The Role of Solar Data in Advancing Scientific Research
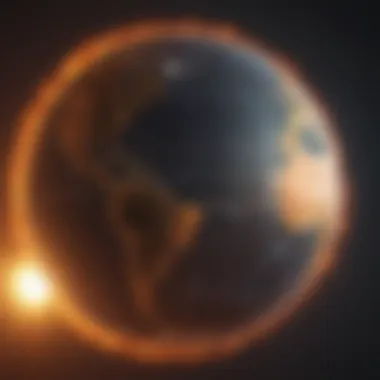
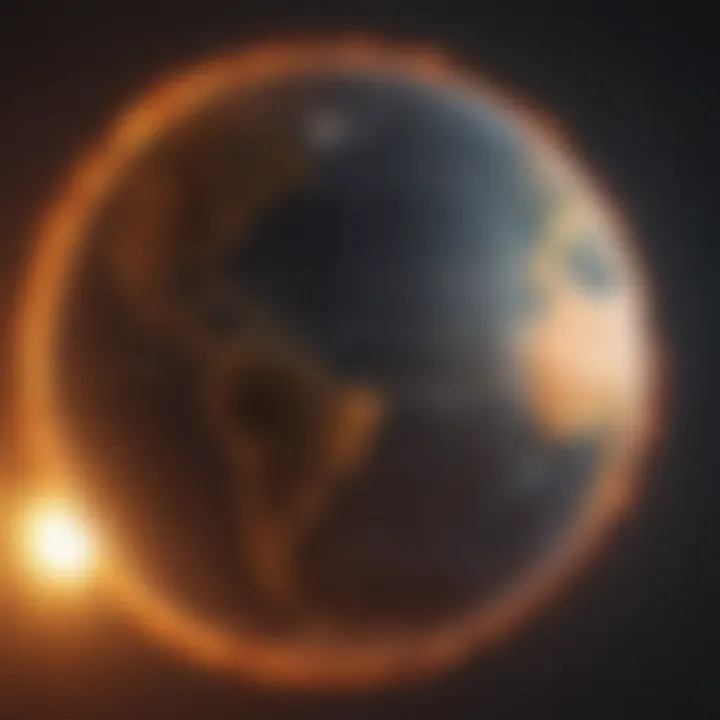
Intro
Solar data has become essential in modern research. It is not only relevant to specific fields like climate science but also impacts areas such as renewable energy development. The large quantities of solar data available today can influence methodologies, models, and policies worldwide. Understanding the significance of this data is critical for students, researchers, educators, and professionals in various disciplines.
Research Overview
Summary of Key Findings
Solar data encompasses numerous measurements related to solar radiation, solar winds, and atmospheric conditions. These metrics are collected using satellites, ground stations, and various observational tools. A key finding is that solar data helps in comprehending climatic patterns, which is crucial for predicting trends in climate change. The insights gained assist scientists in mapping out the ongoing shifts in weather systems, energy consumption habits, and even economic impacts.
Importance of the Research in Its Respective Field
The research surrounding solar data plays a transformative role in shaping several fields. In climate science, such data is critical for understanding global warming and its effects on biodiversity. In renewable energy, solar data aids in optimizing energy systems and developing more efficient solar panels. By analyzing this data, researchers can also assess the sustainability of solar energy trends.
Solar data is not simply numbers; it is the key to unlocking solutions to some of the most pressing global issues today.
Methodology
Description of the Experimental or Analytical Methods Used
The methodologies for collecting solar data vary. Typically, remote sensing techniques and in situ measurements are employed. Remote sensing involves using satellite imagery to gather data across large geographical areas. Instruments like pyranometers measure solar radiation intensity on the ground. This combination allows for a comprehensive understanding of solar energy patterns.
Sampling Criteria and Data Collection Techniques
Data collection techniques must follow specific criteria to ensure accuracy. Samples must represent diverse locations and times to avoid bias. For instance, solar data is often collected from urban, rural, and remote areas, showcasing variability. Instruments must be calibrated regularly to maintain precision. The combination of proper sampling and advanced tools supports robust data analysis that can yield meaningful insights.
Understanding Solar Data
Solar data is essential in various fields of research today. This data serves as a foundation for understanding solar energy's role in climate systems, renewable energy advancements, and agricultural practices. The growing demand for sustainable energy solutions highlights the need for comprehensive solar data collection and analysis.
In this section, we will delve into the definition of solar data and explore its various types to better grasp its relevance in modern practices. By doing so, we can appreciate how effective utilization of solar data can influence scientific research and development in energy technologies.
Definition of Solar Data
Solar data refers to the information collected regarding the sun's characteristics and the energy it emits. This data encompasses various measurements, including solar irradiance, solar radiation, and solar energy production, along with data that captures weather conditions affecting solar technologies. Understanding solar data involves knowing how to interpret and apply these measurements effectively within different research contexts.
Types of Solar Data
Solar data can be categorized into several significant types, each contributing uniquely to research and practical applications:
- Solar irradiance: This measurement refers to the power of solar radiation received per unit area. It is crucial for evaluating potential solar energy generation. The primary characteristic of solar irradiance is its variability due to atmospheric conditions and time of day. This variability affords researchers the opportunity to analyze patterns that help improve solar power systems' efficiency. However, the fluctuating nature can pose challenges in precise calculations, making data collection and accuracy vital.
- Solar radiation: This term encompasses all types of sunlight or electromagnetic energy received from the sun. Solar radiation is essential for understanding how much energy is available for solar technologies. Its key characteristic is that it accounts for both direct sunlight and diffuse components. A unique feature is its ability to influence climate models significantly. Although solar radiation data is crucial, factors like geographical location can complicate its analysis, requiring localized studies.
- Solar energy production: This refers to the actual energy output produced by solar systems, such as photovoltaic panels. One key characteristic here is its direct correlation with solar irradiance and its role in sustainable energy resource planning. The unique feature of solar energy production data is its application in renewable energy forecasting. However, measuring this production can sometimes involve dealing with technical disruptions and data loss, making consistent monitoring necessary.
- Weather data related to solar technologies: This involves atmospheric information such as temperature, humidity, and cloud cover, influencing solar energy efficiency. Its characteristic lies in its direct impact on solar technology performance, establishing a framework for improving energy systems. The unique attribute is its ability to inform real-time operational adjustments for solar installations. Yet, integrating weather data can be complex, requiring advanced processing techniques.
Understanding these types of solar data provides insights necessary for various applications, from climate modeling to efficient solar energy deployment. By mastering these concepts, researchers and professionals can better navigate the challenges of solar data analytics and contribute to advancements in sustainable practices.
"The integration of diverse types of solar data enhances the accuracy of analyses and the effectiveness of subsequent applications in sustainability initiatives."
Collection Methods
Collection methods are a backbone of solar data research. Understanding how solar data is acquired is essential for accurate interpretation and application in diverse fields. Each method comes with its advantages and limitations, ultimately influencing how data can be utilized in real-world scenarios.
Satellite-Based Observations
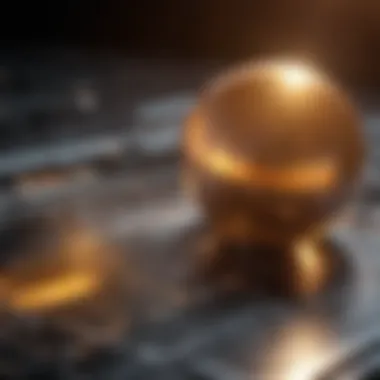
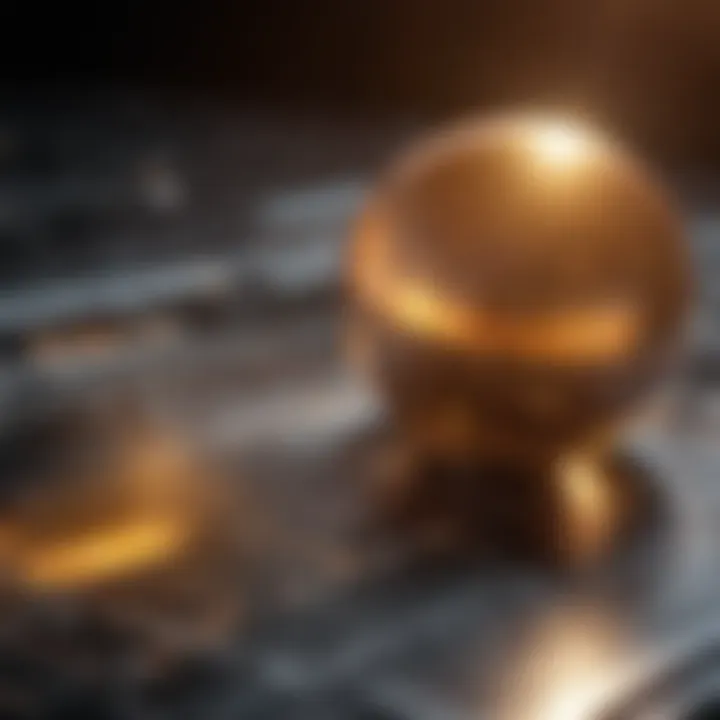
Satellite-based observations involve using satellites equipped with sensors to capture data about solar irradiance and weather patterns. This method has significant benefits. For instance, it offers a broad coverage that ground stations cannot provide. Satellites can collect data over vast areas, making it easier to monitor remote regions. Moreover, the technology allows for near-real-time data capture, aiding timely decision-making in various fields such as climate research and solar energy forecasting.
However, the accuracy of this data can sometimes be affected by atmospheric conditions, which is a critical consideration for researchers relying on this method. Careful calibrations are necessary to mitigate these issues.
Ground-Based Measurement Stations
Ground-based measurement stations provide another reliable collection method for solar data. These stations consist of physical sensors situated on land to capture immediate solar conditions. It allows for localized data which can become crucial for detailed analysis.
Types of sensors
Sensors in these stations typically include photometers and pyranometers.
- Photometers measure the intensity of light, which makes them useful for studying cloud cover and solar irradiance.
- Pyranometers measure solar radiation received on a surface, thus giving insights into energy availability.
These sensors are popular because they can offer accurate, real-time data at specific locations. Moreover, they can be calibrated for precise measurements. A unique feature of these sensors includes their adaptability; they can be used for various experiments ranging from climate studies to solar panel performance evaluations. However, one disadvantage is their limited range—data is constrained to the specific location where the sensors are installed.
Data logging techniques
Data logging techniques play a crucial role in processing the information collected by ground-based stations. They allow for the persistent recording of solar data. These techniques often incorporate advanced software capable of storing and analyzing large datasets over time.
One key characteristic of data logging is their capacity to send alerts when certain thresholds are met. This is beneficial for researchers seeking to monitor specific conditions or anomalies efficiently. The unique feature of modern data logging systems is remote access; researchers can analyze data without being physically present at the site. However, one must note that these systems require robust power supplies and can be costly to install and maintain, which may limit their adoption in some regions.
Remote Sensing Techniques
Remote sensing techniques further diversify data collection methods. This involves utilizing aerial and satellite imagery to gather solar data from a distance. By employing advanced technologies such as LiDAR and multispectral imaging, researchers can analyze solar potential over large areas without physical sensors on the ground. This method is particularly valuable in identifying suitable sites for solar installations and in evaluating land use changes over time.
All these collection methods work complementarily, providing a comprehensive understanding of solar dynamics. Gathering data through various avenues optimizes research outcomes, enhancing our knowledge of solar energy and its applications.
Data Processing and Analysis
Data processing and analysis are crucial components in the realm of solar data research. Properly analyzing solar data enables researchers to derive meaningful insights that inform decisions in climate science, energy production, and various other fields. This section discusses the importance of data processing and the analytical techniques employed to interpret solar data accurately, with a focus on normalization techniques and statistical methods.
Data Normalization Techniques
Normalization of solar data is essential for ensuring consistency and comparability across different datasets. Raw solar measurements can vary due to discrepancies in data collection methods, equipment sensitivity, and local environmental conditions. Normalization addresses these issues by adjusting data to a common standard.
There are several techniques to achieve normalization, such as:
- Min-Max Normalization: This method rescales the data to fit within a specified range, often between 0 and 1. This ensures that the values can be easily compared across different datasets.
- Z-Score Normalization: This technique transforms the data into a standard normal distribution with a mean of zero and a standard deviation of one. Z-scores are particularly useful when analyzing datasets that follow a Gaussian distribution.
- Log Transformation: Certain solar data, like energy production, may have a right-skewed distribution. Log transformation can help in stabilizing variance and making the data more interpretable.
Employing these normalization techniques enhances the reliability of solar data interpretation, providing a clearer understanding of trends and patterns. Normalized data also aids in integrating diverse datasets from various sources, facilitating comprehensive analysis.
Statistical Methods for Data Interpretation
Once solar data has been normalized, statistical methods become instrumental in interpretation. These methods allow researchers to draw conclusions and make predictions based on the analyzed data. Several statistical approaches are commonly used:
- Descriptive Statistics: This includes basic calculations such as mean, median, and standard deviations which give a quick overview of the dataset characteristics.
- Correlation Analysis: Understanding the relationships between different variables is crucial in solar studies. Correlation coefficients, such as Pearson or Spearman’s, help identify how solar irradiance might correlate with energy output or weather conditions.
- Regression Analysis: Implementing regression techniques, such as linear or nonlinear regression, facilitates the modeling of relationships between dependent and independent variables, providing insights into how various factors influence solar energy production.
- Time Series Analysis: This technique addresses data that is collected over time, helping researchers identify trends, seasonal patterns, and anomalies in solar data.
By utilizing these statistical methods, researchers can extract significant knowledge from solar data, making it applicable to real-world scenarios such as renewable energy strategies and climate modeling.
Applications of Solar Data
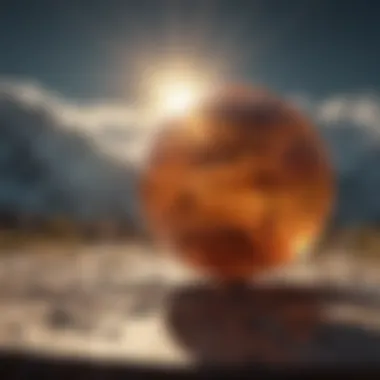
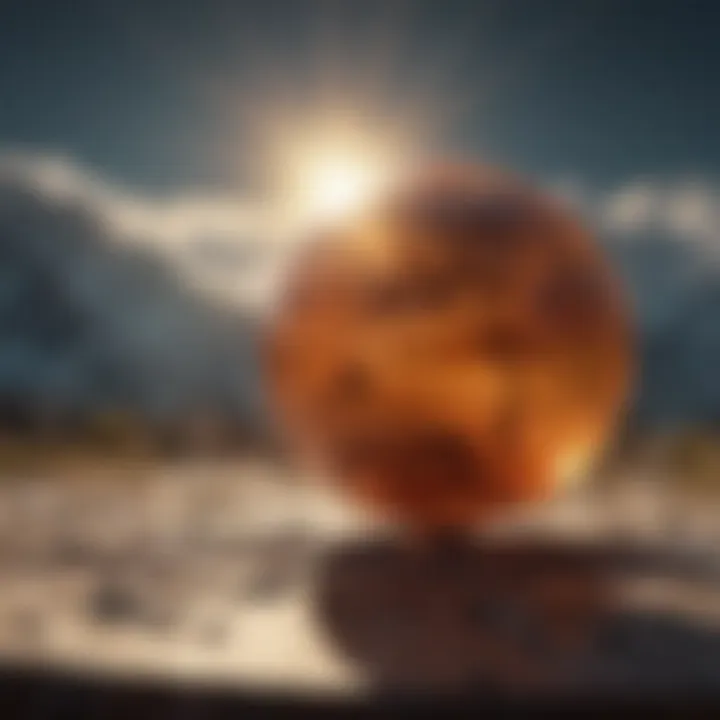
Understanding the applications of solar data is crucial in modern research. Solar data, with its diverse usages, directly impacts various fields, enhancing their capabilities and outcomes. By utilizing accurate solar information, researchers can address significant challenges in climate dynamics, renewable energy optimization, agriculture strategies, urban planning, and infrastructure development. This section delves into specific applications, emphasizing their importance and the benefits they offer.
Climate Modeling
Climate modeling serves as a vital application of solar data. It allows scientists to simulate and predict climatic conditions by integrating solar irradiance and radiation values. Solar data informs models about the energy input from the sun, essential for understanding temperature variations, weather patterns, and seasonal changes. The incorporation of precise solar measurements leads to improved accuracy in climate models, fostering better predictions. In essence, leveraging solar data provides a foundation for informed decision-making related to global warming and climate change adaptation.
Renewable Energy Development
Renewable energy development relies heavily on solar data to innovate and optimize energy extraction technologies. The potential to harness solar power is enormous, but understanding solar patterns and trends is key to maximizing efficiency. Solar data facilitates critical assessments in two main areas: solar panel efficiency analysis and grid management.
Solar panel efficiency analysis
The analysis of solar panel efficiency involves evaluating how well solar panels convert sunlight into usable energy. This aspect is significant as it contributes to optimizing solar technologies for higher output. What makes this analysis beneficial is its ability to provide empirical evidence on panel performance under various conditions. By analyzing solar data, researchers can identify which panel technologies yield the most effective results in different environments.
However, challenges exist in evaluating efficiency consistently across diverse geographic and climatic settings, which can distort performance metrics. Thus, comprehensive analysis using solar data is indispensable for ensuring energy reliability.
Grid management
Grid management is another critical component supported by solar data. Effective management of electrical grids is essential as energy sources diversify. Solar data aids in predicting energy generation patterns, helping grid operators maintain stability and reliability. Specifically, the integration of solar forecasting into grid systems allows operators to optimize load balancing, reducing the risk of blackouts. Furthermore, understanding solar input enables the incorporation of more renewable energy into existing grids. Despite its advantages, grid management requires sophisticated infrastructure and investment, limiting its implementation in some regions. Nonetheless, the role of solar data in enhancing grid management is increasingly recognized.
Agricultural Planning
Agricultural planning benefits significantly from solar data, as it informs farmers about optimal planting and harvesting times based on sunlight availability. By analyzing solar radiation data, farmers can make informed decisions about crop types and their siting, improving yield outcomes. This optimization adds a layer of resilience against shifts in climatic conditions. Solutions derived from solar data contribute to sustainable farming practices and food security.
Urban Planning and Infrastructure
Urban planning and infrastructure development also leverage solar data for informed decision-making. City planners use solar information to optimize building designs, ensuring they capture maximum sunlight. This consideration not only improves energy efficiency but also enhances the comfort of inhabitants. Moreover, incorporating solar data into urban transportation systems can minimize congestion and emissions, promoting sustainability. As cities grow, reliance on solar data will only increase, shaping how urban environments adapt to future energy demands.
In summary, the applications of solar data are vast and impactful across several domains. By understanding and leveraging this data effectively, researchers and practitioners can optimize outcomes, enhance decision-making, and foster sustainable development.
Current Challenges in Solar Data Research
The field of solar data research faces a myriad of challenges that directly affect the credibility, efficiency, and overall impact of solar studies. Understanding these challenges is crucial for researchers, policymakers, and industries that rely on solar data to inform their decisions. These challenges include issues related to data accuracy and validity, integration of various data sources, and the availability of funding and resources. Each of these elements warrants a thorough examination, as they all play significant roles in the advancement of knowledge in solar energy and climate science.
Data Accuracy and Validity
Data accuracy and validity are paramount in solar data research. Reliable data is necessary for effective modeling and analysis, which can directly influence energy policies and strategies. Inaccurate data can lead to misguided decisions, ultimately jeopardizing efforts to harness solar power efficiently.
One major aspect of data accuracy is the calibration of instruments used to collect solar measurements. If sensors are not regularly calibrated, the data they collect may be flawed or untrustworthy. Furthermore, the environmental conditions, such as temperature fluctuations and atmospheric influences, can also skew the data gathered from solar irradiance sensors. Therefore, maintaining strict quality control protocols is essential.
Validity, on the other hand, refers to whether the data truly represents what it is intended to measure. A common challenge is the bias in data sets due to regional factors that may not translate to broader trends. Researchers must acknowledge these biases and actively seek out methods to counteract them, ensuring that their findings represent the actual phenomena being studied.
Integration of Data Sources
The integration of data sources remains a challenge in solar research. Modern solar studies often require data from multiple sources to achieve a comprehensive understanding of solar phenomena. This can include satellite data, ground measurements, and meteorological data. However, combining data from different platforms presents various obstacles, such as differences in data formats, collection methods, and temporal resolution.
Standardization is a critical consideration when attempting to merge diverse data sets. Without clear guidelines for data integration, inconsistencies can arise, leading to flawed analyses. Additionally, the development of software tools that facilitate the integration process is still an evolving area. Efforts must continue to improve these technologies to allow for more seamless data aggregation and analysis, paving the way for more robust results in solar research.
Funding and Resources
Lastly, the issue of funding and resources cannot be overlooked. Progress in solar data research heavily depends on the availability of financial support and resources. Limited funding can restrict the scope of research, leading to fewer innovative projects and less comprehensive studies.
Several funding sources exist, including government grants, private sector investments, and academic partnerships. Each source has its own set of priorities and restrictions, which can sometimes hinder research efforts. Access to high-quality data collection tools, processing capabilities, and research personnel are all contingent upon adequate funding. Thus, advocating for increased investment in solar data research is critical in tackling current challenges and ensuring the field's growth.
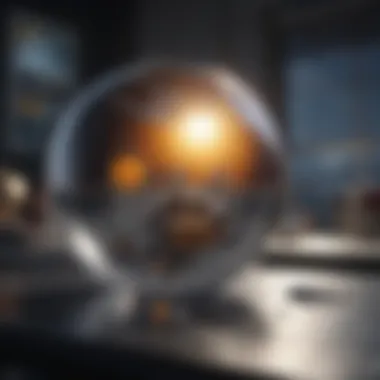
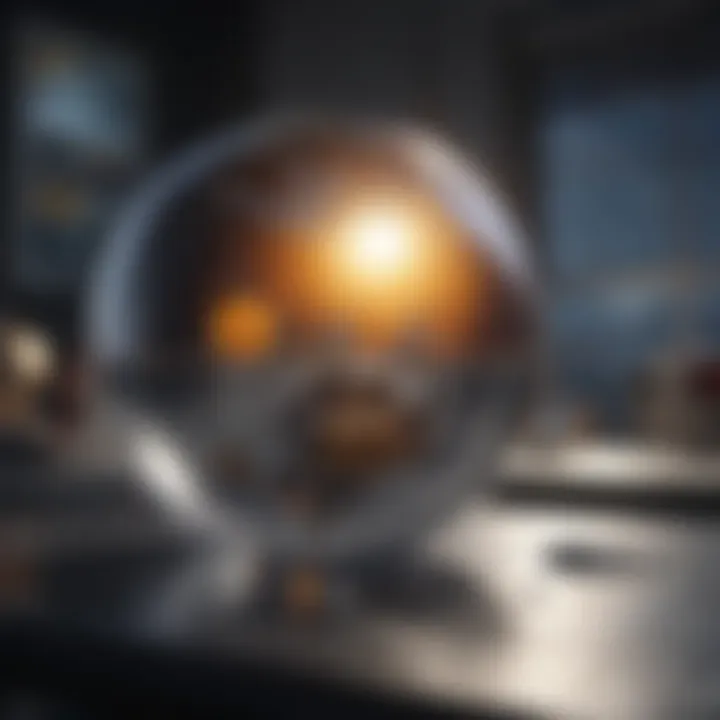
"Addressing these challenges in solar data research is essential for advancing our understanding of solar phenomena, directly influencing policy-making and technological progress in renewable energy."
Ultimately, confronting the challenges of data accuracy and validity, integration of data sources, and funding and resources is necessary for advancing solar data research. These aspects, if addressed effectively, will contribute significantly to strengthening the foundation upon which modern solar studies rest.
Future Directions in Solar Data Research
The field of solar data research is continuously evolving, driven by advancements in technology and changes in societal needs. Understanding the future directions in this domain is essential, not only for researchers but also for policymakers and industry stakeholders. Solar data is vital for informing our approaches to climate change, energy sustainability, and technological innovation. New methodologies and tools are emerging that promise to enhance data collection and analysis, making the research more impactful than ever.
Innovative Data Collection Technologies
The future of solar data collection is closely tied to innovations in technology. Current methods, while effective, can be significantly enhanced through the integration of new tools. For instance, drones equipped with solar sensors can cover vast and remote areas, capturing high-resolution data on solar irradiance and atmospheric conditions. These technologies not only increase the speed of data collection but also improve accuracy, especially in previously hard-to-reach locations.
In addition, IoT devices can gather real-time data on solar energy production at residential and commercial facilities. This information can provide insights into local solar potential and contribute to grid management strategies. Furthermore, the introduction of smart satellite technology will enable more precise tracking of solar events and radiation patterns on a global scale. The combination of these innovative technologies will lead to a wealth of data that can generate new understanding and solutions in the renewable energy sector.
Machine Learning and AI Applications
The use of machine learning and artificial intelligence in solar data analysis is a burgeoning area that holds immense potential. These technologies can sift through large volumes of solar data more effectively than traditional methods, identifying patterns and trends that might not be immediately evident to human analysts. Machine learning algorithms can optimize solar panel placement by analyzing factors such as geographic data, local atmospheric conditions, and historical solar energy output.
Moreover, AI applications can assist in predictive modeling, allowing researchers and energy developers to forecast solar energy production under varying conditions. This can lead to more efficient energy management and planning, ensuring that solar power is harnessed effectively.
Impact on Policy and Decision Making
The impact of solar data on policy and decision making is significant. Governments and organizations increasingly rely on this data to steer energy-related policies. Solar data encompasses a variety of information such as solar irradiance, energy production metrics, and weather data. These factors help inform and shape energy policies that address both current and future energy needs.
Solar data offers critical insights that support the design and implementation of effective energy policies. By understanding the patterns of solar energy production and consumption, policymakers can identify potential areas for growth and investment. Additionally, they can pinpoint regions where solar energy can significantly reduce dependency on non-renewable resources, thus fostering a transition to cleaner energy sources.
Informing Energy Policies
Accurate solar data directly informs energy policies, providing the foundational metrics necessary for developing sustainable strategies. For instance, decisions regarding the expansion of solar farms or incentives for solar panel installation derive from comprehensive data analysis. Governments analyze solar radiation levels and historical weather patterns to assess the viability of solar projects in various locations.
Furthermore, energy policies based on solid solar data promote the adoption of renewable energy technologies. By understanding local energy consumption patterns, policymakers can tailor incentive programs to stimulate solar installation. This can lead to reduced energy costs for consumers and decreased carbon footprints on a community level.
Mitigating Climate Change Effects
Solar data plays a crucial role in addressing climate change. Policymakers utilize solar data to predict future energy usage trends and related environmental impacts. This data helps frame climate policies, aiming to mitigate the adverse effects of climate change. By emphasizing solar energy, governments can reduce greenhouse gas emissions.
Moreover, authorities can use solar data to implement measures that enhance resilience against climate-related disturbances. For example, during extreme weather events, understanding solar data allows for better management of energy resources. The effective deployment of solar technologies can provide backup energy solutions when traditional grids fail.
"Proper analysis and understanding of solar data can shape more effective climate policies, leading to better sustainability practices."
In summary, solar data holds tremendous potential in informing energy policies and mitigating the effects of climate change. As reliance on this data grows, its implications for policy-making will expand, ultimately contributing to a more sustainable energy future.
Closure
The conclusion of this article encapsulates the significant role solar data plays in contemporary research. The insights gathered from solar data inform various disciplines, particularly climate science and renewable energy. By emphasizing analytical methods, researchers can draw meaningful conclusions, leading to advancements that can alter the trajectory of environmental policies and energy strategies.
One of the primary benefits of understanding and utilizing solar data lies in its application in policy-making. Accurate data underpins effective energy policies which are crucial in addressing climate change impacts. Decisions grounded in empirical data can promote sustainable practices and drive innovations in technology.
Furthermore, the evolving nature of data collection techniques and analytical methods enhances the depth of understanding of solar dynamics. As future technologies emerge, the integration of advanced methodologies such as machine learning and AI will refine how solar data is processed and utilized. This evolution in data handling ultimately creates more robust models for energy production and climate modeling.
It is also essential to consider the challenges surrounding solar data, such as data validity and integration processes. Addressing these challenges is necessary for the continuous evolution of solar data research and its applications. Efforts to standardize and improve data accuracy will lead to enhanced research outcomes and greater trust in solar datasets.
Final Thoughts on Solar Data
Solar data holds intrinsic value in shaping our understanding of energy systems and climate dynamics. Researchers and professionals are charged with the responsibility of not only collecting this data but also analyzing it thoughtfully. As we navigate a world increasingly focused on renewable energy and sustainability, leveraging solar data will be paramount.
By focusing on collaboration and interdisciplinary efforts, the future of solar data research appears promising. Advances in data analytics will reveal new insights and foster innovations that can address urgent global challenges. Thus, the pursuit of improved solar data research is not just a theoretical endeavor; it is crucial for our collective future.