical in determining the efficacy and safety of interventions across diverse populations and settings. Its scope encompasses a wide range of therapeutic areas, from oncology to cardiology, reflecting the versatility of this methodology across various clinical domains. The ability to harness information from numerous trials enables researchers to conduct robust tests of hypotheses and generate more generalizable results.
Historical Context
The evolution of pooled analysis can be traced back to the increasing need for rigorous evidence in medical research. Historical precedents show that early medical trials were often limited by their small sample sizes, constraining the reliability of outcomes. As the field advanced, the concept of data pooling emerged as a logical solution to such limitations. Landmark studies, particularly during the late 20th century, highlighted the potential of pooled analysis to synthesize knowledge across different trials. This historical backdrop establishes a foundation for understanding the modern applications and methodologies of pooled analysis in the clinical research landscape.
The Rationale for Pooled Analysis
Pooled analysis serves as a fundamental methodological approach in the realm of clinical trials. It integrates data from different studies, aiming to bolster the overall validity of research conclusions. The rationale behind pooled analysis is multi-faceted, focusing on enhancing statistical power and addressing the complexities inherent in clinical queries.
Enhancing Statistical Power
Statistical power refers to the probability that a study will detect an effect if it exists. One significant advantage of pooled analysis is its ability to significantly increase statistical power. When individual studies have small sample sizes, it becomes challenging to draw robust conclusions. By synthesizing data from multiple trials, researchers can increase the overall number of participants, which leads to more reliable estimates of treatment effects.
For instance, consider two separate clinical trials evaluating a new medication's efficacy. Each trial may not have sufficient power to demonstrate statistically significant results due to limited participant numbers. However, when data from both trials are pooled, the sample size grows, enhancing the analytical robustness. This comprehensive approach reduces the likelihood of type II errors, where a true effect is overlooked due to unpowered studies.
This aspect of pooled analysis also assists in subgroup analysis, enabling researchers to evaluate treatment effects in different populations or settings. This granularity can provide insights that would remain hidden in individual studies.
Addressing Clinical Question Complexity
In today's clinical research landscape, questions often involve complex variables. Individual studies may assess only a few outcomes or risk factors, but pooled analysis allows for a broader examination of various interconnected elements. This method not only answers whether a treatment is effective but also how it performs under different conditions and populations.

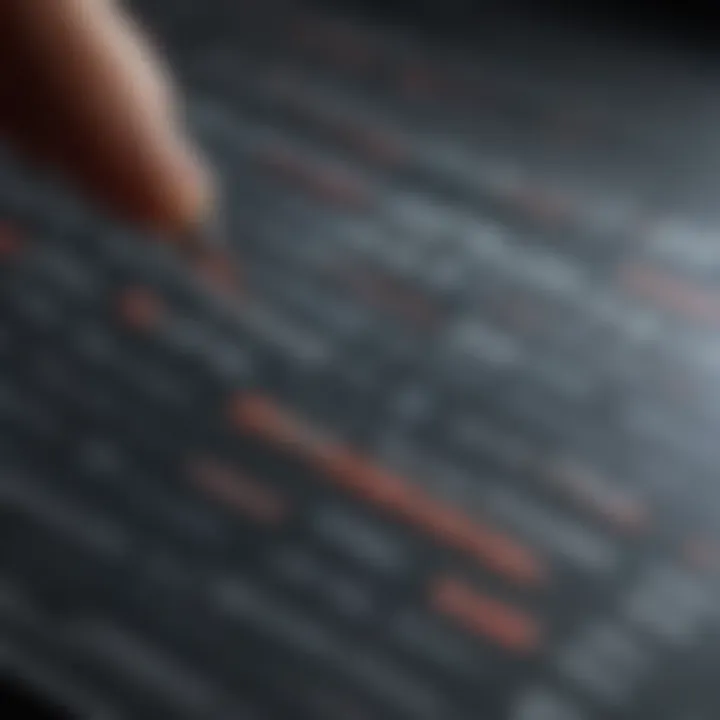
Moreover, pooled analysis enhances the exploration of interactions between various treatment effects and participant characteristics. For example, researchers might want to understand how age or pre-existing conditions influence treatment outcomes. By combining data, more nuanced conclusions can be drawn that reflect real-world variability.
Pooled analysis thus not only amplifies statistical power but also provides a richer, more complex understanding of clinical questions, bridging gaps left by isolated studies.
Methodological Framework
The methodological framework serves as the backbone of pooled analysis in clinical trials. It encompasses the structured procedures and techniques critical for integrating diverse datasets. Establishing a robust framework is essential to ensure the validity and reliability of findings. This framework consists of several vital components, including the methods of data collection, the statistical techniques employed, and the distinctions between meta-analysis and pooled analysis. Understanding each of these aspects can significantly influence the outcome and applicability of the research.
Data Collection Techniques
Data collection techniques are fundamental in any research undertaking. In pooled analysis, these techniques must be meticulously chosen to ensure that the data is compatible across studies. Effective data collection can enhance the credibility of pooled results. Common methods include:
- Standardized questionnaires: To ensure consistency across studies, using the same questionnaires can help in obtaining uniform data.
- Electronic health records (EHRs): Utilizing EHRs can facilitate the collection of data from various clinical settings, enhancing diversity.
- Clinical trial registries: These can provide valuable data on ongoing and completed trials, contributing to the comprehensiveness of the analysis.
Each method has its pros and cons; thus, selecting the appropriate technique is crucial depending on the study objectives and resources available.
Statistical Methods for Pooled Analysis
The choice of statistical methods directly affects the outcomes of the analysis. Effective statistical techniques help to address and control for variability and bias. Key statistical methods for pooled analysis include:
- Fixed Effects Model: This approach assumes that all studies share a common effect size. It is useful when variations in populations are minimal.
- Random Effects Model: This model accounts for variability among studies, acknowledging that different studies may have different effect sizes. This approach is preferable when there is substantial heterogeneity.
- Standardized Mean Differences (SMD): This method is used to compare means across studies with different measurement scales.
Choosing the right statistical method aligns with the research question and the characteristics of the data being analyzed. The impact of selecting an inappropriate model can misrepresent the actual effects.
Meta-Analysis vs. Pooled Analysis
While often used interchangeably, meta-analysis and pooled analysis have distinct characteristics. It is essential to clarify the differences to grasp their specific applications fully.
- Meta-Analysis: This is a statistical technique that combines results from separate but similar studies. The aim is to produce a summary estimate of the effect of an intervention.
- Pooled Analysis: In contrast, this method involves aggregating individual participant data across studies. Such an approach can offer deeper insights, as it maximizes the available data and permits more sophisticated analyses.
In summary, understanding these differences allows researchers to select the most appropriate method for their specific objectives, enhancing the overall quality of clinical evidence.
Types of Pooled Analysis
Understanding the various types of pooled analysis is crucial for researchers and practitioners in clinical trials. This section elucidates the primary models used in pooled analysis, specifically the fixed effects model and the random effects model. Each model serves distinct purposes, addressing different research scenarios and assumptions about study data.
Fixed Effects Model
The fixed effects model is a statistical approach that assumes that the effects being measured across studies are all actually the same, implying that differences in study outcomes are purely due to sampling variability. This model is most suitable when the studies analyzed are sufficiently homogeneous regarding factors such as population characteristics and treatment protocols.
In practical terms, the fixed effects model provides several benefits, such as:
- Precision in Estimation: By pooling data under the assumption of a common effect, researchers can generate more reliable summaries of the intervention's impact on outcomes.
- Increased Statistical Power: Aggregating data enhances the overall sample size, leading to more robust statistical analysis and increased power to detect true effects.
However, the fixed effects model has limitations. It cannot account for variations in treatment effects due to differing populations or conditions across studies. Thus, this model operates best when the included studies are quite similar.
Random Effects Model
In contrast, the random effects model acknowledges variability between studies, allowing for different treatment effects across diverse populations or methodologies. This approach is beneficial when heterogeneity exists among the trials being analyzed, whether due to differences in participant demographics, treatment variation, or study design.
The random effects model brings several advantages, including:
- Generalizability of Results: It enables researchers to draw conclusions that can apply to broader populations, taking into account the variability present among studies.
- Flexibility in Analyses: By estimating variance between studies, this model can better handle the complexities inherent in clinical practice, leading to more nuanced interpretations of results.
Despite its strengths, the random effects model also presents challenges, particularly regarding the potential for increased variance estimates, which can lead to wider confidence intervals and, in some cases, underpowered studies.
In summary, both the fixed effects model and the random effects model play pivotal roles in pooled analysis. Each model has its context where it excels, and understanding their differences is essential for selecting the appropriate approach to address specific clinical questions. Researchers must carefully consider the characteristics of the studies being pooled, the research question at hand, and the inherent assumptions of each model to ensure accurate and meaningful results.
"Choosing the right model for pooled analysis is not merely a statistical choice but a critical decision that can influence clinical outcomes and interpretations."
Ultimately, the effective use of pooled analysis can significantly impact the quality of evidence generated in clinical trials, leading to informed decision-making and improved healthcare outcomes.
Selection Criteria for Clinical Trials
The selection criteria for clinical trials are pivotal in ensuring the integrity and applicability of pooled analysis results. Establishing specific criteria helps in pinpointing which studies will be included or excluded from the pooled analysis. This process is crucial as it ultimately affects the validity of the conclusions drawn from the combined data.
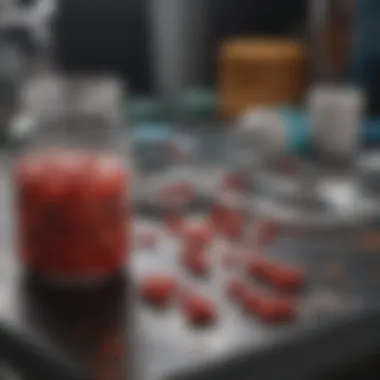
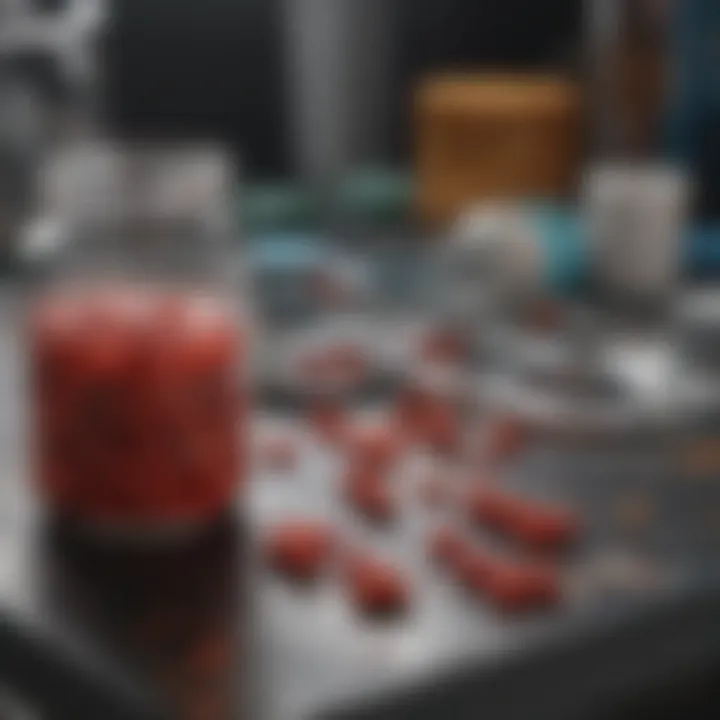
Inclusion and Exclusion Criteria
Inclusion and exclusion criteria serve as the backbone for selecting appropriate trials for analysis. Inclusion criteria outline the specific characteristics that studies must possess to be part of the analysis. These might include factors such as:
- Participant age ranges
- Specific medical conditions or diagnoses
- Treatment types or interventions
Each criterion ensures that the studies selected share a commonality that makes their data comparable.
Conversely, exclusion criteria outline the factors that disqualify certain trials from inclusion, which can include:
- Studies with insufficient sample sizes
- Trials without clear methodological standards
- Studies that report outcomes irrelevant to the research question
Establishing these criteria helps to maintain the quality of the pooled analysis. It simplifies the challenge of ensuring that all included studies are methodologically sound, making the resulting analysis more reliable and applicable to broader clinical questions.
Quality Assessment of Trials
Assessing the quality of the selected clinical trials is equally significant. Quality assessment evaluates the methodological rigor and overall integrity of the studies being considered. Several tools and guidelines exist for the quality assessment process, varying in detail but sharing a common objective: to establish confidence in the data being pooled.
When evaluating quality, researchers consider aspects such as:
- The randomization process used in trials
- The blinding of participants and researchers
- Completeness of data reporting
- The transparency of potential conflicts of interest
The incorporation of quality assessments results in a more robust pooled analysis. It can highlight strengths and limitations across the trials, ensuring that the final results are representative of genuine effects rather than artifacts of poor-quality studies. This rigor is essential for drawing informed conclusions that influence clinical practices and guidelines.
In summary, well-defined selection criteria and thorough quality assessments are foundational for conducting a credible pooled analysis. Their careful execution underpins the reliability and applicability of conclusions drawn, directly impacting clinical decision-making and the advancement of medical research.
Applications of Pooled Analysis
Pooled analysis is a pivotal methodological approach in clinical research that combines data from multiple studies. Its applications are broad and significant. The importance of such analysis lies in its ability to enhance the interpretability of medical data, provide more robust conclusions, and support informed decision-making. The advantages span various sectors in healthcare, most notably in regulatory affairs and the development of clinical guidelines.
Regulatory Perspectives
Regulatory authorities, such as the U.S. Food and Drug Administration (FDA) and the European Medicines Agency (EMA), often rely on pooled analyses to evaluate the safety and efficacy of new drugs and therapies. When reviewing clinical trial data, these organizations look for comprehensive evidence that spans different populations and study designs. Pooled analysis offers a way to systematically aggregate this evidence.
The regulatory significance of pooled analysis includes:
- Stronger Evidence Base: Aggregating data from several trials increases sample size and statistical power. This robustness is crucial for regulatory approval processes.
- Risk Assessment: Pooled analyses can identify rare adverse events by examining a larger cohort than any single study could provide.
- Guideline Formation: Regulatory bodies use findings from pooled analyses to inform their clinical guidelines, ensuring that recommendations are based on the most comprehensive evidence available.
However, ensuring methodological rigor is essential when conducting a pooled analysis for regulatory purposes. Inaccuracies can lead to misleading conclusions, affecting the approval process or clinical recommendations.
Clinical Guidelines Development
Clinical guidelines play a fundamental role in practice standards for healthcare providers. Pooled analysis feeds into the creation of these guidelines by providing a consolidated view of the best available evidence. The nuanced approach can address various conditions and treatment methods, offering clarity on the most effective strategies.
Key benefits of pooled analysis in clinical guidelines development include:
- Consistency: By synthesizing data from different studies, pooled analysis helps create a more uniform set of recommendations that minimizes variability.
- Best Practices: It identifies and promotes best practices across diverse clinical settings, which is vital for patient-centered care.
- Dynamic Adaptation: Clinical guidelines informed by pooled analysis allow for adjustments as new data emerges. This adaptability supports ongoing improvement in clinical outcomes.
In summary, the applications of pooled analysis are extensive and vital for both regulatory oversight and the formulation of clinical guidelines. By offering a more complete view of the evidence, it enhances the standard of care provided to patients and aids in the advancement of medical knowledge.
Challenges and Limitations
In conducting pooled analysis within clinical trials, several challenges and limitations emerge that can greatly influence the integrity and applicability of the results. Recognizing these issues is important because they can significantly affect both the methodological quality of the analysis and the trustworthiness of the conclusions drawn. This section delves into two specific challenges: data heterogeneity and publication bias, both of which require careful consideration in the research process.
Data Heterogeneity
Data heterogeneity refers to the variability in study designs, populations, interventions, and outcome measurements across the trials included in a pooled analysis. Such differences can lead to complications in synthesizing results and drawing reliable conclusions. It is crucial to assess the extent of this heterogeneity before combining data, as high levels can obscure true patterns and effects.
- Impact on Analysis: When studies included in the analysis vary significantly in design or characteristics, it can cloud the reliability of the pooled results. For instance, combining data from trials that utilize different treatment protocols may yield results that are not applicable to any specific population.
- Statistical Tests: Several statistical methods, such as the Iยฒ statistic, can gauge the level of heterogeneity. These tests help researchers determine whether the variability among studies is beyond what could be expected by chance alone. If significant heterogeneity exists, it may be prudent to explore subgroup analyses or meta-regression techniques to identify sources of variability.
As one expert states,
"Understanding heterogeneity is key to deriving meaningful interpretations from pooled analyses.โ
This acknowledgment is vital in guiding researchers in the appropriate techniques to apply, ensuring that they draw accurate conclusions aimed at improving healthcare outcomes.
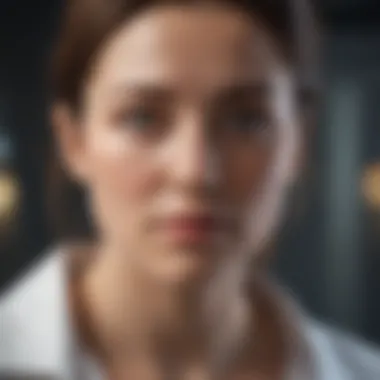
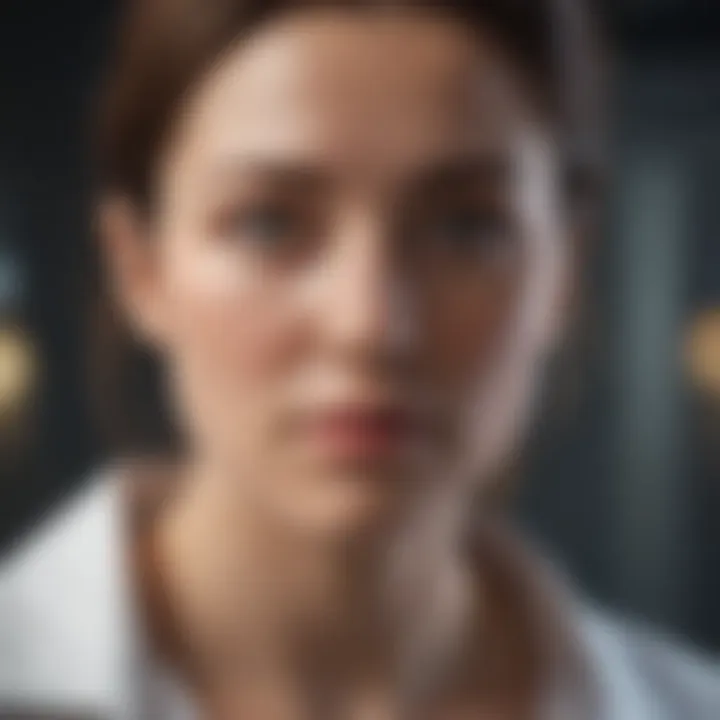
Publication Bias and Interpretation
Publication bias occurs when the results of studies influence the likelihood of publication. Typically, studies with positive or significant outcomes are more likely to be published than those with negative results. This bias can distort the overall findings of a pooled analysis and lead to an overly optimistic representation of treatment effects.
- Consequences of Bias: If unpublished studies, particularly those demonstrating lack of efficacy, are excluded, the pooled results can present a skewed understanding of an interventionโs effectiveness. This bias can mislead clinical practitioners and affect decision-making.
- Attempts to Mitigate: Techniques such as funnel plots and trim-and-fill methods can help assess publication bias. By visualizing the distribution of study outcomes, researchers can identify potential biases and adjust their analyses accordingly.
- Transparency in Reporting: It is crucial for researchers to emphasize transparency when conducting pooled analyses. Reporting biases can shape interpretation and, ultimately, influence clinical guidelines and healthcare decisions.
Best Practices for Conducting Pooled Analysis
Pooled analysis is a vital component in the realm of clinical trials, providing a framework that can enhance the validity and applicability of research findings. Implementing best practices is crucial to ensure that the results of pooled analyses are robust, reliable, and ultimately useful in informing clinical decisions.
Methodological Rigor
Methodological rigor is the cornerstone of any successful pooled analysis. It encompasses the careful design, execution, and evaluation of studies included in the analysis. Prior to pooling data, it is essential to establish clear and consistent inclusion and exclusion criteria. This ensures that all selected studies are comparable in terms of population, intervention, and outcomes.
The use of validated tools for quality assessment is also fundamental. Tools such as the Cochrane Risk of Bias Tool or the Newcastle-Ottawa Scale can provide systematic ways to assess the quality of studies. By ensuring high-quality studies are included, researchers can enhance the validity of the pooled results.
Another key aspect is the appropriateness of the statistical methods employed. The choice between fixed effects and random effects models should align with the level of heterogeneity among the studies. A proper understanding of the data and conditions is necessary to optimize statistical power and minimize type I and II errors. Adjustments for potential confounders or bias must also be considered in the analysis to bolster the rigor of conclusions drawn.
Transparent Reporting Standards
Transparency in reporting is integral to the credibility of pooled analyses. Clear and concise reporting allows others in the field to critically evaluate the methodology used and the implications of the findings. Adopting guidelines like the PRISMA statement can guide researchers in structuring their pooled analyses to ensure comprehensive reporting.
Key elements of transparent reporting include:
- Study selection process: Clearly document the criteria used for selecting studies and any reasons for exclusion.
- Data extraction methods: Describe how data was gathered and the rationale behind the chosen methods.
- Statistical analysis: Specify the statistical techniques used and justify their appropriateness for the analysis at hand.
- Availability of data: Where possible, share datasets and analysis scripts in accessible repositories to promote reproducibility.
Transparency fosters trust and accountability in research, establishing a framework for others to rely on the studyโs findings.
Recent Advancements in Pooled Analysis
The field of pooled analysis is evolving rapidly. As researchers seek more precise and reliable insights from clinical trials, advancements in various areas are becoming essential. This section explores the recent developments that enhance the methodologies used in pooled analysis, thus impacting its overall effectiveness in clinical research.
Technological Integrations
Technological advancements play a critical role in facilitating pooled analysis. Integrating sophisticated software solutions can greatly improve data management and analysis efficiency. Tools such as R and Python libraries offer powerful capabilities for statistical analysis and data visualization. They enable researchers to manipulate large datasets from multiple trials seamlessly.
Moreover, cloud computing has transformed how researchers share and collaborate on data. With platforms developed for data storage and analysis, teams across different locations can access the same datasets in real time. This improves the transparency and reproducibility of results, which are fundamental aspects of quality research.
Another significant technological advancement is the application of machine learning algorithms. These algorithms can detect patterns in large datasets that might not be readily apparent through traditional statistical methods. As techniques such as predictive analytics become more commonplace, they provide a means to refine the selection criteria and stratification methods in pooled analyses. This integration leads to more accurate and tailored clinical insights.
Novel Statistical Approaches
Recent innovations in statistical methodologies are also contributing positively to pooled analysis. One promising development is the refinement of Bayesian methods. Unlike traditional frequentist approaches, Bayesian techniques offer more flexibility in incorporating prior information and beliefs into the analysis. This can be particularly useful in scenarios where data might be sparse or heterogeneous across studies.
Another important advancement is the improvement in meta-regression techniques. These techniques allow researchers to explore the relationships between study characteristics and outcomes. By identifying sources of heterogeneity in pooled analyses, researchers can provide a more nuanced understanding of how various factors influence clinical outcomes.
Additionally, the use of sensitivity analysis has gained traction as a mechanism for assessing the robustness of pooled findings. By systematically varying the parameters and assumptions, researchers can determine how vulnerable their conclusions are to changes in the data. This approach is vital for increasing confidence in the robustness of pooled analysis results, particularly in informing clinical decision-making.
In summary, the advancements in both technology and statistical methods present a significant opportunity to enhance the quality and reliability of pooled analyses. As these methodologies continue to develop and improve, they hold great promise for refining the insights that emerge from clinical trials.
The End and Future Directions
Pooled analysis serves as a fundamental component in the evolution of clinical research. Its ability to combine data from different studies enhances the statistical power, providing more reliable conclusions that can influence healthcare decisions. This section provides a summary of its current implications and outlines future directions that could benefit from this analytical strategy.
Implications for Clinical Research
The implications of pooled analysis for clinical research are profound. It enables researchers to draw conclusions from a more significant amount of data, which can lead to better understanding of treatment effects and interaction among variables. For researchers, pooled analysis presents a robust avenue for answering complex clinical questions. The analytical method also facilitates the examination of diverse populations, improving the generalizability of research findings.
Moreover, regulatory bodies are increasingly recognizing the importance of pooled analyses in decision-making processes. Trials that utilize this methodology often lead to stronger evidence for drug approvals and treatment recommendations. By synthesizing results from multiple studies, clinicians can make informed choices, ultimately enhancing the quality of patient care.
The Future Landscape of Pooled Analysis
As the field of clinical research progresses, the future landscape of pooled analysis holds promise. Advancements in technology, particularly in data-sharing platforms and analytical software, will likely streamline the process of conducting pooled analyses. Innovations in statistical methods will also improve the accuracy of results.
In addition, the industry may see a push for more transparent collaborations between researchers to share trial data. This openness can help mitigate the issues of data heterogeneity and publication bias, which currently challenge pooled analyses. Such collaborative efforts would elevate the integrity of findings and foster an environment for more rigorous testing of hypotheses.
Furthermore, as patient-centered approaches gain more traction, pooled analysis could lead to insights that are more aligned with patient preferences and outcomes. By embracing a holistic view of patient data, researchers can refine their methodologies, leading to breakthroughs in treatment and care strategies.
"Pooled analysis is not just a methodological approach; it is a bridge connecting fragmented clinical insights into coherent narratives that drive healthcare forward."
In summary, the ongoing evolution of pooled analysis signifies its vital role in shaping future research paradigms. With an emphasis on methodological rigor and partnership among stakeholders, we can expect to see enhanced outcomes in clinical practices, underpinned by comprehensive and systematic analysis.